How to measure the quality of macroeconomic models
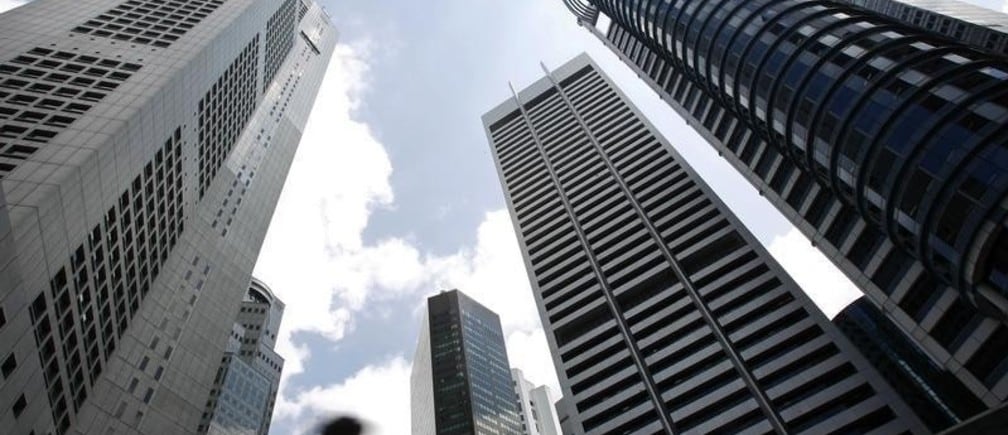
Macroeconomic models have a poor reputation for empirical accuracy. Macroeconomics has been criticised widely (e.g. Hansen and Heckman 1996) as subjective and untestable on the poor data available; thus macroeconomists build highly abstract dynamic stochastic general equilibrium (DSGE) models based on their own beliefs, either imposing calibrated parameters or estimating them by Bayesian methods that impose these beliefs on the data. Furthermore, the widely used practice of comparing stylised facts with model simulations is not based on proper statistical distributions.
Not surprisingly, therefore, the search has been on for effective ways to test macroeconomic models on the data. One such method is ‘out-of-sample forecasting’ (OSF) tests. One economist who has recently devoted much effort to testing DSGE models in this way is Refet Gürkaynak with several coauthors (Edge and Gürkaynak, 2010, Gürkaynak et al. 2013). His method has been to set up various unrestricted time series models and see whether the DSGE model could forecast better or worse than these; the idea being that forecast efficiency is improved by imposing well-specified restrictions on the data. Thus an outperforming DSGE model must embody some good theory providing such restrictions. In their work, Gürkaynak and his coauthors have found mixed results. Some models perform better than some time series models in some episodes, and some worse. All episodes are limited in length, and so are small samples.
New evidence
In recent work, Yongdeng Xu, Peng Zhou, and I ask: What do these tests tell us about the quality of DSGE models (Minford[RB1] et al. 2014)? Two qualities of models are relevant for users such as policymakers:
How good is the model’s specification (relevant for judging the effects of policy changes)?
How well does the model forecast (relevant to the widespread need for forecasts)?
We answer this question by a Monte Carlo experiment with a widely used DSGE model, the Smets and Wouters (2007) model (based on Christiano et al. 2005); the experiment assumes this model, with some error processes derived from US data, to be correct and generates from it many data samples of the size used in these tests. Then we check how models of progressive falseness would perform in forecasting tests on these data samples as compared with an unrestricted time series model – we use the most general one, the VAR. We wish to know how the OSF tests identify whether a model has both a good specification (i.e. is not too false) and a systematically good forecasting performance.
It is always possible that an experiment like this would give different conclusions with a different assumed model – a task for future research. However, the DSGE models used in these exercises are almost all of the Smets and Wouters structure, so it should not be misleading for the bulk of the OSF tests done so far.
OSF tests of a model’s specification
Table 1 summarises what we found about the OSF test’s ability to identify poor specification.
