Bias in AI is a real problem. Here’s what we should do about it
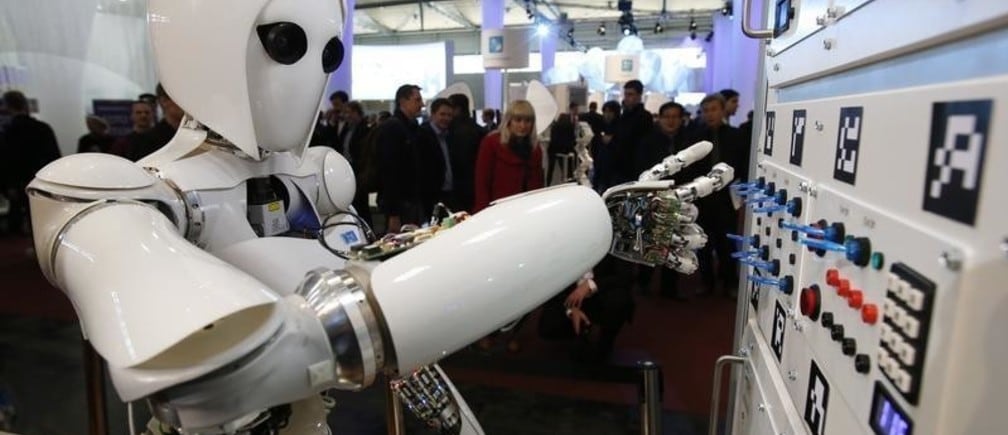
Are we programming AI with our own prejudices? Image: REUTERS/Fabrizio Bensch
Hardly a day goes by without a new story about the power of AI and how it will change our world. However, there are also dangers alongside the possibilities. While we should be rightly excited by the idea of AI revolutionizing eye disease and cancer diagnostics, or the potential transport revolution promised by autonomous vehicles, we should not ignore the risks - and one of the most pressing of these is bias.
Unlike killer time-traveling robots, AI bias is unlikely to be the stuff of the next Hollywood blockbuster - and yet it is a potentially huge problem.
What is algorithm bias?
How can AI be biased?
Although it’s comforting to imagine AI algorithms as completely emotionless and neutral, that is simply not true. AI programmes are made up of algorithms that follow rules. They need to be taught those rules, and this occurs by feeding the algorithms with data, which the algorithms then use to infer hidden patterns and irregularities. If the training data is inaccurately collected, an error or unjust rule can become part of the algorithm - which can lead to biased outcomes.
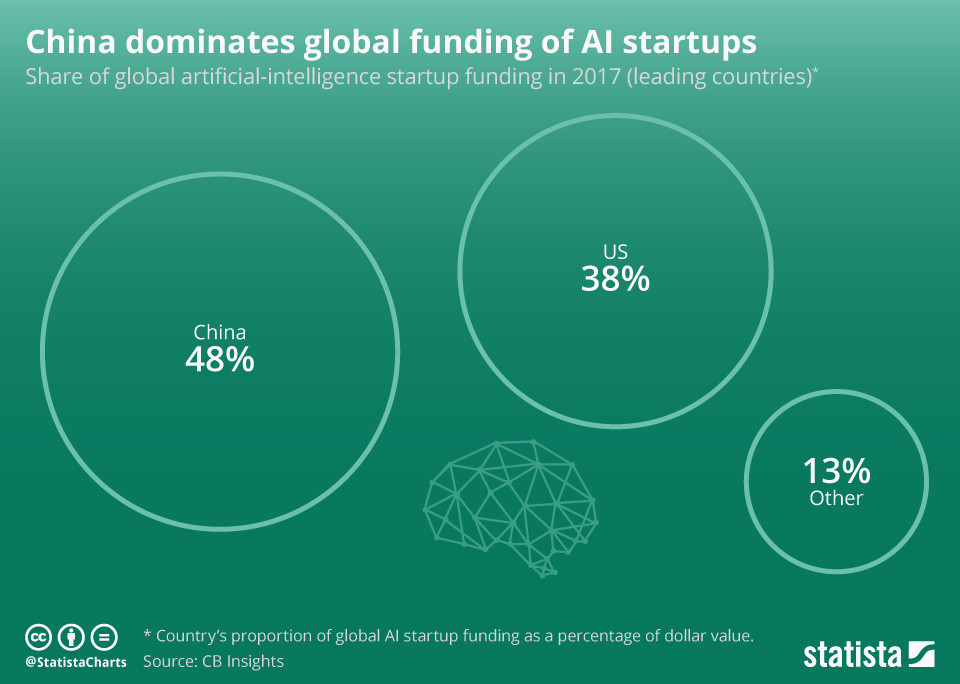
Some day-to-day examples might be worse performing facial recognition software on non-white people, or speech recognition software that doesn’t recognize women’s voices as well as men’s. Or consider the even more worrying claims of racial discrimination in the AI used by credit agencies and parole boards.
