Trust in facial recognition technology can be achieved. Here’s how
A software engineer works on a facial recognition program that identifies people when they wear a face mask at the development lab of the Chinese electronics manufacturer Hanwang (Hanvon) Technology in Beijing as the country is hit by an outbreak of the novel coronavirus (COVID-19), China, March 6, 2020. Picture taken March 6, 2020. Image: REUTERS/Thomas Peter
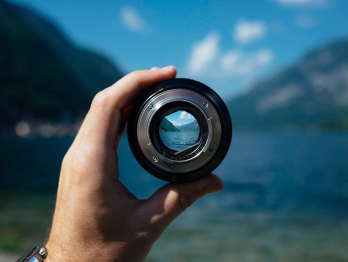
Get involved with our crowdsourced digital platform to deliver impact at scale
Stay up to date:
Global Governance
- The potential to misuse facial recognition software (FRS) has raised fundamental questions around ethical use, privacy and accessibility.
- Those developing facial recognition software can develop standards to help ensure ethical and responsible technology design.
Facial recognition software (FRS) has hit the headlines of late and rightly so. Its potential for misuse has raised fundamental questions around ethical use, privacy and accessibility. In some cases, major companies have even halted development and some NGOs have called for total bans.
Such moves have moved some to push for more standardization in the development of facial recognition technology as many realize that it can be used to conduct unacceptable infringement of liberties. The chief of police in one major US city even pointed out that FRS’ low level of accuracy could lead authorities to misidentify someone 96% of the time if officers were to leverage the technology just by itself.
To help mitigate these fears, those developing facial recognition software can develop standards to help ensure ethical and responsible technology design. Such human-centered standards can help forge trust and protect against bias or misapplication.
What is algorithm bias?
Over the past year, the World Economic Forum Artificial Intelligence team found that responsible limits can be set on facial recognition technology. Its AI team worked alongside public and private sectors to develop a unique FRS framework anchored in responsible development for engineers and deployment for policymakers.
The framework is the first to go beyond general principles and to operationalize commercial use cases under the banner of ethical and responsible AI. It comprises four simple steps:
1. Draft principles that define responsible use.
Facial recognition has gained special importance as COVID-19 has pushed contactless services as a safe way to verify identities. While touchless and passwordless technology is the path ahead, companies can pick the direction of travel.
To guide that journey – and prevent misuse – companies can draft a set of ‘Principles for Action’ that define what constitutes responsible facial recognition technology. These principles should focus on 7 key areas to protect users from any misuse of the technology and be developed in consultation with industry players, policymakers, advocacy groups and academics with the goal of reaching a high level of consensus between these stakeholders. These areas include:
- Privacy: Protecting end user’s personal data
- Risk assessment: Building processes to mitigate risks of errors and its consequences
- Proportional use of technology: Assessing the trade-off between usage risks and opportunities
- Accountability: Defining the responsibility of platform providers and organizations using the technology
- Consent: Naming the rules for free consent by end-users
- Accessibility: Making sure the technology accommodates differently-abled people
- Adaptability: Offering fallback options as situations change and having humans on deck for oversight
To ensure the effective adoption of these Principles for Action, they must be embedded at the core of business operations through internal processes and oversight to lessen potential risks.
Taking these points into consideration can ensure a high level of security and respect of data privacy. Their application could manifest mechanisms such as autodeleting biometric data after 24 hours, conducting impact assessments among designers to identify any potential bias, or designing public signage so passers know when an FRS system is running.
"Human-centered standards can help forge trust and protect against bias or misapplication."
”2. Design systems to support product teams.
In developing ‘responsible by design’ technologies, product teams will need special support, best practices, and systems for testing or quality control. Factors to consider when designing systems to provide this support will include:
- Facial recognition justification
- A data plan matching end-user characteristics
- Bias risk mitigation
- Methods to inform end-users
To take each factor into account, strong collaborations between the organization using the technology and the platform provider will help ease any risks. For example, in bias risk mitigation, algorithms provided by platform providers must be trained with data that will truly represent the users of the service and the system tested to ensure it acts properly before release. To do so, organizations should assess the diversity of its end-users and share it with the platform provider to train the algorithm accordingly.
While acknowledging that biases may still occur, organizations should anticipate this risk by building fallback systems that are robust enough to provide the same level of service to anyone and thus diminish any form of discrimination due to algorithm biases.
3. Auto-assess your work.
Best practices and principles cannot exist in a vacuum. Auto-assessing is a necessary step to check the Principles of Action are being respected and to identify potential blind spots. To that end, an assessment questionnaire can help groups test how well the systems they designed matched the standards they’d set.
For example, when it comes to assessing their proportional use, organizations can check if they have considered alternatives to FRS and documented the reasons they have rejected them. They can also analyse the level of false negatives and false positives and determine if this level is suitable for the use case they deploy. They can also compare their results between skin tones to attest that the system doesn’t produce any form of discrimination.
When gaps are found, the assessment process can drive teams to refer to their best practices to take internal actions to bridge gaps and improve their level of accountability and trustworthiness for their customers.
4. Validate compliance through a third-party audit.
Too often, companies only rely on home-made labels to build transparency among users. The quality of those labels can sometimes be questionable, and also pose a systemic risk of mistrust for the industry that could consequently undermine wider efforts to build transparency.
Being audited by a third and independent party to deliver certification in line with the Principles for Action could be one path forward. Lessons from the accounting industry can be applied, adding additional transparency and safety through independent and accredited agencies. [For example, to draft our FRS framework, the World Economic Forum Artificial Intelligence team partnered with AFNOR Certification, the French leader of certification and assessment services.]
These evaluations should occur right after the deployment of systems for end-users and be conducted regularly to attest to the respect of standards over time. By doing so, certified organizations will be able to communicate among their customers to show their compliance across a range of requirements.
While certification is widely used in many industries and services, facial recognition, despite its high level of scrutiny, is still deployed without any existing certification. We believe that transparency and trust in this field can only be achieved with such mechanisms.
Looking ahead
These four steps can help inform the design of responsible systems for flow management use cases in FRS. They can also ensure that their designers and users are effectively compliant with these achievable principles.
Certification is a reachable step towards the regulation of FRS. The cooperation between industry actors, policymakers, academics and civil society on this policy project has shown a strong willingness for standards for the commercial use of FRS.
Yet, Governments have to step in to adopt bills that will ensure a sustainable regulation of FRS along with international standards defining what a responsible use of the technology should be. They also need to address the thorny question of FRS for law enforcement and determine the right levels of oversight and accountability for its related use-cases.
The recent call for regulation by organizations such as IBM, Microsoft and Amazon should be followed by new regulations or guidance or we’ll likely see a deployment of FRS that will lead to mistrust and consumer avoidance.
Don't miss any update on this topic
Create a free account and access your personalized content collection with our latest publications and analyses.
License and Republishing
World Economic Forum articles may be republished in accordance with the Creative Commons Attribution-NonCommercial-NoDerivatives 4.0 International Public License, and in accordance with our Terms of Use.
The views expressed in this article are those of the author alone and not the World Economic Forum.
Related topics:
The Agenda Weekly
A weekly update of the most important issues driving the global agenda
You can unsubscribe at any time using the link in our emails. For more details, review our privacy policy.
More on Global CooperationSee all
Børge Brende
June 27, 2024
Pooja Chhabria
June 27, 2024
Spencer Feingold
June 26, 2024
Spencer Feingold
June 25, 2024
Blair Palmer
June 21, 2024