How AI can inspire doctors to be more inventive
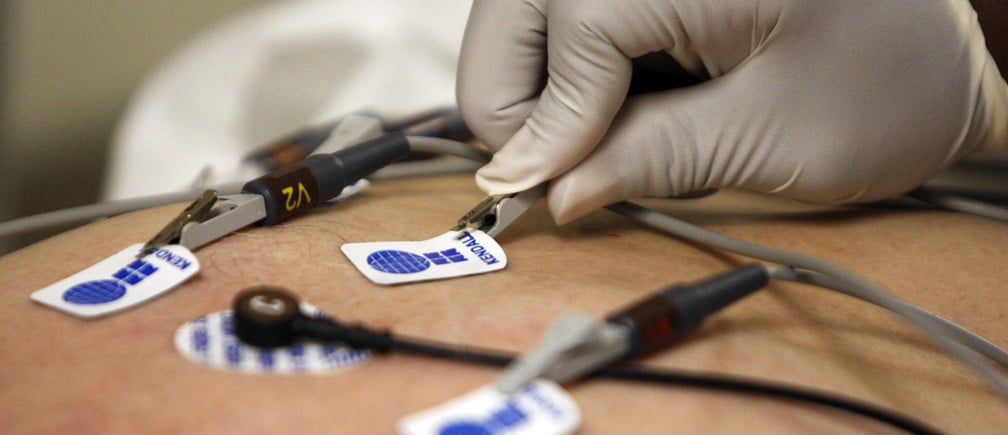
Getting to the heart of the matter: Smart AI could free up doctors Image: REUTERS/Jessica Rinaldi
In 2017, DeepMind’s AlphaGo computer beat the world champion of the strategy board game Go. The computer knew only the rules of the game, which has more possible moves than there are atoms in the visible universe. Some saw it as a watershed - the point at which machines officially became more intelligent than humankind.
Yet, a subtle point (not so widely reported) was that AlphaGo defied long-held conventions for moves. Consequently, the grand masters of the game have admitted to being liberated, and have dared to imagine new moves and strategies which are leading to extraordinary winning streaks.
But what does this have to do with healthcare?
We are currently in the throes of a technological revolution in which machines are being empowered with the ability to learn and to work out solutions for themselves. Machines are beginning to challenge human imagination in a way that may not have been anticipated, and which could, paradoxically, teach humankind to dare to imagine beyond our current constraints and unleash a revolution in creativity. In this way, in the context of healthcare, machine learning is likely to identify new physiological measures that can inform and inspire doctors towards new ways of maintaining health and stabilizing disease.
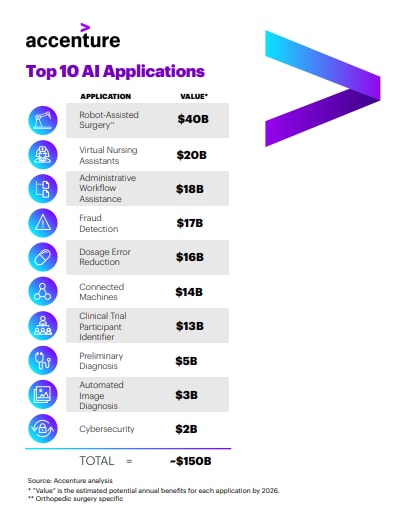
The entirety of clinical science has been shaped solely by an historic ability to measure something. We measure blood pressure only because 150 years ago someone found that they could, without at first understanding the full implications. A decade or so later, doctors showed that individuals with high blood pressures were having more life-threatening health events than those with a lower value, and this in turn encouraged the development of drugs that could lower high blood pressure. Several years later, conclusive evidence arrived that these agents could reduce life-threatening cardiovascular events, including stroke, fatal heart attack and kidney failure. And so a whole field of clinical science and an associated industry of diagnostics, investigation and treatment evolved from this initial enquiry into blood pressure.
