How will deep learning change the industrial economy?
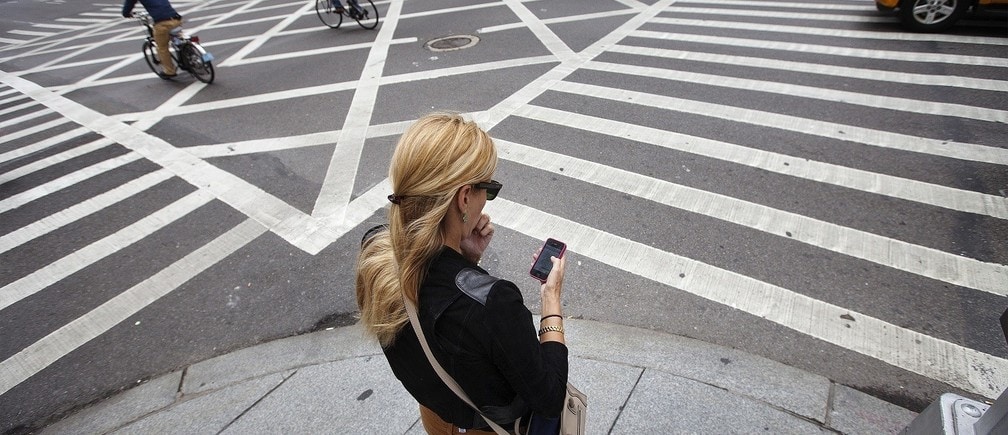
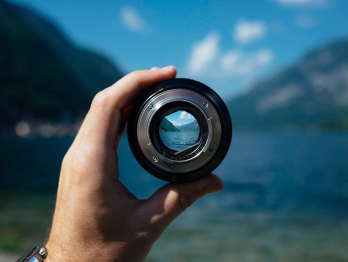
Get involved with our crowdsourced digital platform to deliver impact at scale
Stay up to date:
Hyperconnectivity
Computers are increasingly able to learn on their own and are now superior to humans in certain image-recognition tasks. Jeremy Howard, CEO of Enlitic, is exploring these capabilities for medical applications. He was an early adopter of neural-network and big-data methodologies in the 1990s. As the president & chief scientist of Kaggle, a platform for data science competitions, he witnessed the rise of an algorithmic method called “deep learning”. In 2012, a team led by Geoffrey Hinton of the University of Toronto caught the world’s attention when it used deep learning to win a contest sponsored by Merck on automatic drug discovery. Remarkably, no one on the team had any domain knowledge of pharmaceuticals or molecular chemistry. Mr Howard tells Look ahead about the effect deep learning will have on the industrial economy and some of the future challenges he foresees to capturing these benefits.
New developments using predictive algorithms based on data collected via sensor networks and the Internet can further optimise global value chains (GVCs). In the future, robots and 3D printing might change the way we think about skilled labour and mass production and reshape GVCs into regional value chains.
When did you first become aware of deep learning?
I’ve actually been watching it for over 20 years. As you might know, deep learning is simply an evolution of neural networks with more layers. I used neural networks in the early-to-mid 1990s. I was in consulting at the time, and we had a lot of success improving the targeting of marketing applications. What happened in 2012 was that for the first time deep neural networks started becoming good at things that previously only humans were able to do, particularly at understanding the content of images. Image recognition may sound like a fairly niche application, but when you think about it, it is actually critical. Computers before were blind. Today they are now more accurate and much faster at recognising objects in pictures than humans are.
What kinds of problems in the industrial economy can deep learning solve effectively?
There are three particular areas where this ability to understand images is a very big deal. One is in satellite imagery, whether for agriculture, intelligence or mapping. Deep learning can automate much of that now.
The second, even more excitingly, is in robotics. We can now have things like self-driving cars or machines that can automatically prepare food. Giving robots the ability to see is going to open up a whole massive area there.
