Here's the 8 types of Artificial Intelligence, and what you should know about them
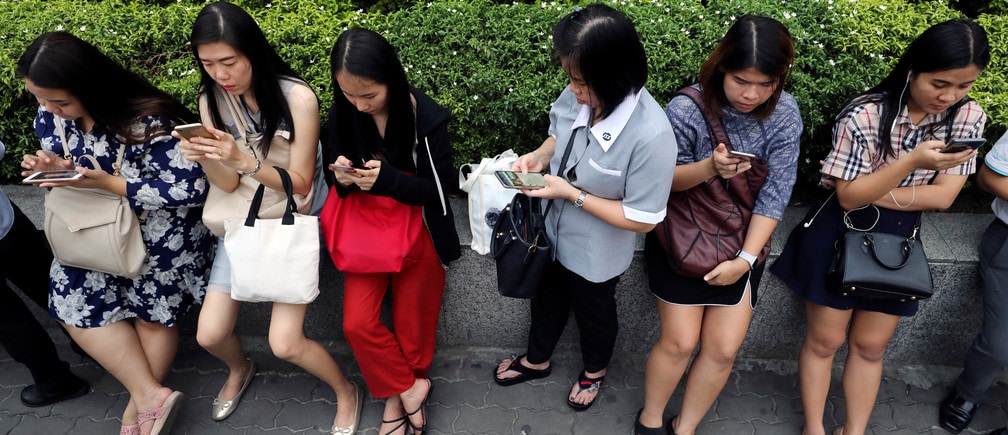
Clarity of purpose can ensure the ethical application of AI technologies, including popular chatbots Image: REUTERS/Jorge Silva
Artificial intelligence (AI) is a broadly-used term, akin to the word manufacturing, which can cover the production of cars, cupcakes or computers. Its use as a blanket term disguises how important it is to be clear about AI’s purpose. Purpose impacts the choice of technology, how it is measured and the ethics of its application.
At its root, AI is based on different meta-level purposes. As Bernard Marr comments in Forbes, there is a need to distinguish between “the ability to replicate or imitate human thought” that has driven much AI to more recent models which “use human reasoning as a model but not an end goal”. As the overall definition of AI can vary, so does its purpose at an applied level.
From my analysis, there are at least eight types of purpose within applied conversational AI. Within each there is a primary intent as suggested in this table:
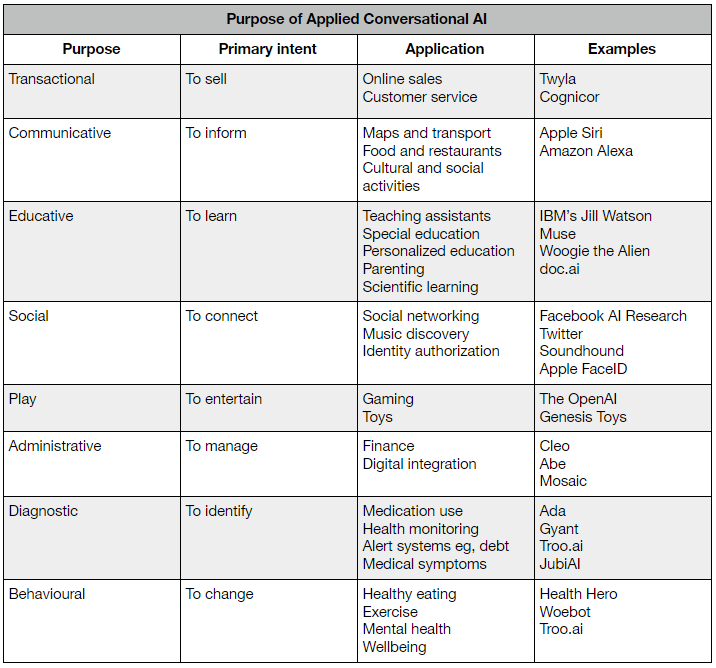
Currently, the most common purpose of conversational AI is transactional. According to a 2017 Statista report, the public views chatbots as primarily for more immediate, personalized and simple interaction with their chosen brands. This may reflect how some companies hide the transactional purpose of their chatbots in interfaces that appear to offer play, diagnosis or information to disguise in-game sales, 2-for-1 offers or travel deals. More broadly, it more likely shows that conversational AI for transactional purposes is easier to implement and monetize.
The Statista report does suggest, however, that chatbots gaining popularity in more complex areas where the purpose is diagnostic or behavioural such as healthcare, telecoms and finance. These conversational AI systems, particularly in high-risk areas like health, are more difficult to develop and monetize; they may be a longer-term game.
