We need to measure innovation better. Here's how
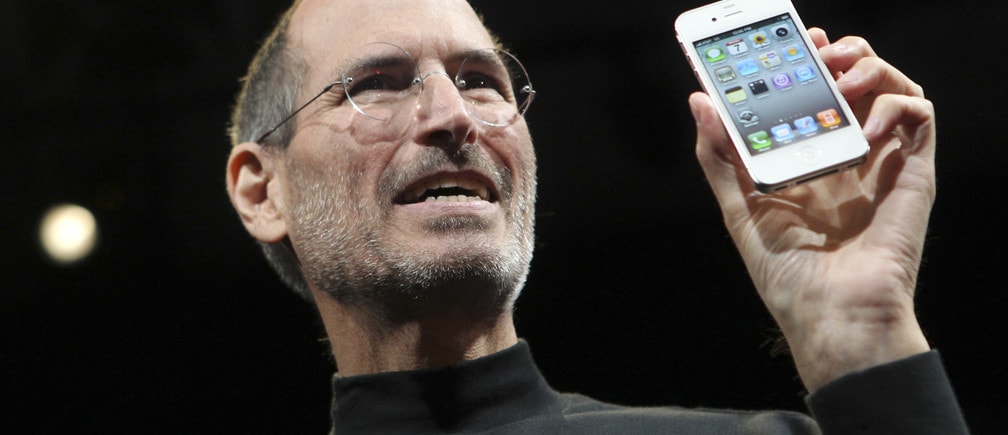
The iPhone wasn't all Apple - it depended on innovations dreamed up elsewhere, such as GPS and the internet Image: REUTERS/Robert Galbraith
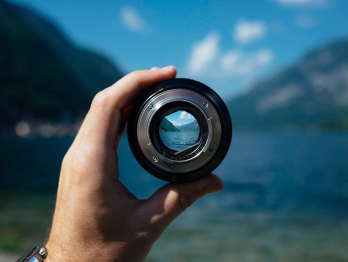
Get involved with our crowdsourced digital platform to deliver impact at scale
Stay up to date:
Innovation
Innovation drives productivity, and productivity is what drives jobs growth and the wealth that pays for our health, education, defence and social security systems. In short, innovation really matters. Governments, businesses and academics obsess over it. We need to know what works, what doesn’t and why - and that means turning to measurements and metrics. But can we really measure innovation today? Are we doing it right, or are our metrics more suited to Industry 2.0 and 3.0, rather than 4.0?
In the era of Industry 4.0, all innovation is combinatorial. Leaders from England’s state-run National Health Service to former Google CEO Eric Schmidt recognise this fact. Almost no innovations can be attributed to a single source.
For example, the iPhone may have been the work of Steve Jobs, Jony Ive and their teams of brilliant engineers and designers, but it was also the product of US government-led innovations such as GPS and, of course, the internet. It would be ludicrous to attribute such innovations as the iPhone to the state alone - but at the same time, there are few truly original ideas. Most successful innovations hinge on the execution rather than the idea itself, which many others may be pursuing simultaneously. The aesthetically similar LG Prada came out shortly before the iPhone, and there were plenty of touchscreen PDA-like phones before the iPhone, but none had the iPhone’s industry-defining impact.
However, the complexity of measuring such innovations should not deter us from trying.
