This AI technique could use a digital version of Earth to help fight climate change
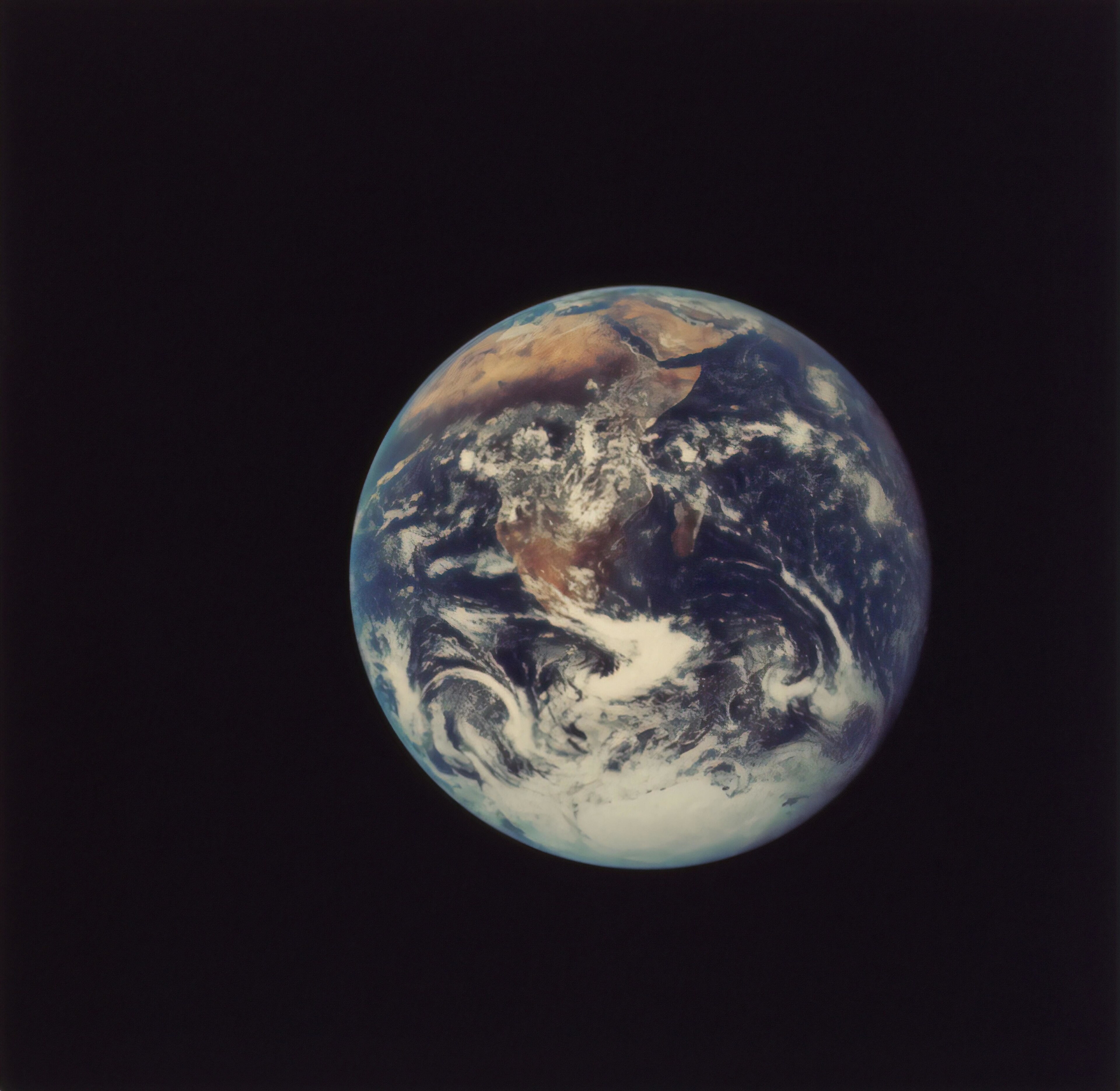
Image: New York Public Library/Unsplash
- An AI technique called reinforcement learning could help us solve some of the world's most complex problems.
- It enables an algorithm to learn how to perform a task through trial and error in a simulator, or digital twin.
- Applying this technique to test climate-saving initiatives across a digital twin of Earth could help us tackle climate change.
Last year we embarked on an ambitious project with the Emirates Team New Zealand sailing team to build an AI bot that could sail a digital version of any type of boat design they engineered in digitally simulated, real-world sailing conditions. This would allow engineers to test various boat designs much faster than having to secure time with the team’s human sailors, who could only step away from practice a few hours here and there.
To sail as well as the world’s best sailors, the AI bot needed to learn to execute many different maneuvers in varying conditions, choosing the best course to set under a wide variety of winds and seas, adjusting 14 different boat controls accordingly, assessing the results of its decisions, and continually improving decisions over long time horizons.