Clarity about purpose drives deliberative choices about technology. For example, in determining how a conversational AI system is trained, the extent to which it is self-learning and whether it is necessary or advisable to keep a human in the loop. In this respect, the debate about what is “pure” conversational AI (neural networks and deep learning) versus “simple” AI (rules-based systems) only has meaning in relation to purpose. Whilst hybrid systems are likely to become more of the norm, its current state suggests that simple (more predictable) AI with a human in the loop is most relevant to behavioural and diagnostic purposes, and there is the potential to take more risk where the purpose is playful or social.
Having said that, Microsoft’s chatbot Tay demonstrated the dangers of releasing a self-learning chatbot into a public arena as it learned hate speech within hours. This serves as a helpful reminder that all current conversational AI is based in statistics and not belief systems. For this reason, some recommend keeping a human in the loop as a better technological choice. As Martin Reddy comments: “[K]eeping a human in the loop of initial dialogue generation may actually be a good thing, rather than something we must seek to eradicate (…) Natural language generation solutions must allow for input by a human ‘creative director’ able to control the tone, style and personality of the synthetic character.”
Critically, what these points emphasize, is that a conversational AI system is dynamic. Many of the commercially available chatbot builders could make this clearer. There is a notion that you build and then deploy and then tweak. The reality is that you continuously build based on conversational logs and the metrics that you deem relevant. Those metrics, I would argue, need to be driven by the purpose of the system that you create.
I am troubled by the dominant metrics for digital that appear to have been applied to conversational AI. The mantra of “how many, how often, how long” is not fit for many purposes yet continues to dominate how success is measured. It is time, perhaps, to add a little less sale and a larger dose of evidence to discussions on metrics.
Whilst conversion and retention rates are valid measures of engagement in digital tools, they need to be contextualized in purpose. For example, is a user who engages 10 times a day a positive outcome for a tool focused on wellbeing or is it a failure of any triaging/signposting system? Perhaps, it is time to look at meaningful use as a better indicator of the value of conversational AI.
Recently we worked with Permobil, a Swedish assistive technology manufacturer, to offer power wheelchair customers conversational AI focused on elements of diagnosis and behaviour change, such as a power actuator supported exercise to reduce pressure sores. Whilst most users found the system helpful, there were different patterns of preferred usage with relatively few people wanting to use it daily. Combined with data, such as time spent in the wheelchair and the user’s underlying condition, integration of preferred usage patterns can drive algorithms for user-centric, conversational AI. The more a system is personalized to the user, the better the likely outcomes for sales and customer retention.
In my view, the long-term winners in the conversational AI space will be those who can demonstrate meaningful use and beneficial outcomes that stem directly from the purpose of their systems. Purpose-related outcomes, beyond those applied to transactional conversational AI, are the best driver of successful business models.
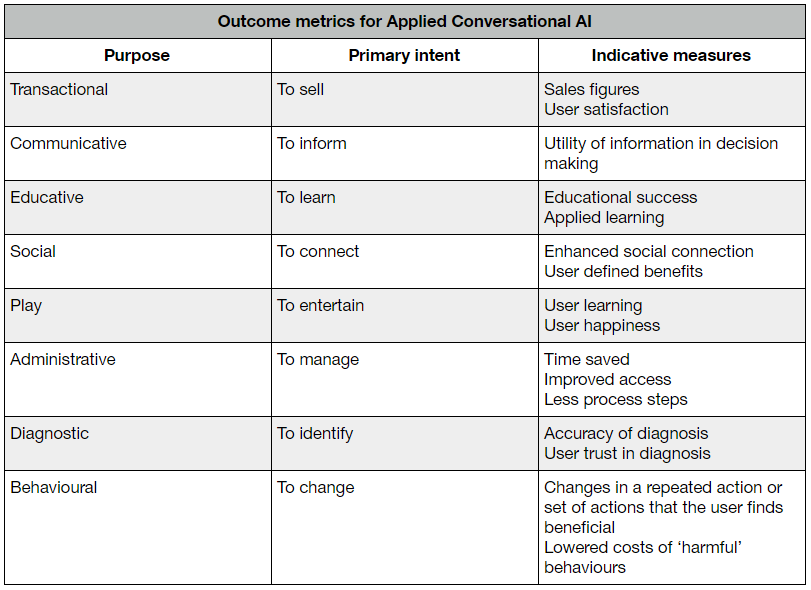
It is easier to track the outcomes for some purposes than others. A Drift report identified 24-hour service, instant responses and answers to simple questions as the essential consumer outcomes for chatbots in the customer services field. These metrics are easier to track than outcomes for conversational AI for diagnostic or behavioural purposes which often require more rigorous scientific approaches.