The table shows how frequently the model is rejected by the test with 95% confidence as its falseness increases to x% – this shows the power of the test against increasing model misspecification.
To create falseness the model’s parameters are all changed by +/-x% alternately.
The test is performed on GDP growth, inflation, and interest rates.
The relevant columns are the last two for the joint performance on the three together. There are two forecast horizons: 4 quarters ahead (4Q) and 8 ahead (8Q). Mostly we focus on 4Q because 8Q has extremely weak power. Even 4Q we see has low power; the rejection rate only rises above one-third when falseness has risen to 10%.
Table 1. Power of OSF test
GDP growth Inflation Interest rate Joint 3
%F 4Q 8Q %F 4Q 8Q %F 4Q 8Q %F 4Q 8Q
True 5.0 5.0 True 5.0 5.0 True 5.0 5.0 True 5.0 5.0
1 10.2 5.0 1 5.8 4.7 1 4.7 4.8 1 6.0 4.9
3 23.2 5.0 3 7.9 4.8 3 6.5 4.2 3 9.4 5.2
5 34.9 5.2 5 13.4 5.1 5 11.5 4.2 5 15.3 6.0
7 42.5 5.1 7 21.3 6.9 7 18.9 5.4 7 22.9 6
10 52.3 5.5 10 35.6 10.7 10 30.3 6.5 10 36.2 9.8
15 58.0 11.0 15 62.7 23.7 15 48.9 11.9 15 73.8 29.5
20 49.9 60.5 20 97.8 72.4 20 62.7 21.3 20 99.8 90.7
What this tells us is that OSF tests will not reliably identify bad models; it is a weak test of specification. To put it in perspective Table 2 shows its power side by side with an in-sample indirect inference test that Le et al. (2014) have found to be highly effective – this test rejects over half the time when misspecification reaches only 3%. The test is based on the model’s simulations and asks whether the simulated data behaves in the same way as the actual data in the sample, with some degree of statistical confidence.
Table 2. Rejection rates: Indirect inference and likelihood ratio for 3 variables
Joint 3
% misspecified Ind. inf. 4Q 8Q
True 5.0 5.0 5.0
1 19.8 6.0 4.9
3 52.1 9.4 5.2
5 87.3 15.3 6.0
7 99.4 22.9 6.6
10 100.0 36.2 9.8
15 100.0 73.8 29.5
20 100.0 99.8 90.7
Why might the OSF test have such weak power? In forecasting, DSGE models use fitted errors, and when the model is misspecified this creates larger errors which absorb the model’s misspecification; these new errors are projected into the future and could to some degree compensate for the poorer performance by the misspecified parameters. To put this another way, as the DSGE model produces larger errors, reducing the relative input from the structural model proper, these larger errors take on some of the character of an unrestricted VAR. By contrast, in indirect inference false errors compound the model’s inability to generate the same data features as the actual data.
OSF tests of a model’s forecasting capacity
This weak power of the OSF test implies that a model can be quite false and still forecast fairly well – the test ‘passes’ such models because they forecast better than the time series model. We found that there is a critical degree of falseness at which the DSGE model forecasts just as well but no better than the time series model – in this model’s case for the 4Q horizon it was 7%. The user interested only in the forecasting capacity of some model M would like to know whether model M is above or below this threshold of falseness.
There are two ways to establish this statistically. One may use the OSF test and check whether model M is rejected on the left-hand or right-hand tail of the OSF test (due to Diebold and Mariano 1995). If model M’s forecast performance lies in the left-hand tail, then one can confidently reject the hypothesis that model M forecasts better by chance; if it lies in the right-hand tail then model M forecasts worse by chance.
Table 3 shows the power of these two tail tests. We can see that the right-hand tail test has some power but the left-hand tail has very weak power. Hence false models that forecast much worse than time series are clearly identified by the test – but notice that they have to be really bad, at least 15–20% false. Meanwhile it is hard to be sure a model is systematically better at forecasting than time series, because the forecast performance of all models of 7% falseness or less is so similar. This accounts for the Gürkaynak et al. findings that it is hard to say for sure whether DSGE models are better or worse than time series.
