This AI thinks like you to solve problems
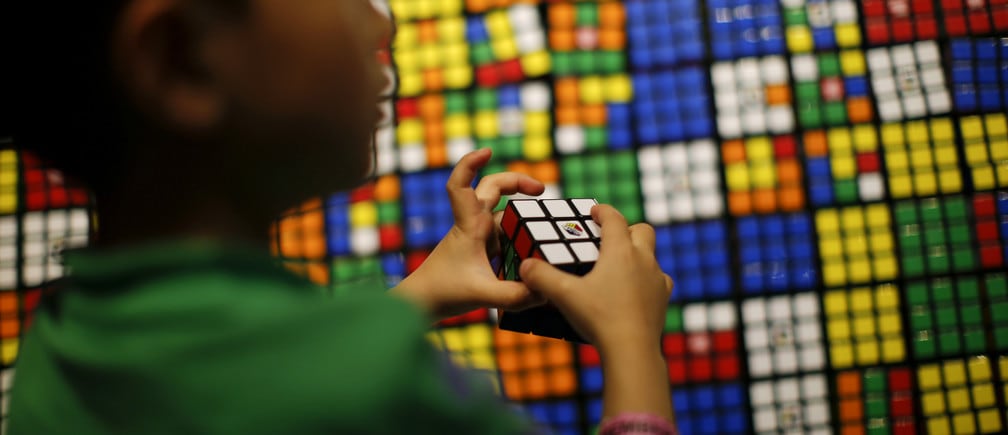
We learn through reason how to interpret the world. So, too, do neural networks. Image: REUTERS/Eduardo Munoz (UNITED STATES - Tags: SOCIETY ANNIVERSARY) - GM1EA4R0B2Z01
A child is presented with a picture of various shapes and is asked to find the big red circle. To come to the answer, she goes through a few steps of reasoning: First, find all the big things; next, find the big things that are red; and finally, pick out the big red thing that’s a circle.
We learn through reason how to interpret the world. So, too, do neural networks. Now a team of researchers from MIT Lincoln Laboratory's Intelligence and Decision Technologies Group has developed a neural network that performs human-like reasoning steps to answer questions about the contents of images. Named the Transparency by Design Network (TbD-net), the model visually renders its thought process as it solves problems, allowing human analysts to interpret its decision-making process. The model performs better than today’s best visual-reasoning neural networks.
Understanding how a neural network comes to its decisions has been a long-standing challenge for artificial intelligence (AI) researchers. As the neural part of their name suggests, neural networks are brain-inspired AI systems intended to replicate the way that humans learn. They consist of input and output layers, and layers in between that transform the input into the correct output. Some deep neural networks have grown so complex that it’s practically impossible to follow this transformation process. That's why they are referred to as "black box” systems, with their exact goings-on inside opaque even to the engineers who build them.
With TbD-net, the developers aim to make these inner workings transparent. Transparency is important because it allows humans to interpret an AI's results.
It is important to know, for example, what exactly a neural network used in self-driving cars thinks the difference is between a pedestrian and stop sign, and at what point along its chain of reasoning does it see that difference. These insights allow researchers to teach the neural network to correct any incorrect assumptions. But the TbD-net developers say the best neural networks today lack an effective mechanism for enabling humans to understand their reasoning process.
"Progress on improving performance in visual reasoning has come at the cost of interpretability,” says Ryan Soklaski, who built TbD-net with fellow researchers Arjun Majumdar, David Mascharka, and Philip Tran.
The Lincoln Laboratory group was able to close the gap between performance and interpretability with TbD-net. One key to their system is a collection of "modules," small neural networks that are specialized to perform specific subtasks. When TbD-net is asked a visual reasoning question about an image, it breaks down the question into subtasks and assigns the appropriate module to fulfill its part. Like workers down an assembly line, each module builds off what the module before it has figured out to eventually produce the final, correct answer. As a whole, TbD-net utilizes one AI technique that interprets human language questions and breaks those sentences into subtasks, followed by multiple computer vision AI techniques that interpret the imagery.
Majumdar says: "Breaking a complex chain of reasoning into a series of smaller subproblems, each of which can be solved independently and composed, is a powerful and intuitive means for reasoning."
Each module's output is depicted visually in what the group calls an "attention mask." The attention mask shows heat-map blobs over objects in the image that the module is identifying as its answer. These visualizations let the human analyst see how a module is interpreting the image.
Take, for example, the following question posed to TbD-net: “In this image, what color is the large metal cube?" To answer the question, the first module locates large objects only, producing an attention mask with those large objects highlighted. The next module takes this output and finds which of those objects identified as large by the previous module are also metal. That module's output is sent to the next module, which identifies which of those large, metal objects is also a cube. At last, this output is sent to a module that can determine the color of objects. TbD-net’s final output is “red,” the correct answer to the question.
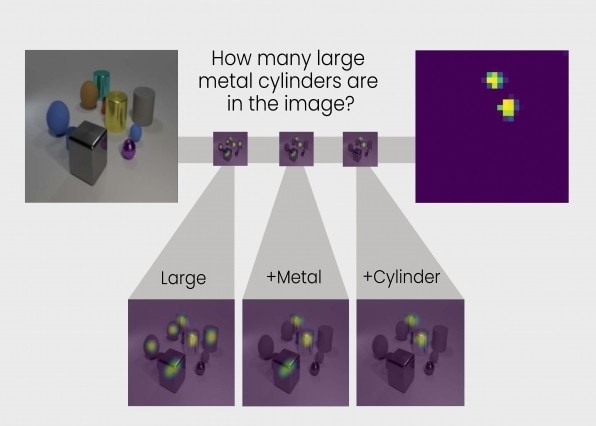
When tested, TbD-net achieved results that surpass the best-performing visual reasoning models. The researchers evaluated the model using a visual question-answering dataset consisting of 70,000 training images and 700,000 questions, along with test and validation sets of 15,000 images and 150,000 questions. The initial model achieved 98.7 percent test accuracy on the dataset, which, according to the researchers, far outperforms other neural module network–based approaches.
Importantly, the researchers were able to then improve these results because of their model's key advantage — transparency. By looking at the attention masks produced by the modules, they could see where things went wrong and refine the model. The end result was a state-of-the-art performance of 99.1 percent accuracy.
"Our model provides straightforward, interpretable outputs at every stage of the visual reasoning process,” Mascharka says.
Interpretability is especially valuable if deep learning algorithms are to be deployed alongside humans to help tackle complex real-world tasks. To build trust in these systems, users will need the ability to inspect the reasoning process so that they can understand why and how a model could make wrong predictions.
Paul Metzger, leader of the Intelligence and Decision Technologies Group, says the research “is part of Lincoln Laboratory’s work toward becoming a world leader in applied machine learning research and artificial intelligence that fosters human-machine collaboration.”
The details of this work are described in the paper, “Transparency by Design: Closing the Gap Between Performance and Interpretability in Visual Reasoning," which was presented at the Conference on Computer Vision and Pattern Recognition (CVPR) this summer.
Don't miss any update on this topic
Create a free account and access your personalized content collection with our latest publications and analyses.
License and Republishing
World Economic Forum articles may be republished in accordance with the Creative Commons Attribution-NonCommercial-NoDerivatives 4.0 International Public License, and in accordance with our Terms of Use.
The views expressed in this article are those of the author alone and not the World Economic Forum.
Stay up to date:
Digital Communications
Forum Stories newsletter
Bringing you weekly curated insights and analysis on the global issues that matter.
More on Emerging TechnologiesSee all
Michele Mosca and Donna Dodson
December 20, 2024