The algorithm used by a credit agency might be developed using data from pre-existing credit ratings or based on a particular group’s loan repayment records. Alternatively, it might use data that is widely available on the internet - for example, someone’s social media behaviour or generalized characteristics about the neighborhood in which they live. If even a few of our data sources were biased, if they contained information on sex, race, colour or ethnicity, or we collected data that didn’t equally represent all the stakeholders, we could unwittingly build bias into our AI.
If we feed our AI with data showing the majority of high-level positions are filled by men, all of a sudden the AI knows the company is looking to hire a man, even when that isn’t a criteria. Training algorithms with poor datasets can lead to conclusions such as that women are poor candidates for C-suite roles, or that a minority from a poor ZIP code is more likely to commit a crime.
As we know from basic statistics, even if there is a correlation between two characteristics, that doesn’t mean that one causes the other. These conclusions may not be valid and individuals should not be disadvantaged as a result. Rather, this implies that the algorithm was trained using poorly collected data and should be corrected.
Fortunately, there are some key steps we can take to prevent these biases from forming in our AI.
1. Awareness of bias
Acknowledging that AI can be biased is the vital first step. The view that AI doesn’t have biases because robots aren’t emotional prevents us from taking the necessary steps to tackle bias. Ignoring our own responsibility and ability to take action has the same effect.
2. Motivation
Awareness will provide some motivation for change but it isn’t enough for everyone. For-profit companies creating a product for consumers have a financial incentive to avoid bias and create inclusive products; if company X’s latest smartphone doesn’t have accurate speech recognition, for example, then the dissatisfied customer will go to a competitor. Even then, there can be a cost-benefit analysis that leads to discriminating against some users.
For groups where these financial motives are absent, we need to provide outside pressure to create a different source of motivation. The impact of a biased algorithm in a government agency could unfairly impact the lives of millions of citizens.
We also need clear guidelines on who is responsible in situations where multiple partners deploy an AI. For example, a government programme based on privately developed software that has been repackaged by another party. Who is responsible here? We need to make sure that we don’t have a situation where everyone passes the buck in a never-ending loop.
3. Ensuring we use quality data
All the issues that arise from biased AI algorithms are rooted in the tainted training data. If we can avoid introducing biases in how we collect data and the data we introduce to the algorithms, then we have taken a significant step in avoiding these issues. For example, training speech recognition software on a wide variety of equally represented users and accents can help ensure no minorities are excluded.
If AI is trained on cheap, easily acquired data, then there is a good chance it won’t be vetted to check for biases. The data might have been acquired from a source which wasn’t fully representative. Instead, we need to make sure we base our AI on quality data that is collected in ways which mitigate introducing bias.
4. Transparency
The AI Now initiative believes that if a public agency can’t explain an algorithm or how it reaches its conclusion, then it shouldn’t be used. In situations like this, we can identify why bias and unfair decisions are being reached, give the people the chance to question the outputs and, as a consequence, provide feedback that can be used to address the issues appropriately. It also helps keep those responsible accountable and prevents companies from relinquishing their responsibilities.
While AI is undeniably powerful and has the potential to help our society immeasurably, we can’t pass the buck of our responsibility for equality to the mirage of a supposedly all-knowing AI algorithms. Biases can creep in without intention, but we can still take action to mitigate and prevent them. It will require awareness, motivation, transparency and ensuring we use the right data.
Don't miss any update on this topic
Create a free account and access your personalized content collection with our latest publications and analyses.
License and Republishing
World Economic Forum articles may be republished in accordance with the Creative Commons Attribution-NonCommercial-NoDerivatives 4.0 International Public License, and in accordance with our Terms of Use.
The views expressed in this article are those of the author alone and not the World Economic Forum.
Stay up to date:
Digital Communications
Forum Stories newsletter
Bringing you weekly curated insights and analysis on the global issues that matter.
More on Emerging TechnologiesSee all
Michele Mosca and Donna Dodson
December 20, 2024