We're asking all the wrong questions about the future of jobs
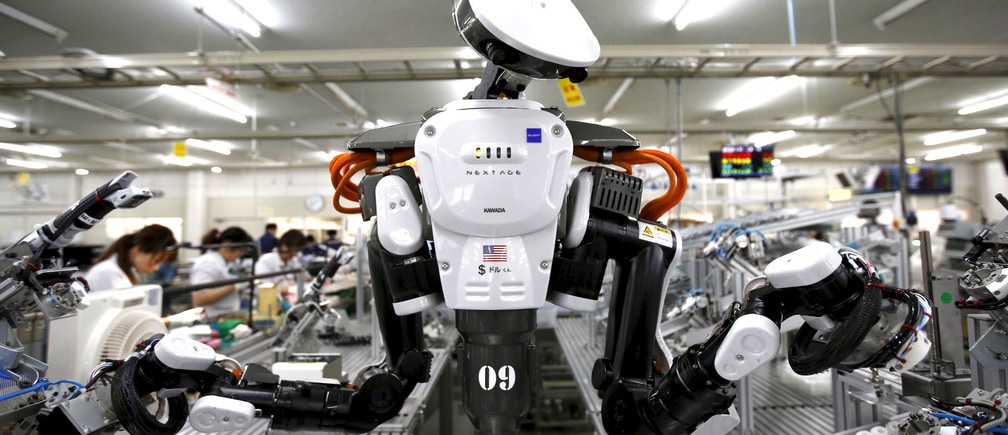
A humanoid robot works side by side with employees on a Japanese assembly line Image: REUTERS/Issei Kato
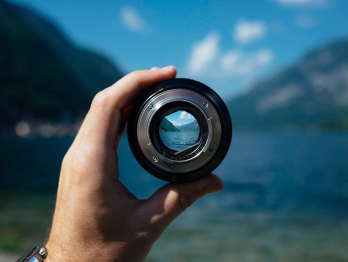
Get involved with our crowdsourced digital platform to deliver impact at scale
Stay up to date:
The Digital Economy
Work is not what it used to be. The very concept of work has evolved considerably over the centuries, as newer technologies have become integrated into how we function individually as well as a society.
In engineering, work is defined as the product of force and distance. Early machines amplified force and turbocharged human productivity, providing an early platform for industrialised society. They eliminated physical grunt work and created new kinds of work for humans, such as operating and fixing machines and using their capabilities in new ways. Computers have similarly reduced our informational grunt work, enabling more productive, creative, and rewarding work for humans.
But today’s machines are different in that they are increasingly figuring things out for themselves. They have learned how to learn, a quality that has until now been exclusive to humans. Our ability to perceive the world and integrate unstructured information spontaneously serves as the bedrock of much of human work. With machines now performing this role, often better than us, how valuable will the human - or ‘nonroutine’ - aspects of work be in the future?
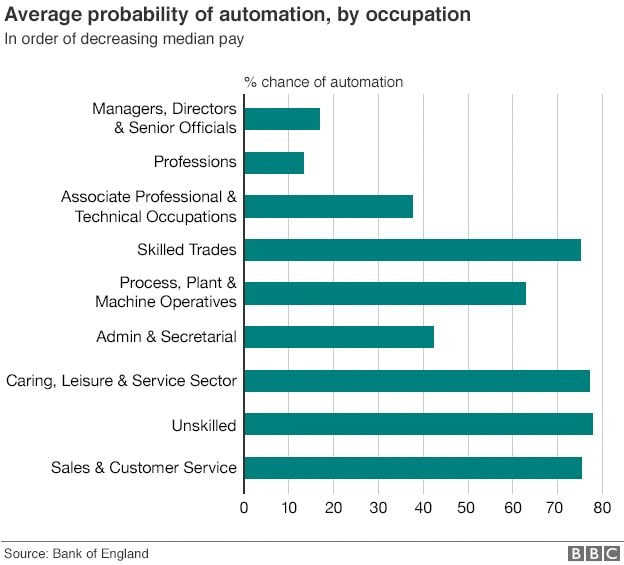
The current political preoccupation with job numbers ignores the quality distribution of such jobs. Of the 50,000 people Amazon is looking to hire immediately, for example, what proportion has the potential for real career and earnings growth?
It isn’t possible to answer this question based on past data, because nonroutine work does not lend itself well to scientific management-style observation, or the standard occupational descriptions as laid down by the O*NET occupational classification system. Technology has altered the composition of jobs and the associated skills required; the work of an accountant or lawyer today, for example, is very different from what it was a decade or two ago.