Table 3. Power of OSF tests for left-hand tail and right-hand tail
Joint 3 -RH Tail Joint 3 -LH Tail
% F 4Q % F 4Q
True True 16.7
1 1 14.2
3 3 9.8
5 5 7.2
7 5.0 7 5.0
10 11.3 10
15 46.8 15
20 99.5 20
There is a neat solution to this question, however, if one is willing to use the indirect inference test to establish model M’s degree of falseness. Return to Table 2; against the 7% false model the indirect inference test has power of 99.4%. So if policymakers could find a DSGE model that was not rejected by this test, then they could have complete confidence that it would be at least as good at forecasting as a time series model! Such a model would also be reliable for policy assessment.
Conclusions
OSF tests are increasingly used to establish the quality of DSGE models – both their specification and their forecasting ability. In Minford et al. (2014), my coauthors and I assess via a Monte Carlo experiment on a widely used model what OSF tests can establish with confidence. We found that these tests cannot reliably distinguish quite seriously false models from each other and from the true model (they have weak power against misspecification); as far as forecasting ability goes, again they do not reliably distinguish either good models or bad models from a model that is just able to match time series performance – thus again they have quite weak power against both good and bad forecasting performers. This accounts for the ambivalent results of DSGE versus time series forecast comparisons. If users are willing to put their DSGE model to an in-sample indirect inference test, however, this can be used to establish reliably both the model’s specification quality and its forecasting capacity.
References
Christiano, L J, M Eichenbaum, C L Evans (2005), “Nominal Rigidities and the Dynamic Effects of a Shock to Monetary Policy”, Journal of Political Economy 113(1): 1–45.
Diebold, F X and R S Mariano (1995), “Comparing Predictive Accuracy”, Journal of Business and Economic Statistics 13: 253–263.
Edge, R M and R S Gürkaynak (2010), “How Useful Are Estimated DSGE Model Forecasts for Central Bankers?”, Brookings Papers on Economic Activity 41(2): 209–259.
Gürkaynak, R S, B Kisacikoglu, and B Rossi (2013), “Do DSGE models forecast more accurately out-of-sample than VAR models?”, CEPR Discussion Paper 9576, July.
Hansen, L P and J J Heckman (1996), “The empirical foundations of calibration”, Journal of Economic Perspectives 10(1): 87–104.
Le, V P M, D Meenagh, P Minford, and M Wickens (2014), “Testing DSGE models by indirect inference and other methods: some Monte Carlo experiments”, Cardiff Economics Working Paper E2012/15, updated 2014.
Minford, P, Y Xu and P Zhou (2014), “How good are out of sample forecasting tests?”, CEPR Discussion Paper 10239.
Smets, F and R Wouters (2007), “Shocks and Frictions in US Business Cycles: A Bayesian DSGE Approach”, American Economic Review 97(3): 586–606.
This article is published in collaboration with VoxEU. Publication does not imply endorsement of views by the World Economic Forum.
To keep up with Forum:Agenda subscribe to our weekly newsletter.
Author: Patrick Minford is Professor of Economics at Cardiff University where he directs the Julian Hodge Institute of Applied Macroeconomics.
Image: A man walks past buildings at the central business district of Singapore February 14, 2007. REUTERS.
Don't miss any update on this topic
Create a free account and access your personalized content collection with our latest publications and analyses.
License and Republishing
World Economic Forum articles may be republished in accordance with the Creative Commons Attribution-NonCommercial-NoDerivatives 4.0 International Public License, and in accordance with our Terms of Use.
The views expressed in this article are those of the author alone and not the World Economic Forum.
Stay up to date:
Economic Progress
The Agenda Weekly
A weekly update of the most important issues driving the global agenda
You can unsubscribe at any time using the link in our emails. For more details, review our privacy policy.
More on Economic GrowthSee all
Harsh Vijay Singh and Attilio Di Battista
November 15, 2024