The new assembly lines: Why AI needs low-skilled workers too
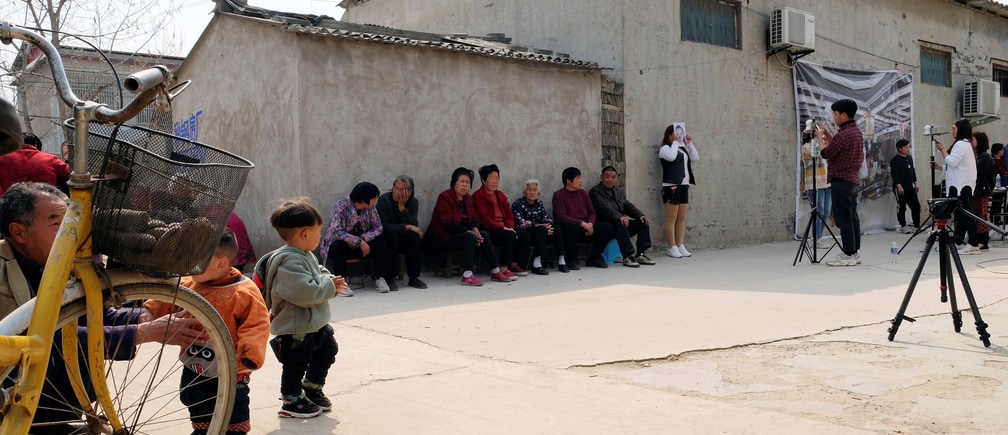
The human end of AI …workers from a Chinese data company take photos of the villagers for a facial recognition project.
Image: REUTERS/Cate Cadell
Stay up to date:
Artificial Intelligence
Whether AI and automation will create, eliminate or modify jobs, and how much, is the central question of a never-ending debate. In 2013, the Oxford academic duo Carl Benedikt Frey and Michael Osborne published a study titled “The Future of Employment: How Susceptible Are Jobs to Computerization?”, which estimated that 47% of American jobs are at high risk by mid-2030.
Last year, the World Economic Forum (WEF) released The Future of Jobs 2018 report, stating that algorithms and intelligent machines are expected to create 133 million new roles globally while displacing around 75 million by 2022 – a total net gain of 58 million jobs.
Regardless of the specific numbers or percentages, the underlying omnipresent message in all of the above-mentioned reports, on the news and on social media, is that automation and artificial intelligence will make low-skilled jobs disappear in favor of specialized, highly knowledgeable workers. Apparently, we are facing the fading of unskilled labor and the rise of software engineers, data scientists, digital communicators and online specialists.
But is this really the case? It is certainly true that automation and AI have the exact scope (and advantage) of replacing repetitive and predictable cognitive and physical tasks. However, there’s a hidden side of artificial intelligence and machine learning that is seldom discussed, and the majority of the public is not even aware of: the new assembly lines of data.
“Data is the new oil” has become a familiar motto nowadays. And it’s definitely true, data is the increasingly valuable new commodity, especially for AI. Most machine-learning algorithms need to be trained on a usually voluminous dataset. This happens because the majority of ML techniques today fall under the umbrella of what is called “supervised learning”: the computer is eventually able to make “inferences” or “decisions”, but only after it has been shown enough examples with their respective “solutions”. We can teach a neural network to recognize pictures of a car by feeding the network with thousands of images of cars while specifying every time to the algorithm: “Hey, this is a car!” The more pictures of cars we give to it, the better it can recognize them.
Now, this training data needs to be annotated by someone – meaning a human. I recently wrote an article specifically around computer vision LiDAR and image annotation that explains the concept, the process and the different labeling techniques. In short, there is someone specifying that in that image there is actually a car, sometimes also stating where it is, what is doing, whether it has the lights on or off, and so on.
Humans are in the loop and vet every – or almost every – image, every line of text and every piece of data fed to neural networks. To give you an idea of the order of magnitude, if we just consider self-driving cars, vehicles will generate 40 terabytes of data every eight hours of driving, according to Intel. Waymo, which is just one of the many autonomous vehicle companies, recently claimed to have driven 16 million kilometres on public roads in the US. You do the math, that’s a huge amount of data.
All of this translates into thousands and thousands of human hours of work. And labeling tasks are not just a prerogative of self-driving cars or autonomous robots. Machine learning is everywhere and so it is annotation, even in healthcare. Pattern-recognizing software is used in radiology, pathology, cardiology, oncology and even psychiatry. Massive datasets encompassing imaging files, CT, MR, ECGs and so on also need to have humans in the loop landmarking tumoral cells, drawing polygons around outliers and highlighting pathologic signals. And the list goes on, including other imaging, text or speech recognition examples across multiple industries and applications.
