Algorithms can be sexist too. This is what we can do about it
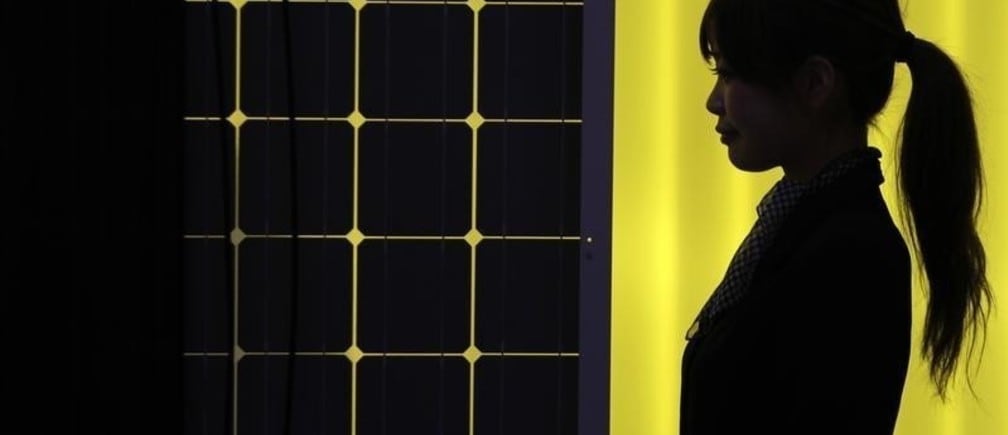
The algorithm system can potentially amplify gender stereotypes.
Image: REUTERS/Yuriko Nakao
Stay up to date:
Fourth Industrial Revolution
Machine learning is ubiquitous in our daily lives. Every time we talk to our smartphones, search for images or ask for restaurant recommendations, we are interacting with machine learning algorithms. They take as input large amounts of raw data, like the entire text of an encyclopedia, or the entire archives of a newspaper, and analyze the information to extract patterns that might not be visible to human analysts. But when these large data sets include social bias, the machines learn that too.
A machine learning algorithm is like a newborn baby that has been given millions of books to read without being taught the alphabet or knowing any words or grammar. The power of this type of information processing is impressive, but there is a problem. When it takes in the text data, a computer observes relationships between words based on various factors, including how often they are used together.
We can test how well the word relationships are identified by using analogy puzzles. Suppose I ask the system to complete the analogy “He is to King as She is to X.” If the system comes back with “Queen,” then we would say it is successful, because it returns the same answer a human would.
Our research group trained the system on Google News articles, and then asked it to complete a different analogy: “Man is to Computer Programmer as Woman is to X.” The answer came back: “Homemaker.”
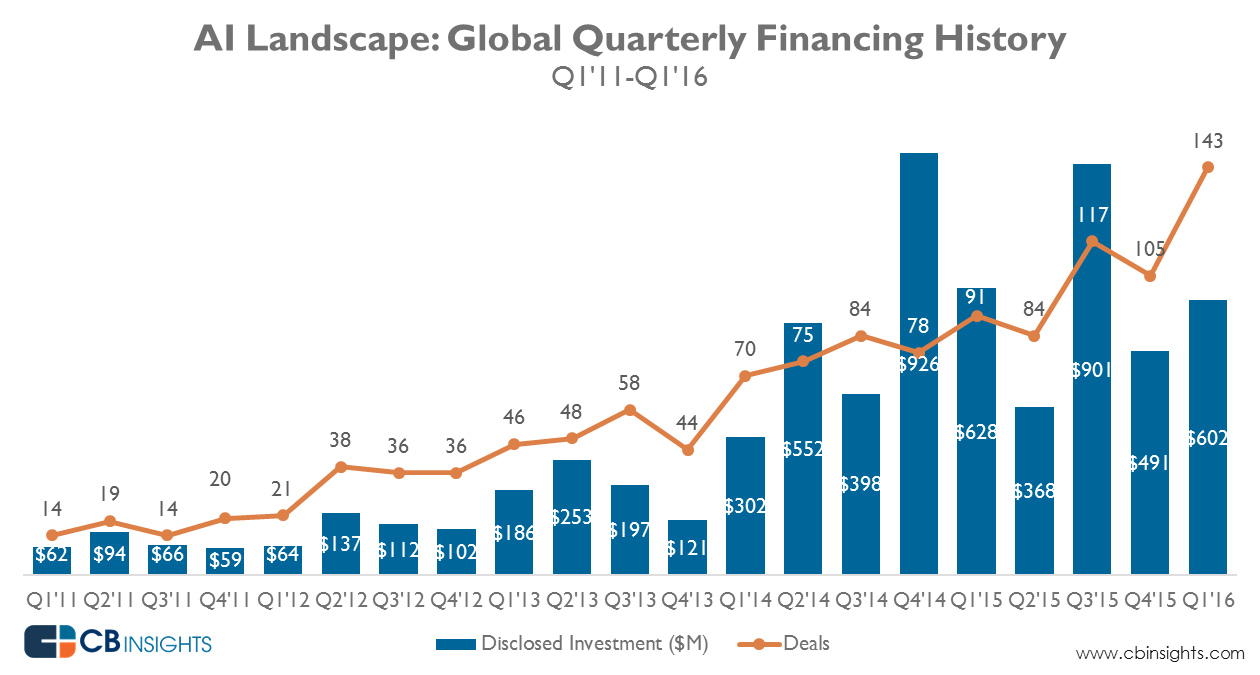
We used a common type of machine learning algorithm to generate what are called “word embeddings.” Each English word is embedded, or assigned, to a point in space. Words that are semantically related are assigned to points that are close together in space. This type of embedding makes it easy for computer programs to quickly and efficiently identify word relationships.
