How the future of computing can make or break the AI revolution
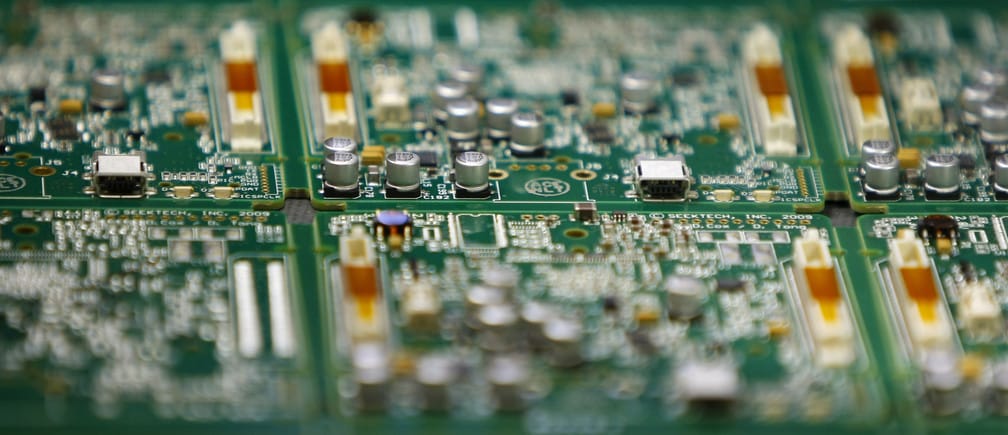
Moore's law, which dictates that the number of transistors per square inch doubles every 18 months to two years, may soon be obsolete. Image: REUTERS/Mike Blake
When it comes to technology trends, AI has been undeniably leading the way in recent years and is expected to continue to do so for decades to come.
From self-driving cars to predictive medicine and personalized learning, AI is increasingly shaping both practical and intimate aspects of our everyday lives, including matching us to our better halves. When talking about AI, we often are interested in the application use cases: what is AI going to enable next? In the race towards building powerful AI systems and applications, the public, including tech-domain experts, often dismisses the close interconnection between AI and computing. However, the AI revolution that we are witnessing could not have happened without the evolution of the computing hardware and of the computing ecosystem.
AI in a nutshell aims at allowing computers to mimic human intelligence. One way to do so is machine learning (ML), consisting of a set of statistical techniques that allow machines to learn from data, instead of being explicitly programmed to perform certain tasks. The most famous ML techniques include regression models, decision trees and neural networks, consisting of adaptive systems of interconnected nodes modeling relationship patterns between input and output data. Multilayered neural networks are particularly relevant for performing complex tasks such as image recognition and text synthesis. These form a subset of machine learning referred to as deep learning (DL), due to the depth of the underlying neural networks. ML techniques in general, and DL techniques in particular, are data- and computing-heavy. A basic deep neural network classifying animal pictures into dogs and cats needs hundreds of thousands of classified animal pictures in training data and billions of iterative computations in order to mimic a four year’s old ability to discern cats from dogs. Data and computing represent in this sense the pillars for building high-performance AI systems.
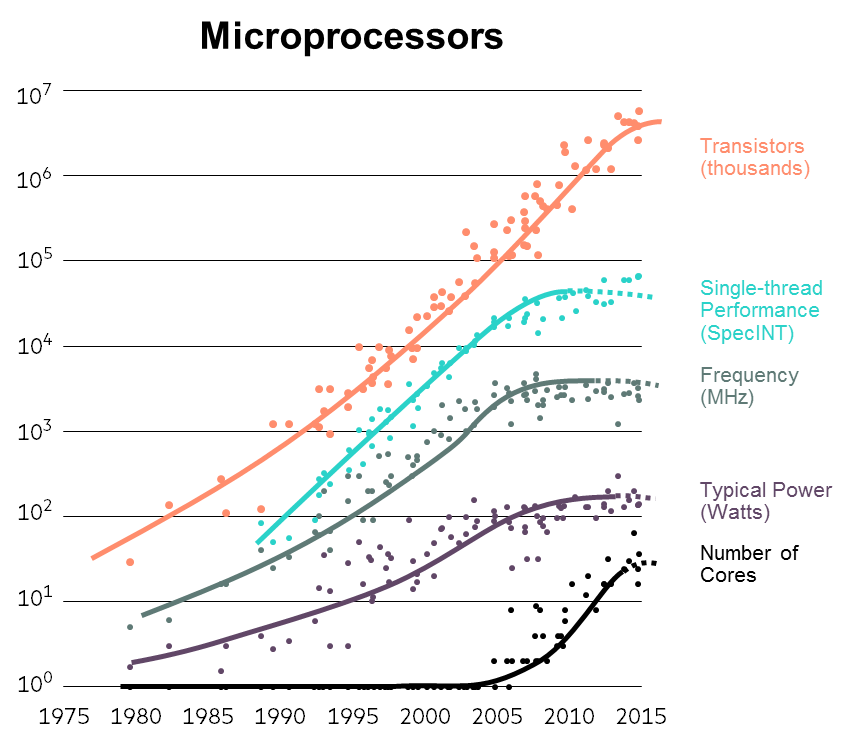
• Moore’s law: Named after Intel co-founder Gordon Moore, Moore’s law states that the number of transistors per square inch in an integrated circuit (IC) has doubled every 18 months to two years since the mid-1960s. Thanks to Moore’s law, computers have become smaller and faster at an exponential rate, reducing computing costs by ~30% per year, allowing the execution of complex mathematical operations on small ICs, and paving the way towards embedded and ubiquitous computing.