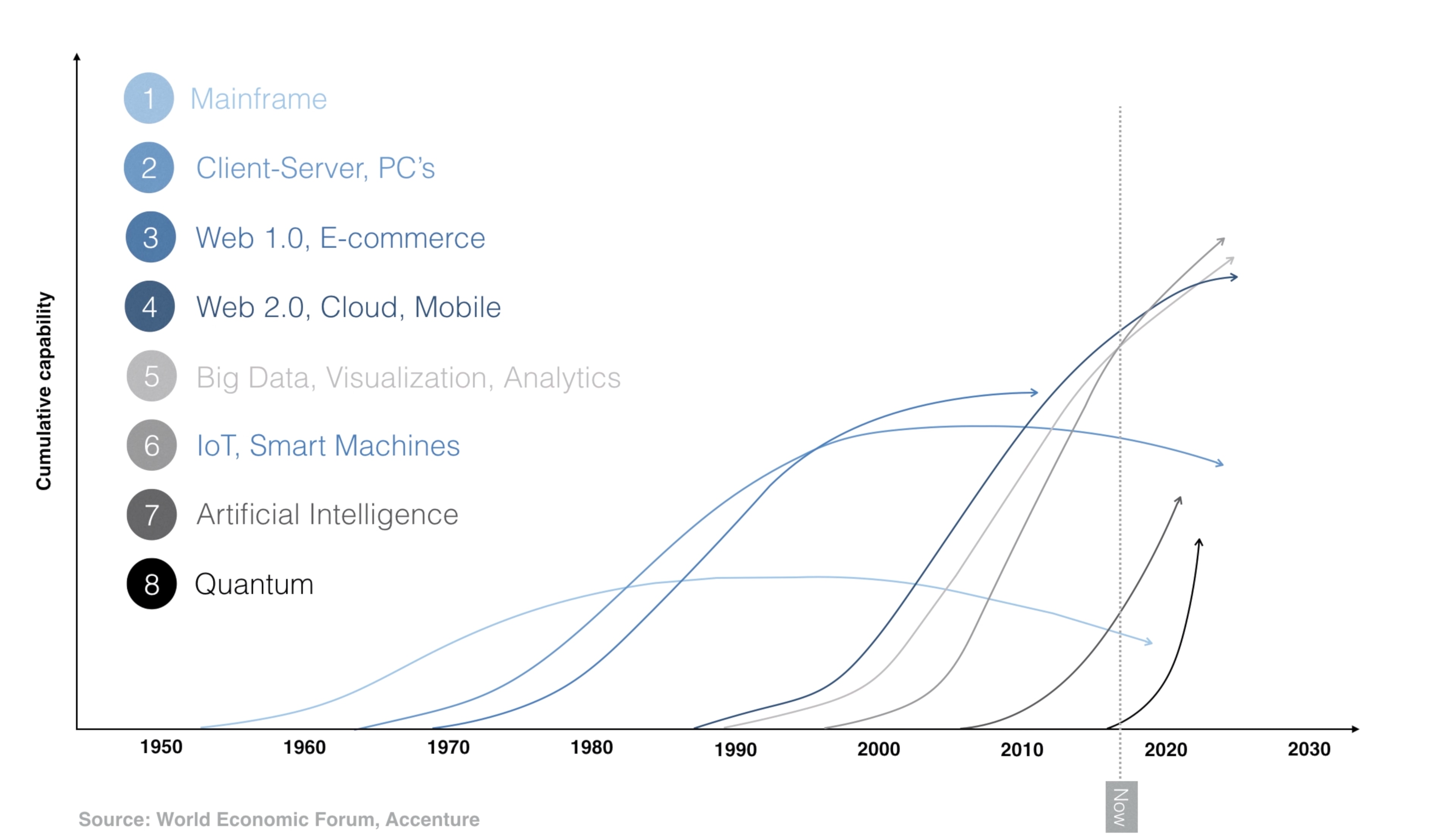
Measuring innovation matters…
Good metrics can direct better policies. The OECD’s Programme for International Student Assessment (PISA), which measures school pupils’ performance in mathematics, science and reading, has improved policy debates and led to improved outcomes in some countries. It has driven Singapore’s extraordinary educational success. Statistics on the causes of mortality, meanwhile, have been instrumental in shifting health resources towards early intervention, which has improved life expectancies.
But measuring innovation is troublesome.
…but it’s hard
Indicators have to account for many different forms of innovation, with widely differing motivations, processes of development and consequences. In the past it was possible to identify innovation within particular organizations, teams or individuals; nowadays innovation is more often networked among multiple contributors, which complicates its measurement. Collecting data on innovation is hampered by the desire to ensure indicators are simple, easily accessible, comparable across nations, and cheap to acquire and compute. And these requirements do not reflect the complex and often messy realities of innovation, let alone capture whether the innovation has negative consequences, such as putting CFCs in refrigerators.
The move over the past 60 years from products to services to an increasingly experiential economy has changed the nature of research and development (R&D). Traditional measures of innovation, such as R&D investment and patents, were fine when innovation mostly occurred in large manufacturing firms, but are of limited value when much of the action lies in services, business models, and entrepreneurial start-ups. Much innovation does not rely on traditional R&D investment and processes, and many innovations are not protected by formal intellectual property rights, but by the speed of changes and secrecy around them - and this makes them difficult to measure.
A great deal of expertise has been developed around innovation surveys that ask firms whether they innovate, and in what forms. The EU’s Community Innovation Survey (CIS), for example, has coordinated national statistical agencies to collect extensive data on the innovativeness of EU regions and sectors. But self-reported innovations can be subjective and difficult to calibrate. We know of world-leading innovations in infrastructure and resources industries that are not accounted for; conversely, a firm that was recorded as innovative in a government survey turned out to be a hairdresser using a new brand of hair colouring.
