What would it take to make AI ‘greener’?
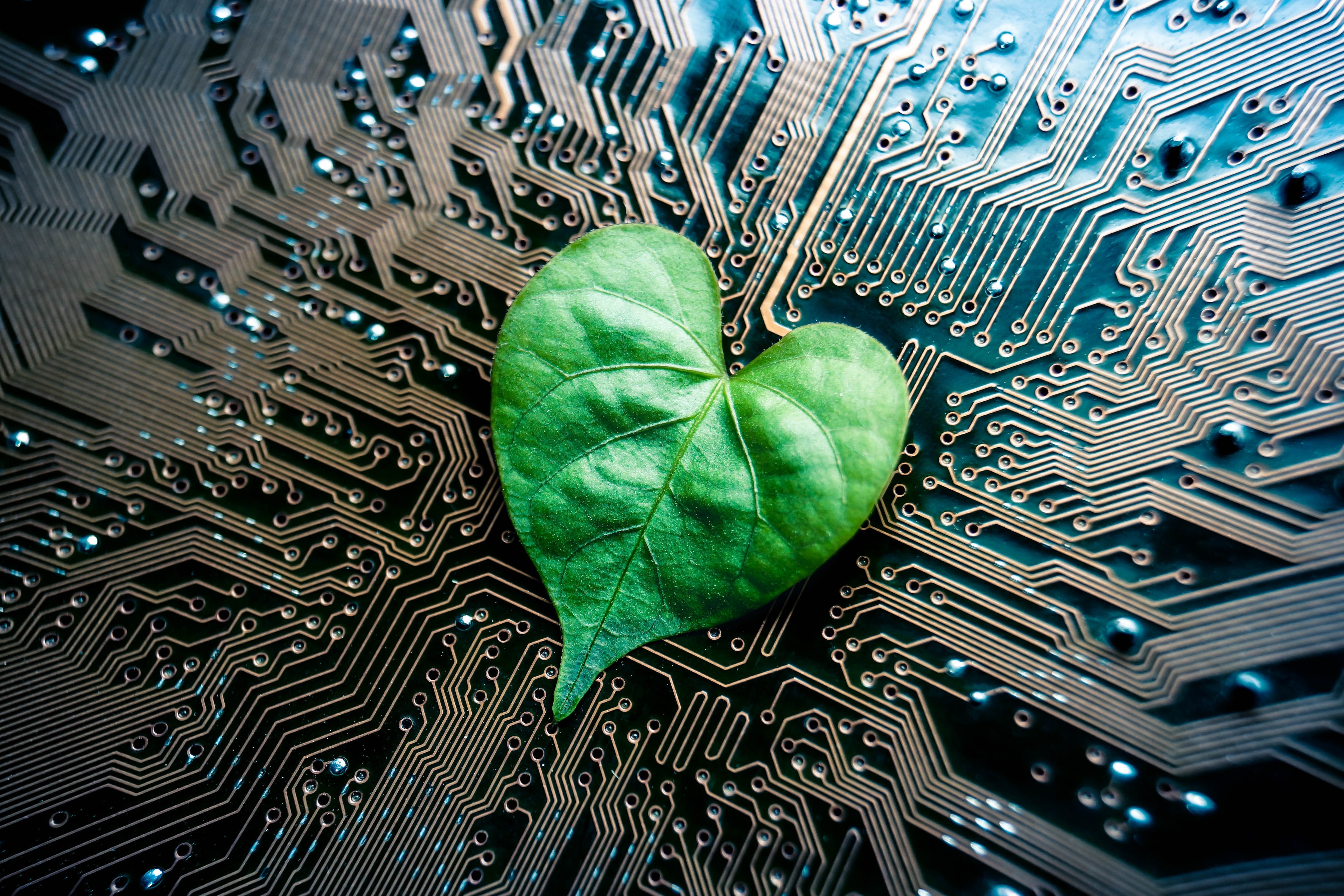
AI can become a sustainable tech of the future and a major asset in the protection of our global climate. Image: Shutterstock
Listen to the article
- AI can be a powerful tool to combat climate change, but its role also as a contributor to emissions cannot be overlooked.
- The first step to make AI greener is to promote the practice of more holistic and multidimensional model evaluation.
- By changing our mindset that bigger is always better and by pursuing AI use cases in the environmental space, AI can be a major asset in the fight against climate change.
With record heat waves globally and extreme flooding impacting Europe and China, now is a pivotal moment to interrogate the interplay of technology and the environment, including the role of artificial intelligence (AI).
What would it take to make AI ‘greener’? On the one hand, we first need to collectively recognize that there are tangible costs to the creation and use of AI systems – and, in fact, they can be quite large. GPT-3, a recent powerful language model by OpenAI, is estimated to have consumed enough energy in training to leave a carbon footprint equivalent to driving a car from Earth to the moon and back.
