Artificial intelligence can make our societies more equal. Here’s how
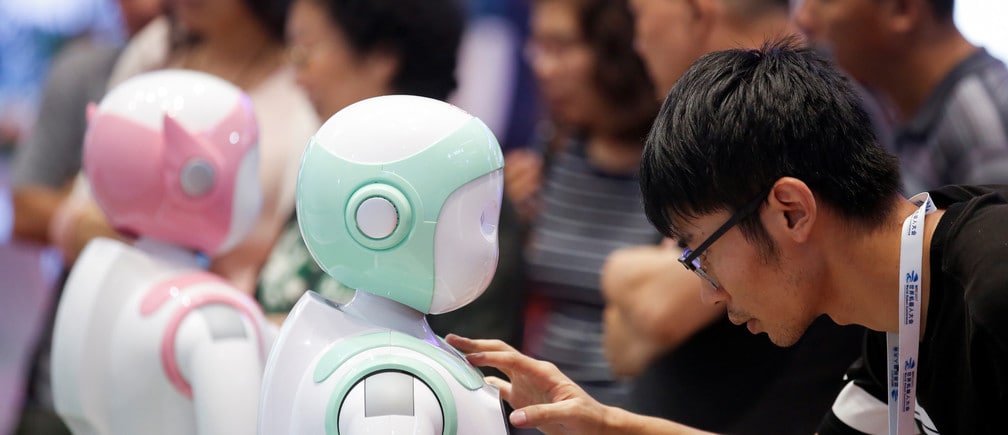
Image: REUTERS/Thomas Peter
Stay up to date:
The Digital Economy
Artificial intelligence is taking on a more central role in high-stakes decision-making within our most critical social institutions. It has entered into our hospitals, courthouses, and employment offices, deciding who gets insurance, who receives parole, and who gets hired. While in many cases use of AI is intended to increase efficiency and effectiveness by overcoming errors and biases inherent in human decision-making, risks of algorithmic bias—when an algorithm takes on the prejudices of its creators or the data it is fed—may amplify discrimination, not correct for it.
We must recognize that algorithms are not neutral. They reflect the data and assumptions inherent in their calculations. If prejudiced data is fed into an algorithm or factors that reflect existing social biases are prioritized, discriminatory results will follow. Algorithms function by prioritizing certain factors—identifying statistical patterns from observed and latent variables and subsequently offering “if this, then that” conclusions. By assuming that certain factors are appropriate predictors of an outcome and historical trends will be repeated, an algorithm can exhibit a self-reinforcing bias. For those who are over-, under- or misrepresented in the data and calculations, decisions made on their behalf can perpetuate inequality.
People worry that computers will get too smart and take over the world, but the real problem is that they’re too stupid and they’ve already taken over the world.
”- Pedro Domingos, The Master Algorithm
To put this in context, let’s take a look at predictive policing models and health insurance risk predictions. Predictive policing models use historical crime records, including date, time, and location to generate predicted crime hotspots. Since minority and low-income communities are far more likely to have been surveilled by police than prosperous white neighbourhoods, historical crime data at the core of predictive policing will provide a biased picture, presenting higher crime rates in communities that have been more heavily patrolled. As a result, predictive policing may amplify racial bias by perpetuating surveillance of minority and low-income communities.
In the case of health insurance, insurers can now predict an individual’s future health risks through the combination of thousands of non-traditional “third party” data sources, such as buying history and the health of their neighbours. While use of this data may accurately predict risk for the insurer, this also means that at-risk individuals may be charged premiums they cannot afford or will be denied coverage altogether. For those living in communities that have faced systemic health challenges, these predictive models may serve to perpetuate health disparities.
As AI is increasingly applied to make consequential decisions that affect social, political, and economic rights, it is imperative that we ensure these systems are built and applied in ways that uphold principles of fairness, accountability, and transparency. There are two ways to better ensure these principles are embedded into AI, leading not only to more efficient but also more equitable decision-making.
Broadly speaking, bias inserts itself into algorithms through the incorporation of value-laden data and prioritization of subjective factors. Datasets that are incomplete, non-standardized, or collected with faulty measurement tools can present a false reflection of reality. And data collected on a process that is itself reflective of longstanding social inequality will likely perpetuate it.
For example, an algorithm trained with a dataset from an industry that tended to hire and promote Caucasian males may result in systematic prioritization of these candidates over others. By analyzing data and assumptions through a “social-systems analysis” approach—where developers question, and correct for, the impacts of systemic social inequalities on the data AI systems are trained on—biases may be identified and corrected for earlier, lowering the risks of entrenching discrimination through AI. This leads to the next recommendation that more diverse teams will be more capable of identifying bias.
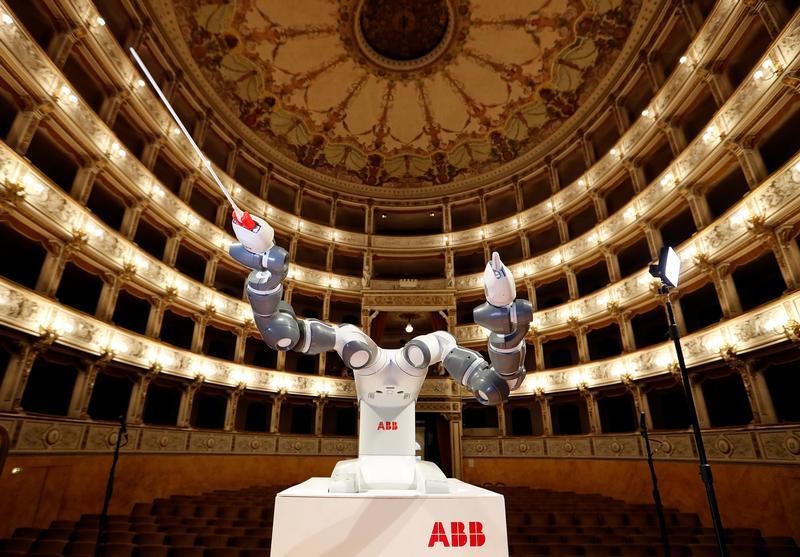
Diversity should be incorporated at every stage of the AI process, from design and deployment to questioning its impacts on decision-making. Research has shown that more diverse teams are more efficient at problem solving, regardless of cumulative IQ. Explicit attention to inclusivity in design, application, and evaluation of the effects of AI-enabled decision-making will not only minimize inadvertent discriminatory effects, but can also lead to its design and application as a driving force for greater social, economic, and political inclusion.
Artificial intelligence is at an inflection point. Its development and application can lead to unprecedented benefits for global challenges such as climate change, food insecurity, healthcare, and education. But its application must be carefully managed, ensuring it leads to a more equitable digital economy and society, not a more discriminatory one.
Don't miss any update on this topic
Create a free account and access your personalized content collection with our latest publications and analyses.
License and Republishing
World Economic Forum articles may be republished in accordance with the Creative Commons Attribution-NonCommercial-NoDerivatives 4.0 International Public License, and in accordance with our Terms of Use.
The views expressed in this article are those of the author alone and not the World Economic Forum.
Forum Stories newsletter
Bringing you weekly curated insights and analysis on the global issues that matter.
More on Emerging TechnologiesSee all
Spencer Feingold
March 26, 2025
Adam Hildreth and Julie Inman Grant
March 26, 2025
Yair Reem
March 25, 2025
Marie Penelope Nezurugo
March 25, 2025
Yuval Rooz
March 24, 2025