Other judgement calls are needed: are innovations new to the world, new to the sector or region, or just new to the firm? Is adapting an existing product or service to a new market an innovation? While these surveys can tell us about the ITALnumbers and ITALtypes of firms that claim to be innovative, they don’t address the more important question of ITALhow firms are innovating and whether this is improving.
The nature and measurement of capital goods - the assets that are put to productive use – have changed significantly in Industry 4.0. In the past, these consisted mainly of investments in large-scale factories and production machinery, and were carefully recorded for accounting and taxation purposes, providing accurate information for governments. Nowadays, intangible investments and activities such as design are much more significant - but these are more difficult to measure. The new digital technologies of Industry 4.0, including AI and Machine Learning, virtual and augmented reality, simulation and modelling methods, and novel, small-scale ways of manipulating matter such as additive manufacturing and genome editing, for example, complicate the recording and classifications of important innovation investments.
The measurement of innovation is changing
Agencies that collect national and international statistics are highly aware of the shortcomings of their approaches and are seeking more relevant ways of measuring innovation performance. The Australian government, for example, which has been a leader in this field, is undertaking a significant review of indicators, including a comprehensive literature review and public consultations.
Important lessons have been learned in these agencies. The shortcomings of input measures, such as R&D, and averages of innovation performance, such as gross expenditure on R&D as a percentage of GDP, can hide skewed distributions and are not helpful. The opportunities for gaming the system at the national and organizational levels are being recognized. And there is awareness of the dangers when poorly determined indicators become targets for governments.
We welcome the improvement in the collection of such information, but believe this needs to be complemented by some radically different approaches.
New opportunities for measurement and insight
There is value in including insights from behavioural science. Ideas for new services, the largest component of modern economies, occur at the point of consumption. This means the behaviours of consumers and ‘user-innovators’ matter more and more. Furthermore, as AI and automation replace repetitive work, and human attributes such as creativity, intuition and empathy become more important, understanding behaviours around innovation becomes crucial. By changing the behaviours of individuals and populations, behavioural science is starting to be used to address significant global issues such as the environment and health. Governments and organizations wishing to improve innovation performance need increasingly to bring insights from behavioural psychology and economics into their metrics. By applying behavioural science to, for example, the adoption of good innovation practices and better risk management, we may get insights into future performance.
Data and digital science provide new opportunities for developing useful innovation metrics. Innovation statistics agencies are exploring the ways in which new sources of data can complement and supplement their work. Analysing social media sites and electronic marketplaces for ideas and employment, such as InnoCentive and LinkedIn, can provide valuable insights. While there are concerns about the self-selecting and potentially unrepresentative nature of the information collected, data-scraping and analytical tools can be used to provide useful new and real-time insights into innovation activity.
Companies such as Digital Science are using AI tools for mapping the trajectories of science, and these could be used for mapping innovation. Scientists have created algorithms and used machine learning to study complex and emerging phenomena such as health and weather forecasting, and these could be repurposed and applied to measuring innovation. Real understanding of innovation requires a deep dive into what goes on within organizations. It has to take into account how risks are assessed, decisions are made and implemented, and how the rocky roads of internal politics and organizational battles over resources are navigated. If government policy is to be informed, quantitative indicators of innovation performance must be complemented with qualitative case studies. For these case studies to be valuable they have to comply with formal research method protocols to ensure they are relevant, accurate and can be compared.
Government innovation policies have to be based upon, and directed towards improving, the performance and practices of the new industrial era. The way innovation occurs is changing - and so the indicators that measure it must respond to this new reality.
Don't miss any update on this topic
Create a free account and access your personalized content collection with our latest publications and analyses.
License and Republishing
World Economic Forum articles may be republished in accordance with the Creative Commons Attribution-NonCommercial-NoDerivatives 4.0 International Public License, and in accordance with our Terms of Use.
The views expressed in this article are those of the author alone and not the World Economic Forum.
The Agenda Weekly
A weekly update of the most important issues driving the global agenda
You can unsubscribe at any time using the link in our emails. For more details, review our privacy policy.
More on Fourth Industrial RevolutionSee all
How governments can attract innovative manufacturing industries and promote 4IR technologies like AI
M.B. Patil and Alok Medikepura Anil
June 24, 2024
John Lombard
June 24, 2024
Pooja Chhabria
June 23, 2024
Sara Al Hudaithy and Anu Devi
June 4, 2024