How can we build machines that feel empathy?
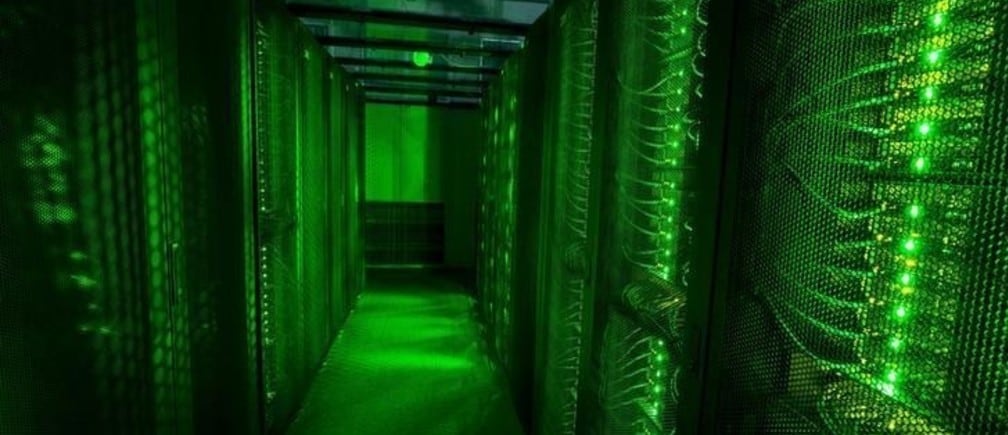
Mechanical learning systems might be the only way for governments to maintain ethical standards. Image: REUTERS/Sigtryggur Ari
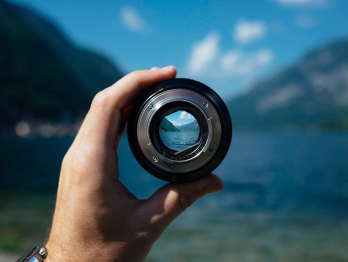
Get involved with our crowdsourced digital platform to deliver impact at scale
Stay up to date:
The Digital Economy
Machine learning algorithms work blindly towards the mathematical objective set by their designers. It is vital that this task include the need to behave ethically.
Such systems are exploding in popularity. Companies use them to decide what news you see and who you meet online dating. Governments are starting to roll out machine learning to help deliver government services and to select individuals for audit.
Yet the algorithms that drive these systems are much simpler than you might realise: they have more in common with a pocket calculator than a robot from a sci-fi novel by Isaac Asimov. By default, they don’t understand the context in which they act, nor the ethical consequences of their decisions.
The predictions of a machine learning algorithm come from generalising example data, rather expert knowledge. For example, an algorithm might use your financial situation to predict the chance you’ll default on the loan. The algorithm would be “trained” on the finances of historical customers who did or did not default.
For this reason, a machine learning system’s ethics must be provided as an explicit mathematical formula. And it’s not a simple task.
Learning from data
Data61, where I work, has designed and built machine learning systems for the government, as well as local and international companies. This has included several projects where the product’s behaviour has ethical implications.
Imagine a university that decides to take a forward-looking approach to enrolling students: instead of basing their selection on previous marks, the university enrols students it predicts will perform well.
The university could use a machine learning algorithm to make this prediction by training it with historical information about previous applicants and their subsequent performance.
Such training occurs in a very specific way. The algorithm has many parameters that control how it behaves, and the training involves optimising the parameters to meet a particular mathematical objective relating to the data.
The simplest and most common objective is to be able to predict the training data accurately on average. For the university, this objective would have its algorithm predict the marks of the historical applicants as accurately as possible.
Ethical objectives
But a simple predictive goal such as “make the smallest mistakes possible” can inadvertently produce unethical decision-making.
Consider a few of the many important issues missed by this often-used objective:
1. Different people, different mistakes
Because the algorithm only cares about the size of its mistakes averaged over all the training data, it might have very different “accuracies” on different kinds of people.
This effect often arises for minorities: there are fewer of them in the training data, so the algorithm doesn’t get penalised much for poorly predicting their grades. For a university predicting grades in a male-dominated course, for example, it might be the case that the algorithm is 90% accurate overall, but only 50% accurate for women.
To address this, the university would have to change the algorithm’s objective to care equally about accuracy for both men and women.
2. The algorithm isn’t sure
Simple machine learning algorithms provide a “best guess” prediction, but more sophisticated algorithms are also able to assess their own confidence in that prediction.
Ensuring that confidence is accurate can also be an important part of the algorithm’s objective. For example, the university might want to apply an ethical principle like “the benefit of the doubt” to applicants with uncertain predicted marks.
3. Historical bias
The university’s algorithm has learned to predict entirely from historical data. But if professors giving out the marks in this data had biases (say against a particular minority), then new predictions would have the same bias.
The university would have to remove this bias in its future admissions by changing the algorithm’s objective to compensate for it.
4. Conflicting priorities
The most difficult factor in creating an appropriate mathematical objective is that ethical considerations often conflict. For the university, increasing the algorthm’s accuracy for one minority group will reduce its accuracy for another. No prediction system is perfect, and their limitations will always affect some students more than others.
Balancing these competing factors in a single mathematical objective is a complex issue of judgement with no single answer.
Building ethical algorithms
These are only a few of the many complex ethical considerations for a seemingly straightforward problem. So how does this university, or a company or government, ensure the ethical behaviour of their real machine learning systems?
As a first step, they could designate an “ethics engineer”. Their job would be to elicit the ethical requirements of the system from its designers, convert them into a mathematical objective, and then monitor the algorithm’s ability to meet that objective as it moves into production.
Unfortunately, this role is now lumped into the general domain of the “data scientist” (if it exists at all), and does not receive the attention it deserves.
Creating an ethical machine learning system is no simple task: it requires balancing competing priorities, understanding social expectations, and accounting for different types of disadvantage. But it is the only way for governments and companies to ensure they maintain the ethical standards society expects of them.
Don't miss any update on this topic
Create a free account and access your personalized content collection with our latest publications and analyses.
License and Republishing
World Economic Forum articles may be republished in accordance with the Creative Commons Attribution-NonCommercial-NoDerivatives 4.0 International Public License, and in accordance with our Terms of Use.
The views expressed in this article are those of the author alone and not the World Economic Forum.
Related topics:
The Agenda Weekly
A weekly update of the most important issues driving the global agenda
You can unsubscribe at any time using the link in our emails. For more details, review our privacy policy.
More on Emerging TechnologiesSee all
Henry Ajder
July 19, 2024
Sebastian Buckup
July 18, 2024
Nii Simmonds and Ayodele Okeowo
July 17, 2024
Jerome Desbonnet and Oded Vanunu
July 16, 2024
Tariq Malik and Prerna Saxena
July 12, 2024
Stefanie Ólives
July 12, 2024