We trained the bot using an AI technique called reinforcement learning, which enables an algorithm to learn how to perform a task through trial and error as it tests actions in a simulator, or digital twin, and receives instant feedback on those actions through a reward system.
Getting the bot to sail at such an elite level was a highly complex problem to tackle. But this type of complex problem that has a dynamic environment containing many variables and many possible actions and paths to choose from is where reinforcement learning excels.
The power of reinforcement learning
There are applications for this in nearly every industry. For example, whereas once retailers could reasonably expect that past consumer behaviors would indicate future preferences, they now operate in a world where consumer purchase patterns and preferences evolve rapidly—all the more so as the COVID-19 pandemic repeatedly redefines life. Manufacturers and consumer-packaged-goods companies are under pressure to build dynamic supply chains that account for climate, political and societal shifts anywhere in the world at a moment’s notice. Each of these challenges represents a complex and highly dynamic optimization problem, which, with the right data and feedback loops, is well suited for solving with reinforcement learning.
After completing the project, we took a step back to consider how an increasingly viable technique like reinforcement learning could be applied to pressing societal challenges. Reinforcement learning is good at solving complex optimization problems and predicting the next best action. Could the technology be used, for example, to identify and prioritize areas in desperate need of food assistance and optimize its distribution worldwide?
How it could fight climate change
In the example of climate change, could reinforcement learning be applied to a digital twin of the Earth to test how a multitude of separate climate-saving initiatives across the globe can best be sequenced and combined into a mutually reinforcing whole? Several developments may be converging to make this feasible.
First, we have enough data: the global datasphere is set to reach 175 zettabytes by 2025, and satellites constantly beam data to Earth that can be used to advance our understanding of how and why the climate is changing. In June 2016, for example, GHGSat launched the first high-resolution satellite capable of capturing atmospheric measurements from any industrial facility in the world and calculating the associated greenhouse-gas emissions. In another example, AI-enabled satellite imagery is allowing NCX to take accurate inventories of the world’s forests, providing information that can help us grow bigger, stronger trees that absorb more CO2.
Ocean monitoring has improved dramatically, too. The US National Oceanic and Atmospheric Administration, for instance, monitors ocean temperatures, currents, levels and chemistry using thousands of buoys and floats that take daily measurements at surface and deep levels.
Second, some of the world’s largest companies are waking up to their environmental responsibilities, launching many climate-saving initiatives with ambitious goals. Reinforcement learning could asses the effects of these initiatives.
A few examples:
- Microsoft has pledged to invest $1 billion in climate measures and eliminate carbon emissions by 2030.
- Starbucks has vowed to store more carbon than it emits, eliminate waste and provide more clean water than it uses.
- Google has switched to 100% renewable sources for its energy supply, uses a reinforcement learning algorithm to cool its data centers for energy savings of about 40%, and is working with DeepMind to use ML algorithms to predict wind levels and schedule energy deliveries to the grid accordingly.
- At McKinsey & Company, we have pledged to reach net-zero climate impact by 2030 and have already switched to 95% renewable energy sources and have offset 100% of our greenhouse gas emissions since 2018.
Third, the cost and complexity of implementing reinforcement learning are coming down. The latest iterations in reinforcement learning algorithms are much more efficient to train to perform tasks, substantially driving down compute costs. At the same time, the cost of compute itself has declined significantly. Companies can now access specialized systems in the cloud and pay only for what they use. Cloud providers have also ramped up efforts to deliver prepackaged, enterprise-ready reinforcement learning frameworks that can be deployed in assembly-line fashion to eliminate some of the manual coding and integration work.
Finally and importantly, in March the European Commission announced its "Destination Earth" initiative in which scientists will work to create a digital twin of the Earth that enables the mapping of climate change and the assessment of solutions that could slow or reverse it. The EU plans to open the digital Earth model up for use by industry over time. The models generated by the Destination Earth initiative could provide a testing ground to determine whether reinforcement learning could analyze climate initiatives across the world, gauging their collective effect and determining what further actions need to be taken to halt or reverse climate change.
This won't be easy or serve as a silver bullet. But as new AI tools and other technologies continue to become more viable and powerful, we should look for such ways to combine efforts in industry, academia and public institutions to help us collectively move the needle on important global issues.
Don't miss any update on this topic
Create a free account and access your personalized content collection with our latest publications and analyses.
License and Republishing
World Economic Forum articles may be republished in accordance with the Creative Commons Attribution-NonCommercial-NoDerivatives 4.0 International Public License, and in accordance with our Terms of Use.
The views expressed in this article are those of the author alone and not the World Economic Forum.
Stay up to date:
Fourth Industrial Revolution
Related topics:
Forum Stories newsletter
Bringing you weekly curated insights and analysis on the global issues that matter.
More on Fourth Industrial RevolutionSee all
Mihir Shukla
December 23, 2024