The coming revolution will start with conventional measures of health that underpin existing clinical fields, then evolve to use machine learning to identify other signals derived from the use of consumer devices that can be incorporated into the cradle-to-grave pathways of healthy living.
Consider, for example, how the healthcare ecosystem might evolve around ubiquitous availability of the electrocardiogram (ECG), or an equivalent indicator of heart beating and rhythm.
A major clinical challenge for conventional clinical science is a heart rhythm disturbance called atrial fibrillation, which is increasing in prevalence and is often present without symptoms until the point of causing a stroke. Atrial fibrillation may be intermittent, but it is easily diagnosed from the ECG and easily treated to prevent stroke. Incidentally, the recently launched Apple Watch 4 incorporates an ECG, and although yet to prove its value, it nonetheless demonstrates how consumer diagnostics might have an impact on population health.
Once such diagnostics are widely available, novel population care pathways will evolve. Another, different type of heart rhythm disturbance can cause sudden cardiac death and is detectable by ECG or an equivalent indicator of heart beating. Someone in the state of cardiac arrest needs to receive CPR within five minutes to avoid brain damage. An ecosystem that uses smart consumer devices to alert and recruit a nearby citizen who has registered their competence to deliver CPR would have a major impact on survival rates.
Beyond this, the long-term monitoring and consolidation of large volumes of ECG with other biosensor and general lifestyle and behavioural data will bring to light as yet unrecognized signals that are relevant to health outcomes. Most importantly, it will enable entirely new fields of sharper, better and more cost effective clinical science to emerge that may supersede blunt measurements such as the likes of blood pressure.
In the face of the inexorable increase in the burden on healthcare systems worldwide, there is a very worrying trend of diminishing numbers of young people aspiring to study medicine. Many cite unnecessarily long and inflexible hours and the failure of healthcare systems to embrace innovation as contributing factors.
Healthcare has become less based on clinical acumen and judgement, and more based on tests and investigations to produce data for protocoled management decisions. The result is an increasing obsession with numbers that has changed both the role of the doctor and the expectations of an increasingly demanding public.
Paradoxically, the solution may lie in even more data, algorithms and protocols, but with these processes taken out of the hands of the healthcare worker and fully automated using smart technologies. This has enormous advantages that include a greater knowledge base and objectivity, indefatigability and the ability to identify and then exploit new and previously unrecognized data signals in patients.
The problem right now is that we are in a transitional phase, in which clinical staff are having to deal with data overload as well as managing increasingly demanding patients. The algorithmic component of care, estimated to be 70% of the workload of a doctor, needs to be taken on by technologies. This would free up the doctor to focus on the 30%, resulting in better, more cost-effective and more enjoyable healthcare delivery.
This will ultimately prompt a global rethink about how healthcare is organized and delivered. Could connected technologies herald a real and sustainable means of enabling effective large-scale disease prevention and management programmes? For the first time, we have connected care tools to give people greater control over their own health and well-being, and to drive changes in attitudes, expectations and behaviour.
Just like the reinvigorated grand masters of Go, let doctors and researchers dare to imagine that the transition to connected self-care, coupled with deep machine learning, can identify better and more useful signals around which to enrich clinical science to improve population health.
Don't miss any update on this topic
Create a free account and access your personalized content collection with our latest publications and analyses.
License and Republishing
World Economic Forum articles may be republished in accordance with the Creative Commons Attribution-NonCommercial-NoDerivatives 4.0 International Public License, and in accordance with our Terms of Use.
The views expressed in this article are those of the author alone and not the World Economic Forum.
Stay up to date:
Healthcare Delivery
Related topics:
The Agenda Weekly
A weekly update of the most important issues driving the global agenda
You can unsubscribe at any time using the link in our emails. For more details, review our privacy policy.
More on Health and Healthcare SystemsSee all
Zili Huang and Kejun Albert Ying
November 1, 2024