After finding our computer programmer/homemaker result, we asked the system to automatically generate large numbers of “He is to X as She is to Y” analogies, completing both portions itself. It returned many common-sense analogies, like “He is to Brother as She is to Sister.” In analogy notation, which you may remember from your school days, we can write this as “he:brother::she:sister.” But it also came back with answers that reflect clear gender stereotypes, such as “he:doctor::she:nurse” and “he:architect::she:interior designer.”
The fact that the machine learning system started as the equivalent of a newborn baby is not just the strength that allows it to learn interesting patterns, but also the weakness that falls prey to these blatant gender stereotypes. The algorithm makes its decisions based on which words appear near each other frequently. If the source documents reflect gender bias – if they more often have the word “doctor” near the word “he” than near “she,” and the word “nurse” more commonly near “she” than “he” – then the algorithm learns those biases too.
Making matters worse
Not only can the algorithm reflect society’s biases – demonstrating how much those biases are contained in the input data – but the system can potentially amplify gender stereotypes. Suppose I search for “computer programmer” and the search program uses a gender-biased database that associates that term more closely with a man than a woman.
The search results could come back flawed by the bias. Because “John” as a male name is more closely related to “computer programmer” than the female name “Mary” in the biased data set, the search program could evaluate John’s website as more relevant to the search than Mary’s – even if the two websites are identical except for the names and gender pronouns.
It’s true that the biased data set could actually reflect factual reality – perhaps there are more “Johns” who are programmers than there are “Marys” – and the algorithms simply capture these biases. This does not absolve the responsibility of machine learning in combating potentially harmful stereotypes. The biased results would not just repeat but could even boost the statistical bias that most programmers are male, by moving the few female programmers lower in the search results. It’s useful and important to have an alternative that’s not biased.
Removing the stereotypes
If these biased algorithms are widely adopted, it could perpetuate, or even worsen, these damaging stereotypes. Fortunately, we have found a way to use the machine learning algorithm itself to reduce its own bias.
Our debiasing system uses real people to identify examples of the types of connections that are appropriate (brother/sister, king/queen) and those that should be removed. Then, using these human-generated distinctions, we quantified the degree to which gender was a factor in those word choices – as opposed to, say, family relationships or words relating to royalty.
Next we told our machine-learning algorithm to remove the gender factor from the connections in the embedding. This removes the biased stereotypes without reducing the overall usefulness of the embedding.
When that is done, we found that the machine learning algorithm no longer exhibits blatant gender stereotypes. We are investigating applying related ideas to remove other types of biases in the embedding, such as racial or cultural stereotypes.
Accept our marketing cookies to access this content.
These cookies are currently disabled in your browser.
Don't miss any update on this topic
Create a free account and access your personalized content collection with our latest publications and analyses.
License and Republishing
World Economic Forum articles may be republished in accordance with the Creative Commons Attribution-NonCommercial-NoDerivatives 4.0 International Public License, and in accordance with our Terms of Use.
The views expressed in this article are those of the author alone and not the World Economic Forum.
Forum Stories newsletter
Bringing you weekly curated insights and analysis on the global issues that matter.
More on Emerging TechnologiesSee all
Robert Opp and Keyzom Ngodup Massally
April 4, 2025
Ibrahim Abdullah Alshunaifi and Caroline Tasse
April 3, 2025
David Elliott
April 2, 2025