Medical data has a silo problem. These models could help fix it.
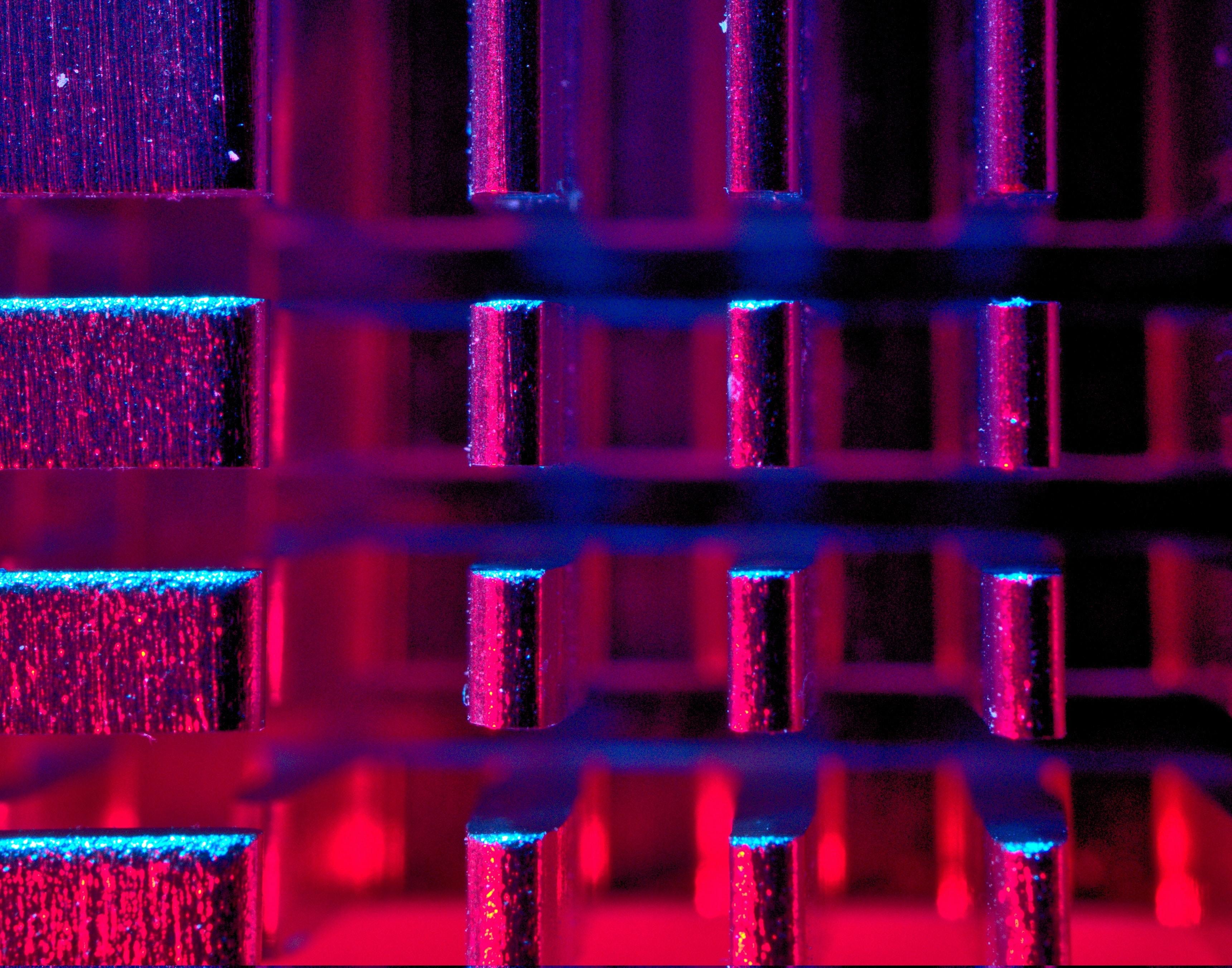
Image: Photo by Markus Spiske on Unsplash
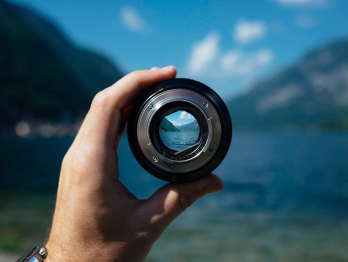
Get involved with our crowdsourced digital platform to deliver impact at scale
Stay up to date:
Precision Medicine
- Much health data is out of reach to researchers in specific countries, halting discovery and innovation.
- Government policies must consider technological capabilities to ensure data's true potential can be unlocked.
Every day, more and more data about our health is generated. Data, which if analyzed, could hold the key to unlocking cures for rare diseases, help us manage our health risk factors and provide evidence for public policy decisions. However, due to the highly sensitive nature of health data, much is out of reach to researchers, halting discovery and innovation. The problem is amplified further in the international context when governments naturally want to protect their citizens’ privacy and therefore restrict the movement of health data across international borders. To address this challenge, governments will need to pursue a special approach to policymaking that acknowledges new technology capabilities.
What is the World Economic Forum doing about data sharing?
Understanding data siloes
Data becomes siloed for a range of well-considered reasons ranging from restrictions on terms-of-use (e.g., commercial, non-commercial, disease-specific, etc), regulations imposed by governments (e.g., Safe Harbor, privacy, etc.), and an inability to obtain informed consent from historically marginalized populations.