• The internet of things (IoT): With more than 40 billion (mini)-computers expected to be connected to the internet by 2020, the IoT is enabling the collection of exabytes of text, voice, image and other forms of training data, feeding into ML and DL models, and increasing the accuracy and precision of these models.
• The rise of application (and AI) specific computing hardware: A computer is composed of many processing units, each of which contains one or many cores or ICs. A CPU (central processing unit) represents the most standardized and general-purpose microprocessor that can be programmed and used for almost any purpose. Recent years have seen the emergence of new types of processing units including GPUs (graphics processing units) and TPUs (tensor processing units). A GPU or TPU ideally contains more cores than a CPU and also presents a different transistors topology at an integrated circuit (IC) level. Due to their specialized architecture, GPUs and TPUs are particularly suited for computing-intensive deep learning applications such as images processing and voice recognition, as well as unstructured text mining.
• The era of exascale computing: Along with data explosion, the evolution of the computing hardware and of the computing needs of applications such as AI and blockchain have driven computing giants such as HPE to start building super-computers. Placed in centralized data centers, super computers are capable of at least one exaFLOPS, or a billion billion (i.e. a quintillion) calculations per second. Such capacity represents a thousand-fold increase over the first petascale computer that came into operation in 2008. China and the EU are also investing millions in the race towards exascale computing.
Due to the high availability and declining costs of in-house and on-demand cloud computing, computing has rarely been considered as a scarce resource when it comes to enabling the AI revolution. However, computer systems are facing many limitations that can slow the development of AI applications built on top. The main challenges include:
• The end of Moore’s law: Due to physical limitations, transistors cannot go in size below a certain level, which is likely to invalid Moore's law sooner than we expect and limit the ability to infinitely shrink microprocessors.
• Increasing data regulation: A speedy exascale processing of data in centralized super-computers requires the data to be stored close to the processors. The increasing appetite for data regulation worldwide, including the recent adoption of GDPR in Europe, is likely to make centralized data placement more difficult and to challenge the purpose of building super-computers in the first place.
• The costs (and feasibility) of data transfer and storage: Building on the last point, the high volume of training data and the bandwidth costs associated with data transfer closer to centralized processors invite a redesign of computer memory and of underlying I/O (input/output) operations, as well as significant investments in the network capacity. Running centralized supercomputers also comes with OPEX and environmental costs, particularly in terms of power consumption and generating CO2 emissions.
• Lack of a computing-supportive ecosystem: As more IT talent goes into tech giants or launches AI startups, the appetite for working for traditional computer manufacturers or for launching startups focused on developing new computing hardware is on the decline. Despite accelerating the prototyping of AI products, the emergence of high-level programming languages, APIs and libraries is contributing to a less nuanced understanding of computing architectures and basic computing operations, even among computer scientists.
The regulatory constraints and the energy and bandwidth costs associated with centralized super-computing are likely to drive data storage and computing to the edge. Intel has already developed a USB stick that allows users to effectively carry out computer vision and image recognition on edge network devices; like smart cameras and augmented reality hardware.
The imminent end of Moore’s law is likely to drive the emergence of more specialized computing architectures, focused on changing the way processing units and individual circuits are structured, in order to achieve performance gains without further shrinking the size of transistors or processors.
On the other hand, a new form of computing, quantum computing, is progressively gaining more ground, although its practical implementation remains a challenge. Quantum computers rethink computing by leveraging the strange laws of quantum mechanics. Instead of using transistors designed around binary units that classical computers use, quantum computers employ “quantum bits” or “qubits”. Unlike bits, which are limited to being either 1 or 0 at all times, qubits can exist in “superposition”, this enables them to simulate multiple states at the same time. Another property of matter at quantum level, “entanglement”, means that multiple qubits can be connected through logic gates. The "superposition" and "entanglement" properties of quantum computing makes it possible to carry many operation streams in parallel both at the level of one "qbit" and many "qbits", thus making a quantum computer more suited to tackle complex computational problems, when compared to a classical computer. This being said, physically building a “universal” quantum computer remains extremely difficult in engineering terms. Creating and maintaining qubits requires stable systems under extreme conditions – for example, maintaining component temperatures very close to absolute zero.
Encouraging more people to go into STEM and retaining tech talent will provide the computer manufacturing industry with the talent that it needs to disrupt itself and keep up with the needs of the AI revolution.
Finally, when building ML models and writing algorithms, data scientists, computer scientists, ML engineers and AI practitioners should consider the least complex way to solve the problem at hand. Going for a complex neural network when a regression could have provided a good accuracy or running data pipelines on a daily basis when a weekly data update could have been enough are real examples of how tech talent is wasting a scarce resource such as computing on a daily basis. Promoting computing-friendly practices within the tech ecosystem has become a necessity for the tech industry to sustain its own growth, namely on the AI front.
Don't miss any update on this topic
Create a free account and access your personalized content collection with our latest publications and analyses.
License and Republishing
World Economic Forum articles may be republished in accordance with the Creative Commons Attribution-NonCommercial-NoDerivatives 4.0 International Public License, and in accordance with our Terms of Use.
The views expressed in this article are those of the author alone and not the World Economic Forum.
Stay up to date:
Data Science
Related topics:
The Agenda Weekly
A weekly update of the most important issues driving the global agenda
You can unsubscribe at any time using the link in our emails. For more details, review our privacy policy.
More on Fourth Industrial RevolutionSee all
Matt Price and Anna Schilling
November 20, 2024