The third is in medicine. Understanding what you are looking at is critical to diagnosing and treating disease. Applications include radiology (looking at the inside of the body using X-rays or MRIs), pathology (looking at tissue through a microscope) and dermatology (looking at pictures of skin).
It takes literally decades for humans to see enough examples of things so that they can accurately pick up what’s going on, for example, in a MRI. Computers, on the other hand, can look at 50m MRIs and understand every kind of disease as it appears in every kind of person at every stage of time, and can, therefore, be as good as the best radiologist in every single sub-specialty.
They can allow the physician, or even a nurse in some remote province of China, to deeply understand what’s going on in a medical image. Healthcare, in general, is an almost $10trn industry—that’s $3trn in the US—possibly the largest industry in the world. The fact that we can now use deep learning to understand medical data in this way is going to be totally world changing.
Healthcare, in general, is an almost a $10trn industry—that’s $3trn in the US—possibly the largest industry in the world. The fact that we can now use deep learning to understand medical data in this way is going to be totally world changing.
Of the sectors you mentioned, which do you feel will be impacted most by deep learning?
I suspect long-term robotics will be where the impact is greatest. Having devices that can see impacts the large percentage of the world’s employment that relies on human vision. I suspect that long-term robotics will impact everybody in the world, every day, all the time.
Medicine is kind of a close second. We can use deep learning to understand the genome, imaging, lab tests and provide answers to doctors’ questions. This will allow doctors to have the kind of decision-support tool that they dream about.
When I looked at robotics, many people that I respected were working on it and making quick progress. But you don’t see this kind of progress in medicine. Obviously there’s Watson, but that really was designed to win Jeopardy. It was never designed to be good at medicine. As a result, it can’t handle something like imaging, so they have been struggling to fill these huge gaps.
I suspect long-term robotics will be where the impact is greatest. Having devices that can see impacts the large percentage of the world’s employment that relies on human vision. I suspect that long-term robotics will impact everybody in the world, every day, all the time.
More and more of the data that we’re dealing with in the world are time-based and continuous. Deep learning has mostly been used on large batches of static data, like a million images of cats. Is it also applicable to time-based and real-time data?
It’s particularly good at that, actually. The reason it’s mainly been used in imaging so far is an accident of history. In 2012, a competition was won using deep learning in image recognition. As a result, many people in computer vision switched their research to deep learning. It is just as useful for language or time-based signals. These models are trained that every time you present a new data point it is incorporated into an updated model very quickly.
The challenge is that there have not been many people with a good enough technical understanding of deep learning to apply it to domains it hasn’t been applied to before. For 20 years, there were only five university labs in the world working on it. Today those five labs are very famous [Ed note: the five are University of Toronto, Université de Montréal, New York University, Stanford University and University of Oxford]. Everybody who comes out of them gets picked up by Google, or Facebook or a top university. We haven’t even had enough time for our first PhD students to come out of programmes that have grown since 2012. There aren’t yet the technical resources available to apply these tools to new areas. There’s a huge opportunity industrially for people to build out this stuff. It reminds me of the early days of the Internet, that’s where we’re at with deep learning right now.
What are the differences between the ways that humans and machines learn? Are there specific weaknesses of the deep-learning approach that you are aware of?
The difference between humans and machines is that once you create a cat-detector module, you don’t have to have every machine learn it. We can download these networks between machines, but we can’t download knowledge between brains. This is a huge benefit of deep-learning machines that we refer to as “transfer learning”.
The real missing pieces in deep learning are twofold. The first is very simple: We need better data access. In medicine. you spend the most time not on how to train the model to recognise new diseases, but in putting together the data sets that have enough examples of diseases. The data actually exist in the world, but they are sitting on thousands of disconnected hospital networks. The legislation, which was developed to ensure medical data privacy, didn’t foresee that we could save millions of lives by aggregating data to train these neural networks. We have this opportunity cost of millions of lives. What we’re trying to do at Enlitic is to work directly with big hospital networks to access that data through research agreements so that we can create these life-saving networks.