The skill requirements of jobs change as what was nonroutine becomes routine. The superior far-distance vision capability of professional drivers, for example, diminishes in value if a machine can see better than humans, and a superior ability to memorise precedents is less valuable for lawyers if a machine can find them faster and better than humans. In order to predict what impact artificial intelligence (AI) will have on human work, we need to understand at a detailed level the ‘digital fingerprint’ associated with work, and especially nonroutine work.
Past research shows that automation has replaced routine tasks while complementing nonroutine ones. Furthermore, the share of the labour force employed in occupations that make intensive use of nonroutine tasks has increased substantially over the last few decades, while those involving mostly routine cognitive and manual tasks have declined substantially. If this is how the future will unfold, one might reasonably project that the nature of human employment will depend on the supply, and in particular the demand, for nonroutine task-oriented occupations.
But this routine/nonroutine view of the world is blurry and breaks down when autonomous learning systems – as opposed to automated systems – become capable of performing tasks previously considered nonroutine. In 1972 the philosopher Hubert Dreyfus asserted that computers would never be able to perform simple tasks such as driving. As recently as 10 years ago, driving a vehicle was considered nonroutine and therefore inherently human. And yet autonomous driving vehicles are about to make navigation largely routine. More generally, machines are becoming better at other cognitive tasks long considered human, including investment decision making, creating tax returns, and more. Big data, especially perception-based data from systems that can see, hear, read, and touch, is providing machines with the ability to learn on their own.
A recent report by the McKinsey Global Institute reported that 60% of occupations are 30% automatable. The report notes that such occupations make up almost $2.7 trillion in wages in the United States, slightly more than half the country’s total economic activity. The report also envisions humans working alongside machines in new ways. Indeed, AI machines are already redefining the boundaries of physical as well as cognitive work, helping humans with the time-consuming or error-prone components of work. But when machines become much better than us at making important nonroutine decisions involving our health, finance, navigation or entertainment, for example, what value will humans add?
At universities, we teach students how to think in order to prepare them for the future workplace. This is largely about how to conduct inquiry scientifically, which begins by asking the right questions, formulating the problem, execution, analysis of outputs, and communication of results. Some researchers have proposed that we should encourage computational thinking, which considers these steps as a whole by “reformulating a seemingly difficult problem into one we know how to solve, perhaps by reduction, embedding, transformation, or simulation”, according to Jeannette Wing, head of the computer science department at Carnegie Mellon University. Existing skills-based descriptions of work don’t adequately address these increasingly important aspects of nonroutine knowledge work.
My research on the boundary between situations where we trust and those where we don’t trust machines with decision making – which I call the ‘decision automation frontier’ – depends on how often we expect the machine to be wrong and what the consequences of that might be, especially in the worst case. We trust machines when they perform at a predictable rate of accuracy and the cost of their errors is sufficiently low. For example, searching for precedents for legal cases or ranking outcome predictions might be effectively performed by machines, but tasks such as preparing the argumentation for how a legal case is presented are human.
Interestingly, the O*NET work taxonomy categorises lawyers as requiring high levels of oral and written expression and comprehension, stress tolerance, and integrity. Imagine if we could take a bottom-up ‘digital snapshot’ of all legal work and allow the features to emerge from the data? For example, what proportion of lawyers' time is spent on aspects of work such as researching, writing and talking, and how are these valued by the labour market? The measurement of cognitive activity associated with nonroutine work, which I have termed the digital fingerprint of work, is a fertile area for research. Better instrumentation will yield a data-driven picture of what jobs actually consist of, and a basis for predicting how they will change.
The digital fingerprint of work will undoubtedly vary across professions. For healthcare professionals, nonroutine tasks might involve deep knowledge or medical skills, as well as skills such as empathy and communication. For insurance professionals it might involve an ability to assess risk based on records and subtle cues. In sports it might involve an analysis of decision-making under pressure. In principle, these are all observable and measurable to various degrees. But more research is needed to understand how humans and smarter machines will complement and compete with each other at a micro level before we have the basis for making predictions about the future of work.
Don't miss any update on this topic
Create a free account and access your personalized content collection with our latest publications and analyses.
License and Republishing
World Economic Forum articles may be republished in accordance with the Creative Commons Attribution-NonCommercial-NoDerivatives 4.0 International Public License, and in accordance with our Terms of Use.
The views expressed in this article are those of the author alone and not the World Economic Forum.
The Agenda Weekly
A weekly update of the most important issues driving the global agenda
You can unsubscribe at any time using the link in our emails. For more details, review our privacy policy.
More on Jobs and the Future of WorkSee all
Peter Brown, Kathy Parker and Harriet Newlyn
July 15, 2024
Don Howard and George Zuo
July 9, 2024
James Fell and Linda Lacina
July 3, 2024
Sarah Hewitt
June 25, 2024
Patrick Tsang
June 25, 2024
Gayle Markovitz
June 23, 2024