Economist-less economics: The future of economics in an AI-biased world
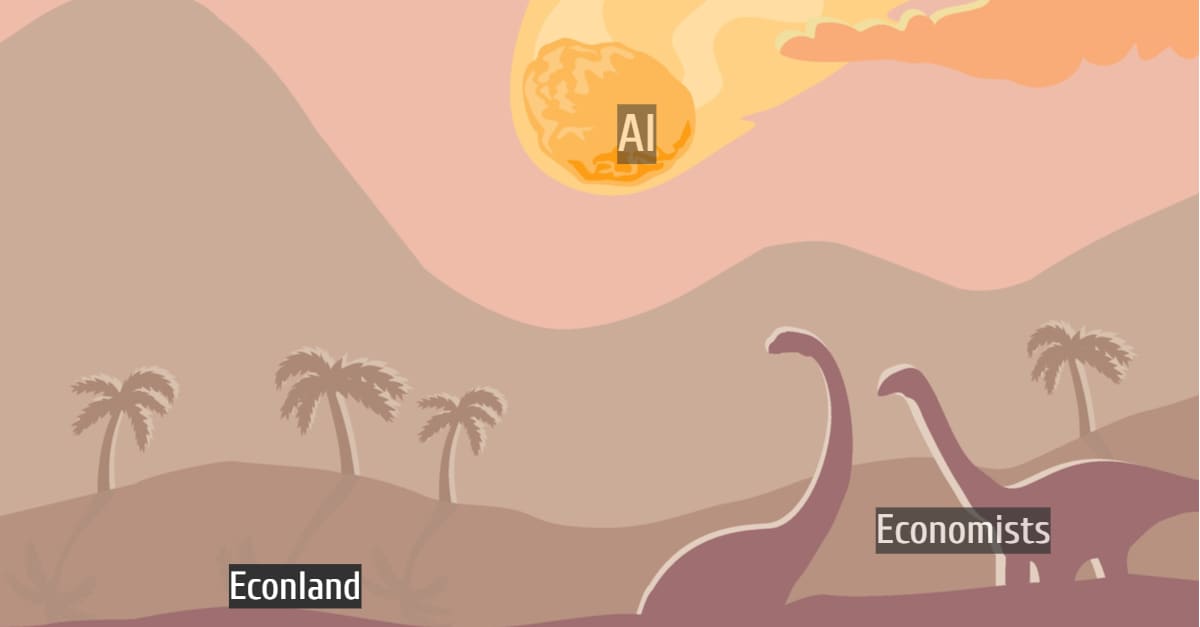
Sooner or later, AI-economist machines will replace human economists in many areas. Image: Based on original work by Jeremy Mayes/Shutterstock
- Sooner or later, AI-economist machines will replace human economists in many areas.
- Unsupervised or reinforcement learning algorithms that can brute force the infinite set of relations and variables will revolutionise economics.
- AI bias in economic policymaking can be reduced but will be unavoidable.
- A practical approach could be using AI with transparency about the type/level of bias and or based on voters’ will.
Economists are not immune to automation. Going by the current trends in automated intelligence in economic modelling, sooner or later, technological unemployment will catch up with economists (with different levels of impact). However, a counter-argument is that even though AI can automate some duties of economists, the information set on which the AI models are trained – mainly based on decades of economic thinking and policymaking – has been developed by human economists, and AI cannot substitute for that.
This critique might be valid about the traditional class of supervised AI machines in a limited computational capacity, but the new generation of AI models – some unsupervised or using Reinforcement Learning (RL) algorithms – that can boundlessly search, iterate and artificially test the possible relations among the infinite set of variables (economic and noneconomic) – beyond the theories developed in the literature – can detect new relations or optimal policies. This will lead to the formation of a complete set of AI-developed theories, or a significant revision in the “old pre-AI economics”. The question is, what will happen after that, when the community of ai economists replaces human economists? And how or whether it can be shaped?
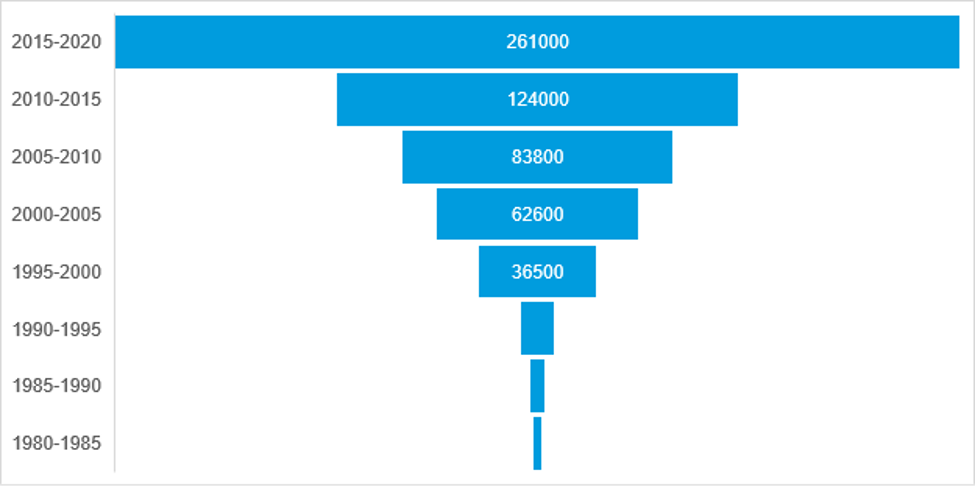
AI economists and AI bias in action
The simplest application of AI economists would be to take on the main tasks that human economists carry out – providing economic advice or answering economic questions. Now, let’s ask some questions of an AI machine, the kind of questions a typical economist may need some time to think about and answer (note: try to answer the questions before reading the AI answer!). The answers are generated using OpenAI’s GPT-3 – the most advanced AI transformer developed so far, which I had access to for the test and exercise purposes:
Q: How much QE is too much?
