AI-assisted recruitment is biased. Here’s how to make it more fair
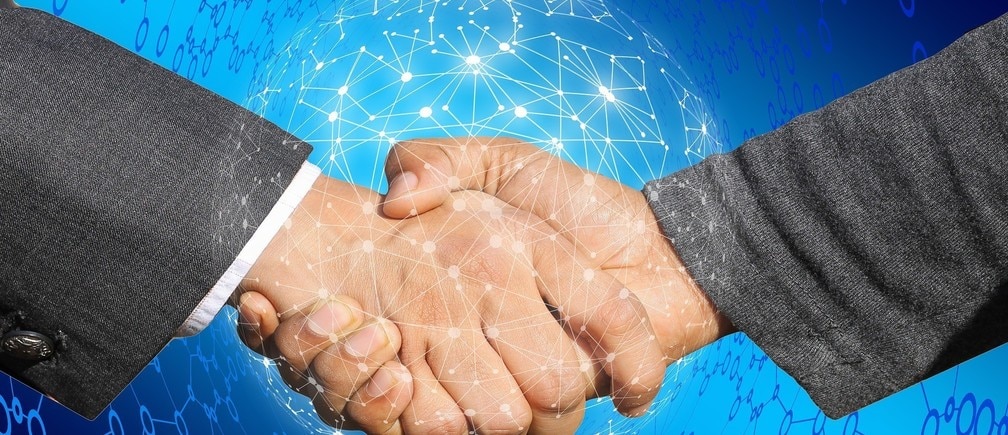
Before you speak to an actual person, your job application could have been screened by multiple algorithms. Image: Maxpixel
Chances are that you have sent hundreds or even thousands of resumes and cover letters over the years to potential employers. This observation is supported by the fact that according to Bureau of Labor Statistics, wage and salary workers in the US have been with their current employer for an average of 4.2 years. Younger workers, however - those aged between 25 and 34 - have on average been with their current employer for just 2.8 years, which suggests they are part of an increasingly transient workforce that is more accustomed to applying for jobs.
This pattern can also be observed in other countries - with a few notable exceptions, such as Japan. As a result, many millennials from around the world are growing increasingly accustomed to this new career model of sending out a near-constant barrage of cover letters and CVs.
Eventually, these applications may lead to you accepting a job offer that determines your quality of life – your income, the time you can spend with friends and family, and the neighbourhood you live in.
In some cases, however, the chances of getting the job for which you have applied for are systematically biased. For example, it has been shown that in the US labour market, African-American names are systematically discriminated against, while white names receive more callbacks for interviews. However, we observe bias not only because of human error, but also because the algorithms increasingly used by recruiters are not neutral; rather, they reproduce the same human errors they are supposed to eliminate. For example, the algorithm that Amazon employed between 2014 and 2017 to screen job applicants reportedly penalised words such as ‘women’ or the names of women’s colleges on applicants’ CVs. Similarly, researchers from Northeastern University, the University of California and Upturn, a public-interest advocacy group, have demonstrated that Facebook’s housing and employment ads delivery follows gender and race stereotypes.
What can you do about it?
Given these biases, what steps can you take to maximise the chances that your CV and cover letter will land you an interview?
Today, recruiters in large companies such as Target, Hilton, Cisco, PepsiCo and Amazon use predictive hiring tools to both reduce the time and cost - and to hypothetically increase the quality and tenure - of each new hire. Understanding at which points algorithms come into play in the hiring process can help identify the origins of bias.
Typically, hiring is not a single decision, but a process involving many small decisions that culminate in a job offer. The aim of the first step - known as sourcing - is to generate a strong set of applicants (see figure 1). This can be done via advertisements, active headhunting or attractive job descriptions. Usually, artificial intelligence (AI) is used to optimise the display of job ads as well as their wording, as done by companies who provide ‘augmented writing’, such as Textio.
The second step, screening, is crucial as this is where algorithmic bias can strongly influence whether your application is rejected. Screening uses algorithms that systematically decipher your cover letter and CV and save this information in the company’s HR database. This information could include your years of experience, the languages you speak, the university degrees you obtained and the countries in which you have you worked. Algorithms are then used to narrow down the selection of candidates automatically – not in an affirmative way, but by rejecting those who do not fit. The company CVViZ, for example, employs machine learning algorithms to screen resumes for keywords in context and to create relative rankings between the different candidates.
