AI could reinforce gender inequality
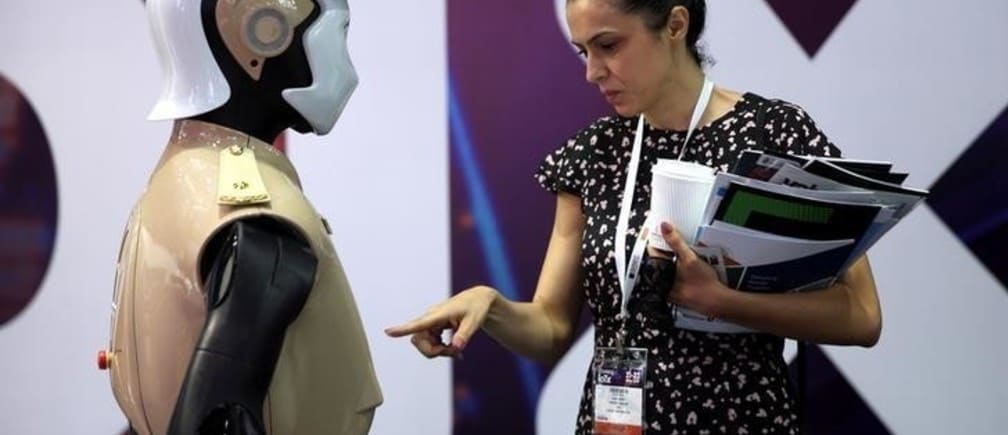
Data being used to train machines is often biased. Image: REUTERS/Stringer
We are not only living in an age where women are being under-represented in many spheres of economic life, but technology could make this even worse. Women hold just 19% of board directorships in the US and Europe. This gender gap in the boardroom persists, despite the fact that, on average, women have obtained higher educational qualifications than their male counterparts for more than two decades in many OECD countries. And the main reason is social bias.
This is on the verge of being further reinforced by artificial intelligence, as current data being used to train machines to learn are often biased.
With the rapid deployment of AI, this biased data will influence the predictions that machines make. Whenever you have a dataset of human decisions, it naturally includes bias. This could include hiring decisions, grading student exams, medical diagnosis, loan approvals. In fact anything described in text, in image or in voice requires information processing – and this will be influenced by cultural, gender or race biases.
AI in action
The way machines learn, a subfield of AI, involves feeding the computer sets of data –whether it’s in the form of text, images or voice – and adding a classifier to this data. An example would be showing the computer an image of a woman working in an office and then labelling this as woman office worker.
Over time and with many images, the computer will have learned to recognise similar images and be able to associate these images with women working in an office. Over time, and with the addition of algorithms, the computer can make predictions for things such as job candidate screening (replacing humans to screen CVs), issuing insurances or making loan approvals.
The financial industry is already advanced in using AI systems. For example, it uses them to assess credit risk before issuing credit cards or awarding small loans. The task is to filter clients in order to avoid having any that are likely to fail to make payments. Using data of declined clients and associating them with a set of rules could easily lead to biases. One such rule could be: “If the client is a single woman, then do not accept her application.”
This is not all. The careers platform LinkedIn, for instance, had an issue where highly-paid jobs were not displayed as frequently for searches by women as they were for men because of the way its algorithms were written. The initial users of the site’s job search function were predominantly male for these high-paying jobs so it just ended up proposing these jobs to men – thereby simply reinforcing the bias against women. One study found a similar issue with Google.
Another study shows how images that are used to train image-recognition software amplify gender biases. Two large image collections used for research purposes – including one supported by Microsoft and Facebook – were found to display predictable gender biases in photos of everyday scenes such as sport and cooking. Images of shopping and washing were linked to women, while coaching and shooting were tied to men. If a photo set generally associates women with housework, software trained by studying those photos and their labels create an even stronger association with it.
Testing for bias
The training of machines using data remains unproblematic as long as it does not lead to discriminatory predictive actions. Yet, as the use of data is extended more and more to the replacement of human decisions, this becomes problematic. Therefore the underlying biases that these black boxes make need to be understood.
One way to test for biases is by stress-testing the system. This has been demonstrated by computer scientist Anupam Datta who designed a programme to test whether AI showed bias in hiring new employees.
Machine learning can be used to pre-select candidates based on various criteria such as skills and education. This produces a score which indicates how fit the candidate is for the job. In a candidate selection program for removal companies, Datta’s program randomly changed the gender and the weight they said they could lift in their application and if there was no change in the number of women that were pre-selected for interviews, then it is not the applicant’s sex that determined the hiring process.
As this example shows, it is possible to remove biases. But this takes effort and money to put in place and so isn’t guaranteed to happen. In fact, it is more likely that we will see an increase in biases in the short term, as AI amplifies them.
In the long run, if artificial intelligence will lead to humans being replaced by machines in some situations, women’s higher levels of emotional intelligence will become all the more valuable. There will be a greater need for roles that understand human behaviour in ways that machines struggle to. These roles will require understanding of social contexts, empathy and compassion – and it is here that people with higher levels of emotional intelligence will excel. So, although biases are likely to increase in the short run, in the long run gender equality does stand a chance.
Don't miss any update on this topic
Create a free account and access your personalized content collection with our latest publications and analyses.
License and Republishing
World Economic Forum articles may be republished in accordance with the Creative Commons Attribution-NonCommercial-NoDerivatives 4.0 International Public License, and in accordance with our Terms of Use.
The views expressed in this article are those of the author alone and not the World Economic Forum.
Stay up to date:
Artificial Intelligence
The Agenda Weekly
A weekly update of the most important issues driving the global agenda
You can unsubscribe at any time using the link in our emails. For more details, review our privacy policy.
More on Emerging TechnologiesSee all
Filipe Beato and Jamie Saunders
November 21, 2024