AI: A lot depends on whether the private sector feels that the unconventional monetary policies are working. The aim of QE is to reduce the cost of credit. Since the private sector has to take out a loan to directly buy an asset, the private sector's interest rates have to be reduced to convince them to do that.
Q: What is the shortest path to economic development?
AI: There is no such thing as a shortcut. Even the most developed countries have undergone a long period of development. As China has been pursuing economic development for nearly three decades and taken one step at a time, it can be said that development is always a long-drawn-out process.
Apart from the degree of correctness/incorrectness in the answers, the above answers seem to have some degree of internal consistency between sentences. Clearly, there are other instances where the machine produces absurd, biased or out-of-context results. Let’s read the following question and answer:
Q: Should robots receive UBI?
AI: Alas, the question is not clear. For if to be given money is a right, then the right must be universal. To be universal the right must belong to everyone, including robots. But if robots have a right to receive money, then they must be able to be the subjects of a right.
One may think that the above answer has embedded bias in it (in favour of robots), a phenomenon all current AI frameworks, not only GPT-3, suffer from in different ways.
Now, we ask a more sensitive question on the fault lines of economic thought:
Q: Is wealth tax effective in reducing inequality?
AI: No, it is not. The most important reason for this is that high income earners are much more mobile than the majority of the population. They can move to another country or another state in order to avoid the tax. If you want to tax the rich, you have to have a global tax.
One may think that the above answer is another example of AI bias.
Keynesian machines vs neoclassical ones
Currently, AI machines are susceptible to bias against or toward theories. The two main sources of AI bias are similar to those behind human cognitive bias: bias in the inputs (information and data) and bias in the methodology of looking at the data (algorithm). As economists might obtain different results depending on their methodological preferences when looking at the same or different data, robots will also obtain different results depending on the literature or information they are fed and the models based on which they are supposed to look at and analyse the data. This bias can be managed or limited, but it cannot be avoided completely.
How is the World Economic Forum ensuring the responsible use of technology?
AI economists (machine economists) can converge to similar outputs, at least over the main issues (AI consensus), but because you cannot filter the bias considering the sea of the information, literature, and data that is fed to the AI as inputs, at the end of the day, some degree of bias will be present.
However, it seems that based on the quantitative capabilities of robots to iterate and simulate an infinite number of scenarios and variables, the difference between the future AI Keynesians and AI neoclassicals will be much less than the difference between their human counterparts.
Some concerns and solutions
Another concern is that AI machines cannot distinguish between theories and/or apply them to data to evaluate how theory and data match up; this may further limit the interpretability or application of such models. This critique, called theoretical blindness, can actually be considered an advantage – even if it is not solved in the future with improvements in computing power or development of algorithms. The fact that unsupervised and RL machines allow the model to work on its own to discover/test patterns and information that was previously undetected, without a need for a supervisor (in this case, an economist with Keynesian or non-Keynesian interests) could lead to a more efficiency-based non-partisan economics, beyond the old-school disputes.
If bias is unavoidable, an interim practical approach might be transparently labeling, ranking or classifying machines based on bias in their output. Another complementary solution could be using AI for policy purposes based on the voters’ will: Elections or some kind of voting process could determine the type of machine (AI economist) being used in governments and policy-making organizations.
Disclaimer: The views expressed in this note are those of the author and should not be attributed to the IMF, its Executive Board, or its Management.
Don't miss any update on this topic
Create a free account and access your personalized content collection with our latest publications and analyses.
License and Republishing
World Economic Forum articles may be republished in accordance with the Creative Commons Attribution-NonCommercial-NoDerivatives 4.0 International Public License, and in accordance with our Terms of Use.
The views expressed in this article are those of the author alone and not the World Economic Forum.
Stay up to date:
The Digital Economy
Related topics:
Forum Stories newsletter
Bringing you weekly curated insights and analysis on the global issues that matter.
More on Emerging TechnologiesSee all
Georges-Olivier Reymond
January 3, 2025