Using a company’s own words to assess its risks
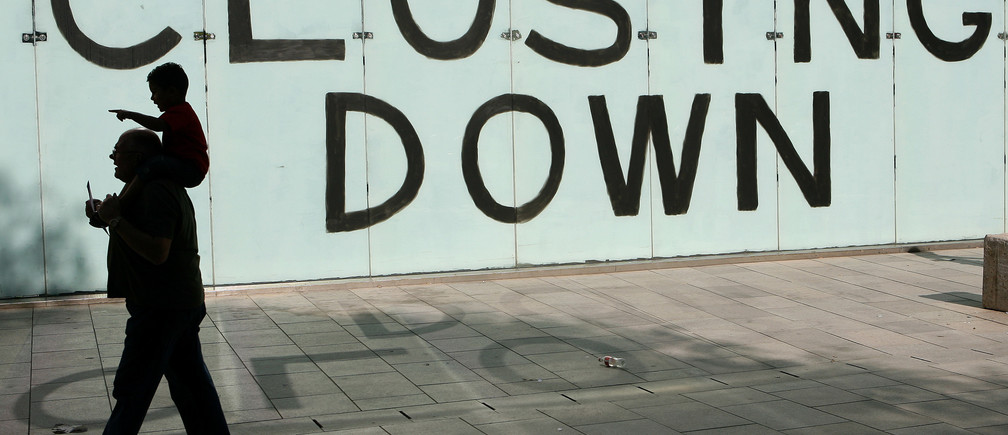
The words companies use in their annual reports tell an interesting story. Image: REUTERS/Phil Noble
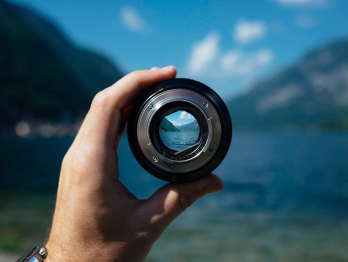
Get involved with our crowdsourced digital platform to deliver impact at scale
Stay up to date:
The Digital Economy
When analysts or academics want to assess the risks that a company faces, they usually look at macroeconomic factors or internal firm metrics such as a declining sales trend to calculate those risks. But research from Wharton doctoral candidate Alejandro Lopez Lira takes a different approach.
He asked this question: What if, instead of letting the outside world tell us what risks a company faces, we let the company tell us itself? After all, a company knows its business best. Lopez Lira used machine learning to read through the annual reports of all U.S. public companies to find out which risks they identified as the most serious ones they face. And the results can be surprising.
His findings are in the paper, “Risk Factors That Matter: Textual Analysis of Risk Disclosures for the Cross-Section of Returns.” His research was supported by Wharton’s Mack Institute for Innovation Management and the Rodney L. White Center for Financial Research.
Lopez Lira recently spoke with Knowledge@Wharton about his paper. An edited transcript of the conversation follows.
Knowledge@Wharton: Tell us how you were able to look through the company’s own words to find out what risks it faces.
Alejandro Lopez Lira: The usual way in which we study the risks firms are facing and how risky it is to invest in them is by checking their fundamentals — for example, sales, dividend yield or market conditions like volatility as well as the macroeconomic environment. This is especially true in academia.
However, publicly held companies file annual reports with the SEC called the 10-K each year. In that annual report, you can see how a company’s business did in the past year as well as a detailed description of each company’s business. And importantly, inside that report, there is also a section that lists all the risks that a company faces. That’s where I decided to look.
Since there are thousands of public companies and years of data, I couldn’t read each statement individually, but luckily there are techniques in machine learning that allowed me to go automatically through the sections listing the risk factors. It looked at all the words and organized them into 25 interpretable risks, without me having to read through any of them. It almost feels like magic. Examples of risks are China or the oil industry.
I ended up organizing them into four systematic risks that affect most firms, since the systematic risk is what investors care about anyways and we can describe a significant portion of the risks with these four.
Knowledge@Wharton: What are the four risks, and can you give us examples for each of them?
Lopez Lira: The first one is technological risk. For example, if a company invests in developing a better screen for its phone product, or in new features for its software product, there’s always a chance that it will not work out as well as you expected. Naturally, firms in the tech sector, such as Oracle or Microsoft, are more affected by this risk.
The second one is production risk. For example, if you’re a hardware company like Samsung, there’s always a chance something goes wrong in the factories or the supply chain and the final product doesn’t end up as planned. As expected, firms with complicated production processes such as Intel or Nvidia are more exposed to this risk.
The third one is international risk. For example, if there’s a recession in Europe, your products may not sell as well as you expected, or if the exchange rate goes sideways you may end up with lower profits than expected. Companies with a global presence such as Apple or Coca-Cola are especially exposed to this risk.