Commitment to research on the benefits of conversational AI is essential to growing customer confidence and use of these tools, as well as to monetization strategies. Natural Language Understanding has an interesting role to play in outcome analytics. For example, at digital mental health service Big White Wall, it was possible to predict PHQ 9 [an instrument used for screening, diagnosing, monitoring and measuring the severity of depression] scores and GAD 7 [General Anxiety Disorder self-report questionnaire] scores with high confidence levels with just 20 non-continuous words contributed by a user.
By extrapolation, there is every possibility that changes in language (words, tone, rhythm) will become valid and routine measures of outcomes related to purpose. The notion that we may no longer need formal assessment and outcome measures but simply sensitive tools to analyse language is exciting. It also raises a host of ethical issues which underlie the extent to which conversational AI will be trusted and adopted.
We live in an era where technological advance has thrown down the gauntlet to ethical practice, not least in the field of conversational AI. I would argue that clarity of purpose, that is reflected in how engagement and outcomes are measured, goes a long way to ensuring a framework of ethical integrity. In that respect, here are six principles that could help shape that framework:
1. Call a bot a bot
Unless you are entering the Loebner prize, where the judges expect to be tricked into believing a bot is human, there is little justification for presenting a bot as a person.
This is a more nuanced debate than a commitment to countering false news, treating customers with integrity or being able to respond to issues where someone tells a bot they are going to, say, self-harm. It is also about choice. For example, research has shown that some people prefer to talk to a bot for improved customer service or because they can be more open.
2. Be transparent in purpose
Whilst a conversational AI system may have more than one purpose, it is important not to disguise its primary intent. If a conversational AI system appears to have a purpose of information with a hidden intent of selling, it becomes hard for a consumer to both trust the information or be inclined to buy what is on offer.
These are serious considerations for systems like Amazon’s Alexa for which Amazon’s Choice was apparently designed. Amazon states that Choice saves time and effort for items that are highly-rated and well-priced with Prime shipping. However, it is less clear whether this is factual information or whether there are more criteria at play, such as the prioritization of Amazon products.
3. Measure for purpose
As previously suggested, metrics need to be related to purpose and meaningful to customers. Increasingly, those companies and organizations that can demonstrate valid and independent evidence that their conversational AI systems make a difference in relation to their purpose will be favoured over those who bury dubious metrics in marketing speak.
4. Adapt business models
In the digital field, some business models encourage poor ethical behaviour,. Take, for example, advertising revenues that are driven by views where social media giants have deliberately created addictive devices such as infinite scrolling and likes. Aza Raskin, the creator of the infinite scroll, described this as “behavioural cocaine”. He says: “Behind every screen on your phone, there are generally a thousand engineers that have worked on this thing to try to make it maximally addicting.”
The pace of technological change, with monetization often playing catch up, can lead to unintended consequences. For example, Airbnb now has to counter ‘air management’ agencies who inflate prices and disrupt local rental communities. The same trends and temptations are in play with conversational AI. It is our job, as an AI industry, to develop business models that are based on meaningful use and purpose-led outcomes.
5. Act immediately
In the dynamic and unpredictable field that is conversational AI, ethical issues are not always foreseeable. When issues do emerge we need to show leadership and bring alive the principle of “do no harm’”. For example, manufacturer Genesis Toys could not be contacted for comment when German regulators pulled their interactive doll from the market after it was used for remote surveillance. Apparently, Cayla proved more responsible than her makers as when asked “Can I trust you?”, she responded: “I don’t know.” The industry needs to take collaborative responsibility and it is to be hoped that initiatives like the Partnership on AI will take on that role.
6. Self-regulate
A lack of self-regulation impacts an industry and not just a brand. Last year, Facebook researchers proudly presented a bot that was capable of acting deceitfully in a negotiation. In Drift’s ChatBot report, 27% of consumers would be deterred from using a chatbot if it was available only through Facebook.
To conclude, conversational AI has enormous and exciting potential to augment the human experience. However, in my view, the long-term winners in this space will be those who do not prioritize economic gain over ethical practice. The brands that gain greatest popularity will be led by pioneers of purpose who are transparent in practice, who measure meaningful outcomes and who monetize on fair rather than false premises.
Don't miss any update on this topic
Create a free account and access your personalized content collection with our latest publications and analyses.
License and Republishing
World Economic Forum articles may be republished in accordance with the Creative Commons Attribution-NonCommercial-NoDerivatives 4.0 International Public License, and in accordance with our Terms of Use.
The views expressed in this article are those of the author alone and not the World Economic Forum.
Stay up to date:
Emerging Technologies
Related topics:
Forum Stories newsletter
Bringing you weekly curated insights and analysis on the global issues that matter.
More on Emerging TechnologiesSee all
Raj Sharma
January 2, 2025