Siloed data, however, also creates a range of problems for researchers looking to make that data useful to the general population. Siloes, for example, block researchers from accessing the most up-to-date information or the most diverse, comprehensive datasets. They can slow the development of new treatments and therefore, curtail key findings that can lead to much needed treatments or cures.
Even when these challenges are overcome, the incidences of data mis-use - where health data is used to explore non-health related topics or without an individual’s consent - continue to erode public trust in the same research institutions that are dependent on such data to advance medical knowledge.
Solving this problem through technology
Technology designed to better protect and decentralize data is being developed to address many of these challenges. Techniques such as homomorphic encryption (a cryptosystem that encrypts data with a public key) and differential privacy (a system leveraging information about a group without revealing details about individuals) both provide means to protect and centralize data while distributing the control of its use to the parties that steward the respective data sets.
Federated data leverages a special type of distributed database management system that can provide an alternative approach to centralizing encoded data without moving the data sets across jurisdictions or between institutions. Such an approach can help connect data sources while accounting for privacy. To further forge trust in the system, a federated model can be implemented to return encoded data to prevent unauthorized distribution of data and learnings as a result of the research activity.
To be sure, within every discussion of the analysis of aggregated data lies challenges with data fusion between data sets, between different studies, between data silos, between institutions. Despite there being several data standards that could be used, most data exist within bespoke data models built for a single purpose rather than for the facilitation of data sharing and data fusion. Furthermore, even when data has been captured into a standardized data model (e.g., the Global Alliance for Genomics and Health offers some models for standardizing sensitive health data), many data sets are still narrowly defined. They often lack any shared identifiers to combine data from different sources into a coherent aggregate data source useful for research. Within a model of data centralization, data fusion can be addressed through data curation of each data set, whereas within a federated model, data fusion is much more vexing.
Addressing Ethical Concerns
Independent of the technology and architectural solutions currently used, there are several overarching ethical challenges that must be considered and addressed by professional societies and tenure committees. The easiest is the professional attribution for all contributors - case and controls, if you will - to the data corpus. Research results often depend just as much on the positives as they do the negatives; it is the controls or the “normals” that can provide the necessary contrast to identify the positives.
The second ethical challenge is assuring proper credit and provenance. Giving creators priority in a resource’s meta data over subsequent citations and attributions can ensure transparency and authenticity, and guarantee intellectual property is shared. Furthermore, understanding who the data-rights holders are can ensure that their rights are considered as data is shared.
This topic is under active discussion thanks to the FAIR movement for open science and data sharing, a movement driven by scholars, funders and publishers to ensure that data could be Findable, Accessible, Interoperable and Reusable. Still, the issue is far from being fully addressed, and challenges in this area continue to complicate activities such as from the publication of research to the analysis of data for new advancements.
"To truly unlock the potential of new data and technologies, research communities will need to re-evaluate their governance models within a context of evolving privacy regulations globally."
”An important ethical issue, one that is not widely discussed, involves the process through which contributors of data can share in the rewards that might result from the use of their data for research and discovery. Within an institutional model of data control, institutions sell or license access to data sets without regard for the data contributors as beneficiaries. If data is being federated, each distributed data set might have a different fee structure for data access that might need to be factored into the decisions around what data to access for each research project. Each contributing institution will use different criteria to assign an inherent value that could skew research based upon economics rather than being optimized for discovery. Moreover, this practice by institutions does not recognize the contributions of the individuals about whom the data is collected in the first place or encourage the return of results which could have clinically relevant findings. This lack of attribution is out of step with the recognition that individuals’ data has value and each individual should be connected with this intrinsic value going forward.
Looking ahead
To truly unlock the potential of new data and technologies, research communities will need to re-evaluate their governance models within a context of evolving privacy regulations globally. Such an approach could reconcile data controlled by institutions with the rights of individuals on behalf of data citizens.
One can argue that putting the control of data in the hands of the individual addresses the many issues around trust. Historically, lack of trust has limited participation in research, to the detriment of the broader communities in need of improved therapies and treatments.
This re-evaluation of governance models should also extend into considerations around how rewards from research discoveries are shared to adequately recognize the contributions of the data contributors.
Additionally, governments worldwide can make space within their regulatory frameworks to promote future-proof data sharing techniques. These could account for federated learning techniques that facilitate the sharing of data analysis at an international level without the data itself having to move. Given the sensitivities around health data this is an important step forward to ensure that siloes can be broken without compromising control.
This month, the World Economic Forum launched the Roadmap for Cross-Border Data Flows, identifying a range of best practices from around the world to help governments develop forward leaning cross-border data flows policy. The recommendations can apply to regional and international data collaboration as well as innovation in data-intensive technologies. The Roadmap specifically highlights technological solutions such as federated learning as being key to fully unlocking data’s potential in applications such as international health data research and innovation. Later this summer, on July 22, the Forum will also release a genomics-specific federated data governance model.
Such guidance can help governments develop the policy levers that strike the delicate balance required to unlock data’s value while still ensuring proper data sharing safeguards are in place. With this guidance, researchers – and the general public - can more fully benefit from data and knowledge flows and keep pace with Fourth Industrial Revolution advancements.
Don't miss any update on this topic
Create a free account and access your personalized content collection with our latest publications and analyses.
License and Republishing
World Economic Forum articles may be republished in accordance with the Creative Commons Attribution-NonCommercial-NoDerivatives 4.0 International Public License, and in accordance with our Terms of Use.
The views expressed in this article are those of the author alone and not the World Economic Forum.
The Agenda Weekly
A weekly update of the most important issues driving the global agenda
You can unsubscribe at any time using the link in our emails. For more details, review our privacy policy.