The second thing that’s missing is technical: the ability to do logic. We can recognise things very well with deep learning—they’re a great kind of analogy machine. But they are not able to use logic to understand unexpected things in a particular image. The human brain is very good at making guesses, because we understand the context of how the world works and how things fit together. At the moment we don’t have that kind of contextual understanding in our deep-learning systems.
You teach at Singularity University and you specifically teach about deep learning as an “exponentially advancing technology”. What resources need to be unlimited for the exponential growth of deep learning?
That’s a really good question. I actually think deep learning could be the first truly exponential technology.
Most technologies are not exponential. Many start off looking exponential, but they always hit some kind of resource constraint, resulting in an S-shaped growth curve that eventually asymptotes to a maximum. The steam engine of the Industrial Revolution, for example, had an exponential growth at the start, but after a while most of the energy inputs had been replaced and the growth stopped.
With deep learning there’s a mathematical proof that it can model anything that can be modelled as long as it has enough computing capacity and data to learn it. Instead of being a physical engine, it is an intellectual engine.
The inputs to this are energy and data. Obviously there’s an infinite amount of data in the universe. There’s not an unlimited amount of energy, but there’s quite a lot of it. It’s going to be a long time before our ability to compute is limited by the amount of energy that we can harness. The computations will become more and more efficient as we get better and better at them.
With deep learning, as you add more layers to the network they increase super-linearly in terms of what they can model. You get increasing returns from larger networks. The intellectual capacity of these things moves beyond us at an increasing rate. We can then harness much greater amounts of energy much more efficiently to create even better deep-learning networks.
There is now, in fact, a deep-learning network for building deep-learning networks! And, indeed, the networks that they come up with are, in fact, much better than those that humans have created.
…deep learning could be the first truly exponential technology.
I understand you’ve been teaching yourself Chinese through a very data-intensive learning algorithm. I’m wondering how this personal learning experience, in your own brain, has informed your ideas about deep learning in silico.
The reason I learned Chinese is actually not that I had any interest in Chinese at all. I wanted to learn more about human learning, so that I could use that knowledge to increase my machine-learning ability. About six years ago, I set out to do a project of study that could last a really long period of time. I picked Chinese because it’s one of the two hardest languages to learn for English speakers, along with Arabic. I spent three months studying human-learning theory, and then I spent three months writing software trying to implement what I had learned, and then I started learning Chinese. As it turned out, luckily, I really like Chinese.
Human-learning theory really is all about the power of creating additional connectivity in the brain, using things like mnemonics and context to use stuff we already learned to help us learn other things. It’s coloured my understanding of the transfer learning that we talked about. For me, the most exciting work in deep learning right now is this ability to transfer knowledge across networks and have networks that are continually getting better, not just at one particular thing but at new things as well.
This article is published in collaboration with GE Look Ahead. Publication does not imply endorsement of views by the World Economic Forum.
To keep up with the Agenda subscribe to our weekly newsletter.
Author: Anthony Wing Kosner is a contributor to GE Look Ahead.
Image: A woman uses her phone while waiting to cross 5th Avenue in New York. REUTERS/Lucas Jackson.
Don't miss any update on this topic
Create a free account and access your personalized content collection with our latest publications and analyses.
License and Republishing
World Economic Forum articles may be republished in accordance with the Creative Commons Attribution-NonCommercial-NoDerivatives 4.0 International Public License, and in accordance with our Terms of Use.
The views expressed in this article are those of the author alone and not the World Economic Forum.
The Agenda Weekly
A weekly update of the most important issues driving the global agenda
You can unsubscribe at any time using the link in our emails. For more details, review our privacy policy.
More on Industries in DepthSee all
Mandy Chan and Daniel Boero Vargas
June 25, 2024
John Lombard
June 24, 2024
Pooja Chhabria
June 23, 2024
Liam Coleman
June 4, 2024