What does artificial intelligence mean for the future of science?
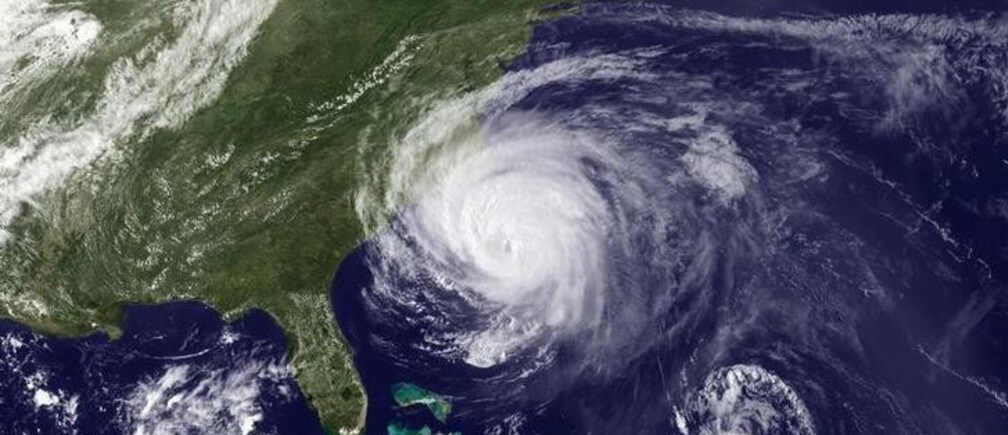
A machine can predict but can it understand?
Image: REUTERS/NOAA/Handout
Stay up to date:
Emerging Technologies
Much to the chagrin of summer party planners, weather is a notoriously chaotic system. Small changes in precipitation, temperature, humidity, wind speed or direction, etc. can balloon into an entirely new set of conditions within a few days. That’s why weather forecasts become unreliable more than about seven days into the future — and why picnics need backup plans.
But what if we could understand a chaotic system well enough to predict how it would behave far into the future?
In January this year, scientists did just that. They used machine learning to accurately predict the outcome of a chaotic system over a much longer duration than had been thought possible. And the machine did that just by observing the system’s dynamics, without any knowledge of the underlying equations.
Awe, fear and excitement.
We’ve recently become accustomed to artificial intelligence’s (AI) dazzling displays of ability. Last year, a program called AlphaZero taught itself the rules of chess from scratch in about a day, and then went on to beat the world’s best chess-playing programs. It also taught itself the game of Go from scratch and bettered the previous silicon champion, the algorithm AlphaGo Zero, which had itself mastered the game by trial and error after having been fed the rules.
Many of these algorithms begin with a blank slate of blissful ignorance, and rapidly build up their “knowledge” by observing a process or playing against themselves, improving at every step, thousands of steps each second. Their abilities have variously inspired feelings of awe, fear and excitement, and we often hear these days about what havoc they may wreak upon humanity.
My concern here is simpler: I want to understand what AI means for the future of “understanding” in science.
If you predict it perfectly, do you understand it?
Most scientists would probably agree that prediction and understanding are not the same thing. The reason lies in the origin myth of physics — and arguably, that of modern science as a whole.
For more than a millennium, the story goes, people used methods handed down by the Greco-Roman mathematician Ptolemy to predict how the planets moved across the sky.
Ptolemy didn’t know anything about the theory of gravity or even that the sun was at the centre of the solar system. His methods involved arcane computations using circles within circles within circles. While they predicted planetary motion rather well, there was no understanding of why these methods worked, and why planets ought to follow such complicated rules.
Then came Copernicus, Galileo, Kepler and Newton.
Newton discovered the fundamental differential equations that govern the motion of every planet. The same differential equations could be used to describe every planet in the solar system.
This was clearly good, because now we understood why planets move.
Solving differential equations turned out to be a more efficient way to predict planetary motion compared to Ptolemy’s algorithm. Perhaps more importantly, though, our trust in this method allowed us to discover new unseen planets based on a unifying principle — the Law of Universal Gravitation — that works on rockets and falling apples and moons and galaxies.
This basic template — finding a set of equations that describe a unifying principle — has been used successfully in physics again and again. This is how we figured out the Standard Model, the culmination of half a century of particle physics, which accurately describes the underlying structure of every atom, nucleus or particle. It is how we are trying to understand high-temperature superconductivity, dark matter and quantum computers. (The unreasonable effectiveness of this method has inspired questions about why the universe seems to be so delightfully amenable to a mathematical description.)
In all of science, arguably, the notion of understanding something always refers back to this template: If you can boil a complicated phenomenon down to a simple set of principles, then you have understood it.
Stubborn exceptions.
However there are annoying exceptions that spoil this beautiful narrative. Turbulence — one of the reasons why weather prediction is difficult — is a notable example from physics. The vast majority of problems from biology, with their intricate structures within structures, also stubbornly refuse to give up simple unifying principles.
While there is no doubt that atoms and chemistry, and therefore simple principles, underlie these systems, describing them using universally valid equations appears to be a rather inefficient way to generate useful predictions.
In the meantime, it is becoming evident that these problems will easily yield to machine-learning methods.
AI might help identify new drugs to treat antibiotic resistant bacterial like Klebsiella, which causes about 10 per cent of all hospital-acquired infections in the United State.
Just as the ancient Greeks sought answers from the mystical Oracle of Delphi, we may soon have to seek answers to many of science’s most difficult questions by appealing to AI oracles.
Such AI oracles are already guiding self-driving cars and stock market investments, and will soon predict which drugs will be effective against a bacterium — and what the weather will look like two weeks ahead.
They will make these predictions much better than we ever could have, and they will do it without recourse to our mathematical models and equations.
It is not inconceivable that, armed with data from billions of collisions at the Large Hadron Collider, they might do a better job at predicting the outcome of a particle physics experiment than even physicists’ beloved Standard Model!
As with the inscrutable utterances of the priestesses of Delphi, our AI oracles are also unlikely to be able to explain why they predict what they do. Their outputs will be based on many microseconds of what might be called “experience.” They resemble that caricature of an uneducated farmer who can perfectly predict which way the weather will turn, based on experience and a gut feeling.
Science without understanding?
The implications of machine intelligence, for the process of doing science and for the philosophy of science, could be immense.
For example, in the face of increasingly flawless predictions, albeit obtained by methods that no human can understand, can we continue to deny that machines have better knowledge?
If prediction is in fact the primary goal of science, how should we modify the scientific method, the algorithm that for centuries has allowed us to identify errors and correct them?
If we give up on understanding, is there a point to pursuing scientific knowledge as we know it?
I don’t have the answers. But unless we can articulate why science is about more than the ability to make good predictions, scientists might also soon find that a “trained AI could do their job.”
Accept our marketing cookies to access this content.
These cookies are currently disabled in your browser.
Don't miss any update on this topic
Create a free account and access your personalized content collection with our latest publications and analyses.
License and Republishing
World Economic Forum articles may be republished in accordance with the Creative Commons Attribution-NonCommercial-NoDerivatives 4.0 International Public License, and in accordance with our Terms of Use.
The views expressed in this article are those of the author alone and not the World Economic Forum.
Forum Stories newsletter
Bringing you weekly curated insights and analysis on the global issues that matter.
More on Emerging TechnologiesSee all
Robert Falck
June 19, 2025
Marco Túlio Moraes
June 18, 2025
Sheikh Tanjeb Islam
June 17, 2025
Stacie Calder
June 16, 2025
Kaushal Rathi and Mandanna Appanderanda Nanaiah
June 16, 2025
Clas Neumann
June 16, 2025