There are beneficial impacts that AI can have on our relationship to the environment as well. A comprehensive study in 2020 assessed the potential impact of AI on the United Nations’ 17 Sustainable Development Goals, encompassing societal, economic and environmental outcomes. The researchers found that AI could positively enable 93% of the environmental targets, including the creation of smart and low-carbon cities; Internet-of-Things devices and appliances that can modulate their consumption of electricity; better integration of renewable energy through smart grids; the identification of desertification trends via satellite imagery; and combating marine pollution.
Cement and telecom
AI use cases in industry can serve to help the environment and reduce carbon emissions. For example, OYAK Cimento, a Turkish based cement manufacturing group is using AI to significantly reduce their carbon footprint. According to Berkan Fidan, Performance & Process Director at OYAK Cimento: “Enterprise AI-assisted process control helps to increase operational efficiency, which means higher production with lower unit energy consumption. If we consider a single moderate capacity level cement plant with 1 million tons of cement production, just a 1% of additional clinker reduction – with AI-assisted process and quality control – produces a reduction of around 7,000 tons of CO2 per year. This equals CO2 absorption of 320,000 trees in a year.”
According to the think tank Chatham House, cement accounts for approximately 8% of CO2 emissions. Thus, there is a clear environmental need to improve efficiency in cement manufacturing and one tool to do so is AI.
Another example of AI having a positive environmental impact concerns Entel, the largest Chilean telecommunications company, and sensor data to identify forest fires. It takes a collaborative effort to successfully fight forest fires that have been raging in many parts of the world, including Greece and Northern California. Chile is frequently impacted by severe climate change and catastrophic weather conditions, which previously led to the worst wildfire in Chile’s history in 2017 that resulted in the burning of around 714,000 acres. For a country steeped in natural wonder, with a population and economy that depends heavily on thriving forests, any type of wildfire is a devastating tragedy.
Entel Ocean, the digital unit of Entel, sought to identify fires earlier using IoT sensors. These sensors act as a digital “nose” placed on trees, capable of detecting particles in the air. The data produced by these sensors enabled Entel Ocean to use AI for automatically predicting when a forest fire would start. “We have been detecting a forest fire 12 minutes before traditional methods – this is a big deal when it comes to preventing fires,” says Lenor Ferrebuz Bastidas, enterprise digital solutions spokesperson for Entel Ocean. “Considering fire can spread in a matter of seconds, every minute helps.”
Trade-offs
Through these applications, AI can be a powerful tool to combat climate change. But its role also as a contributor cannot be overlooked. To that end, the first step is to promote the practice of more holistic and multidimensional model evaluation. To date, the major focus of research and innovation has been on improving accuracy or creating new algorithm methods. These aims often consume larger and larger amounts of data, building ever more complex models. The most telling example is in deep learning, where computational resources went up 300,0000 times between 2012-2018.
Yet, the relationship between model accuracy and complexity is logarithmic. For exponential increases in model size and training requirements, there are linear improvements to performance. In the hunt for accuracy, less priority is given to developing methods with improved time-to-train or resource efficiency. Moving forward, we need to recognize the trade-off between model accuracy and efficiency and the model’s carbon footprint, regarding both during training and when making inferences.
What is the World Economic Forum’s Sustainable Development Impact summit?
The carbon footprint of a model can be complicated to determine and compare across modelling approaches and data centre infrastructures. A reasonable place to start may be by assessing the number of floating-point operations – that is, a discrete count of how many simple mathematical operations (for example, multiplication, division, addition, subtraction, and variable assignment) – that need to be performed to train a model. This factor and others can impact energy consumption along with the architecture of the model and the training resources, such as hardware like GPU or CPUs. Additionally, the physical considerations of the storage and cooling of the servers comes into play. As a final complication, it also matters where the energy is sourced from. Energy primarily from renewable resources compared to natural gas or coal will have a reduced carbon footprint.
Let’s ask: “How much more can we do with less?” Taking into account energy-conserving constraints may drive us towards new and creative innovations in AI. By pivoting to this mindset instead of bigger is always better and by pursuing AI use cases in the environmental space, AI can remain at the cutting edge, becoming a sustainable technology of the future and a major asset in the protection of our global climate.
Don't miss any update on this topic
Create a free account and access your personalized content collection with our latest publications and analyses.
License and Republishing
World Economic Forum articles may be republished in accordance with the Creative Commons Attribution-NonCommercial-NoDerivatives 4.0 International Public License, and in accordance with our Terms of Use.
The views expressed in this article are those of the author alone and not the World Economic Forum.
Stay up to date:
Climate Crisis
Forum Stories newsletter
Bringing you weekly curated insights and analysis on the global issues that matter.
More on Forum InstitutionalSee all
Beatrice Di Caro
December 17, 2024