Finally, there’s consumer demand risk, which means that your product may not sell as well as expected because of weak demand, possibly because of economic conditions or because of other competing products. Naturally, retail firms such as Walmart or Starbucks are more exposed to this.
Knowledge@Wharton: You used a text-based approach to identify the biggest risks each company faces. Tell us about some of the challenges of doing that.
Lopez Lira: The first thing I learned is that you can’t just put text into the computer and expect it to understand it — you need a technique that transforms words into numbers that a machine can understand. This is where the machine learning part was crucial, although I will not bore you with the technicalities.
“My approach differs in the sense that it uses the information revealed by the companies … since they probably understand the risks they face better than we do.”
The second challenge is that this is a huge database since I was working with all of the reports done by all public companies since 2006 — so naturally, it was a challenge to fit everything on my small laptop. Luckily, there are some techniques that allowed me to just work with fewer documents at a time.
Finally, languages are complicated, so sometimes just breaking up [compound words] will make some concepts lose their meaning — real estate becomes real and estate — so I had to put those back together. Also, there are many words that don’t really convey information — such as the articles ‘the, a, an,’ etc. — and I had to dispose of them to make the results cleaner.
Knowledge@Wharton: Can you tell us about your findings?
Lopez Lira: Most of the risk in investing in these companies and the movements in the stock price can be explained by how much each company is exposed to these four interpretable risks. And some answers can be illuminating.
For example, one would think the risk Intel faces most is technology, but it turns out that the company, through its language in SEC filings, is telling us the biggest risk comes from international markets, followed by production risk, then demand and finally technology risk. Anyone looking at it from a traditional standpoint would start with technology risk.
Another example comes from Apple. We would naturally think that most of the risk comes from consumer demand, competition with Google and Microsoft, and some production risks. Well, it turns out that their biggest risk, according to them, is related to their international operations, and this of course makes complete sense if you consider that in the first quarter of fiscal year 2019, Apple generated 62% of its revenue outside the U.S.
Knowledge@Wharton: Were you surprised by any of your findings?
Lopez Lira: Definitely. I think we are not used to, at least in academia, paying attention to the rest of the world and the international markets when assessing the risks that companies face. It turns out this is a great mistake, since most of the biggest companies in the stock market — for example Apple, Exxon and Procter & Gamble — operate on a global scale, and a huge part of their profits are derived from international operations.
“Using text analysis combined with machine learning is relatively new for the finance academic literature.”
Knowledge@Wharton: What are some practical applications of your research? For example, would it make Wall Street analysts do a better job of assessing risks in companies they follow?
Lopez Lira: It certainly would make their work easier. You can quickly apply this technique and assess in a second what are the risks each firm is facing, and relate this to the return you expect to get when investing in them.
Knowledge@Wharton: What makes your research different from prior work in this area?
Lopez Lira: The usual approaches for assessing the risk of investing in a company are statistical or involve economic theory. My approach differs in the sense that it uses the information revealed by the companies and gives them the credit they deserve, since they probably understand the risks they face better than we do.
Using text analysis combined with machine learning is relatively new for the finance academic literature, despite the fact that it has been used extensively in the industry. One big exception is a working paper by Ryan D. Israelsen with a similar approach, trying to use text analysis to understand the traditional risk factors in finance. For example, it is well known that firms with a high book-to-market ratio earn higher returns, and they try to understand if this in any way relates to the risks that firms disclose.
I do feel that using new sources of data and techniques such as machine learning has a huge potential in improving our understanding of markets, and the area is substantially under-explored.
Knowledge@Wharton: How will you follow up this research?
Lopez Lira: I want to explore how the risks that each firm discloses are useful to predict the aggregate risks that the economy faces. Hopefully, this will improve our understanding of what causes economic crises and how we can respond and prevent them.
Don't miss any update on this topic
Create a free account and access your personalized content collection with our latest publications and analyses.
License and Republishing
World Economic Forum articles may be republished in accordance with the Creative Commons Attribution-NonCommercial-NoDerivatives 4.0 International Public License, and in accordance with our Terms of Use.
The views expressed in this article are those of the author alone and not the World Economic Forum.
Related topics:
The Agenda Weekly
A weekly update of the most important issues driving the global agenda
You can unsubscribe at any time using the link in our emails. For more details, review our privacy policy.
More on Jobs and the Future of WorkSee all
David Elliott
July 18, 2024
Peter Brown, Kathy Parker and Harriet Newlyn
July 15, 2024
Don Howard and George Zuo
July 9, 2024
James Fell and Linda Lacina
July 3, 2024
Sarah Hewitt
June 25, 2024
Patrick Tsang
June 25, 2024