If you have made it through the screening process, you may be invited to an interview that might also use different algorithms to support the employer’s final selection decision. HireVue, a US-based company, assesses candidates based on the keywords, facial expressions and tones they use in video interviews. After a video interview you may get a face-to-face interview, after which you are rewarded with an offer.
The use of machine learning algorithms in each of these steps can lead us to question the fairness of an AI-driven recruitment system. As in the case of Facebook, mentioned above, bias may be present in how job advertisements target potential employees. In other cases, web crawlers try to find matching candidates to job descriptions by scanning information from publicly available online sources - and while in this case one might argue the unfairness is limited because it doesn’t prevent you from applying, this screening process may already display strong bias that is difficult to overcome.
Algorithms are often trained to read specific formats of CVs and resumes, which could mean your CV is not evaluated properly. For example, in Japan there is a common CV template (Rirekisho) used by all job applicants. In China, applicants list their work experience in reverse chronology. Other cultural differences exist between American CVs and European CVs; the former is usually one page long with no photo, while the latter can be between two to three pages, headlined with a photo.
If your CV has been successfully parsed - that is, translated into machine-readable data - another algorithm will rank your application vis-à-vis other applications based on the data in your CV and your cover letter. Each factor, such as your years of experience, languages, software skills and the set of words you use, to name but a few success metrics, will be weighted according to what is estimated to have successfully worked in the past. Past hiring decisions are used to train the algorithm to evaluate who is most likely to be the ‘right’ applicant. Often this approach inherently replicates the same biases that were present before the arrival of AI recruiting tools. If the gender distribution of the training data was strongly imbalanced, this may be replicated by an algorithm even if gender is not included in the information provided in the application documents. For example - as in Amazon’s case - strong gender imbalances could correlate with the type of study undertaken. These training data biases might also arise due to bad data quality or very small, non-diverse data sets, which may be the case for companies that do not operate globally and are searching for niche candidates.
Similarly, the evaluation of video interviews conducted prior to any in-person interaction may replicate biases that rely on training data if it has not been thoroughly vetted against categories such as gender, age or religion.
Recommendations
There are several steps both job applicants and employers can take to maximise the chance that the right application will be read by a human being making the ultimate hiring call.
As an applicant, you should:
1) Make sure your CV is formatted according to local norms. Evaluate which length, layout, photo and format are most appropriate. Avoid graphics and fancy fonts that may not be readable by the algorithm.
2) Elaborate on your work experience and adapt your language to that of the job description.
3) Make sure to include key information on your CV – what is not on your CV cannot be evaluated. For example, mention the month and year for each position you held instead of only the year.
4) Optimise your online brand by using the appropriate jargon. Use language that speaks to the job family you are interested in. For example, IT jobs have different titles such as ‘full stack developer’ that are often used in connection with programming languages such as C++ or PHP.
As an employer using machine learning algorithms in the hiring process, ensuring fairness is key. The following concepts, taken from recent research carried out at Delft University of Technology, may provide a guide:
Justification: Does it make sense for an organization of a certain size with specific hiring needs to employ AI hiring tools, given the data requirements and the need for bias remediation?
Explanation: Does the AI tool explain its decisions and are those explanations made available to the recruiter and the applicant? If algorithmic information is proprietary, are counterfactual explanations taken into consideration?
Anticipation: Are mechanisms in place to report biased decisions and what are the recourse mechanisms in place?
Reflexiveness: Is the organization aware of its changing values and its reflection in the data it uses? How is data collected and which limitations become evident?
Inclusion: Do you think about diversity in your team and in the evaluation results?
Auditability: Is the training data publicly available or verified by a third party?
Don't miss any update on this topic
Create a free account and access your personalized content collection with our latest publications and analyses.
License and Republishing
World Economic Forum articles may be republished in accordance with the Creative Commons Attribution-NonCommercial-NoDerivatives 4.0 International Public License, and in accordance with our Terms of Use.
The views expressed in this article are those of the author alone and not the World Economic Forum.
Stay up to date:
The Digital Economy
Related topics:
Forum Stories newsletter
Bringing you weekly curated insights and analysis on the global issues that matter.
More on Jobs and the Future of WorkSee all
Karin Kimbrough
January 21, 2025