But when does this take place, how and where? There are various firms who develop the software tools that are utilized by humans to go through the annotation process. Some of them also have internal employees or others outsource the actual manual work of labeling. In every case, these teams of annotators are almost always located in countries where the cost of labor is low: India, China or various African regions. We are talking about very big teams, in the order of thousands. Some companies deal with more than 50,000 people, drawing from a pool of more than a million of annotators worldwide working day and night shifts.
Basically, while factories and manufacturing facilities are becoming smarter, full of robots taking care of manual tasks that used to be carried out by human workers, data annotation farms are the new assembly lines 2.0 in the age of artificial intelligence. These new jobs would not exist without the machine learning algorithms that are powering this revolution. Labeling data can be thought of as the cognitive equivalent of the assembly line, in which workers do not suffer from exhausting physically demanding tasks, but are instead engaged in cognitive efforts. True, it is still a quite repetitive duty, but it is performed on a chair, far from potentially harmful machinery.
Being a data annotator is not an easy job. It requires training and meticulous attention to detail. You need to draw very precise polygons around objects in an image, or pinpoint landmarks using a mouse and a keyboard. And you need to do it with extreme accuracy: the quality of annotated data is vital to the success of a machine-learning algorithm. Moreover, in many industries like autonomous vehicles, bad training data can make the difference between life and death. Annotating that data is time-consuming, but it is a necessary task to teach our machines how to behave, make decisions and predict outcomes.
Given the volume and the characteristics of the tasks, data annotation represents a great opportunity for low-skilled workers, people living in developing countries or groups who have more difficulty accessing jobs.
Deepen AI, for example, an organization operating in the data annotation business, has started lifelong.ai, a nonprofit organization that enables refugees to access jobs and learn new skills that improve their socioeconomic situation. Lifelong trains Syrian refugees in Jordan to become data annotators and connects them with remote opportunities in the AI market, offering free English, web and mobile development courses as well.
Moreover, Deepen is committed to paying its employees a salary that is higher than the minimum wage, on top of having a well-defined ladder for people to grow from labelers to team leads, software QA, to program manager and HR. Thanks to this effort, many of Deepen’s employees in India had the chance to get married, start families, grow and enrich their communities.
It should be now clear how artificial intelligence is not just creating opportunities only for the MScs and PhDs out there. This booming technology is actually giving birth to new categories of jobs for the low-skilled in ways that are hard to predict.
The responsibility is upon companies operating such businesses to empower communities and make sure employees don’t get stuck in low-skilled jobs, but are instead able to grow in knowledge and skills. But amid this landscape of low-skill opportunities, there is a dark side as well. In an attempt to attack the market with hyper-competitive pricing and earn higher margins, some labeling companies are keeping annotators salary tremendously low. Figures can go as low as $1 per hour or even less, which is even below minimum wage. Organizations adopting this line of business are basically sponsoring a new kind of slavery in the digital era.
The hunger for annotated data is so big, that in the short term this approach can be very monetarily rewarding for the companies adopting it. On the long run, instead, such a line of conduct will determine high employee churn rate, bad quality in the output, and negative impact on communities. Not only these firms are upsetting social norms by exploiting workers and conducting business unethically, but this unfair behavior is also detrimental for the whole industry. AI is a controversial technology, with lots of societal, ethical and moral concerns around it. The dirty game of paying ridiculous salaries to data annotators can definitely throw a dark shadow on the sector, damaging trust in technology and ultimately preventing, or slowing down, society from gaining the many benefits that can come from it.
In sum, the advent of automation and AI is eliminating millions of jobs and creating millions of others. It is not necessarily true that all the jobs created by AI are for highly skilled and highly knowledgeable workers; there are also many new opportunities for the low-skilled. The rate at which these are created might not match the rate at which other low-skilled positions are disappearing, but AI it is still in its infancy. Most likely, the availability of data and the need to access annotated datasets will grow exponentially over the next years, meaning a likely steep demand for data annotators globally.
It is very hard to make reliable predictions. Probably the best we can do is to act similarly to our algorithms: look at today’s facts and make some inference based on those. If we just refer to self-driving vehicles, today we know that many more millions, even billions of driven miles separate us from a safe, fully autonomous vehicle … that’s enough to keep a significant amount of data annotators very busy for some time.
Don't miss any update on this topic
Create a free account and access your personalized content collection with our latest publications and analyses.
License and Republishing
World Economic Forum articles may be republished in accordance with the Creative Commons Attribution-NonCommercial-NoDerivatives 4.0 International Public License, and in accordance with our Terms of Use.
The views expressed in this article are those of the author alone and not the World Economic Forum.
Forum Stories newsletter
Bringing you weekly curated insights and analysis on the global issues that matter.
More on Fourth Industrial RevolutionSee all
Kirsty Paine and Filipe Beato
April 29, 2025
Ronit Avni
April 28, 2025
Gustavo Maia
April 22, 2025
Cathy Hackl
April 21, 2025