Scientists used AI to create smarter health records and improve patient care
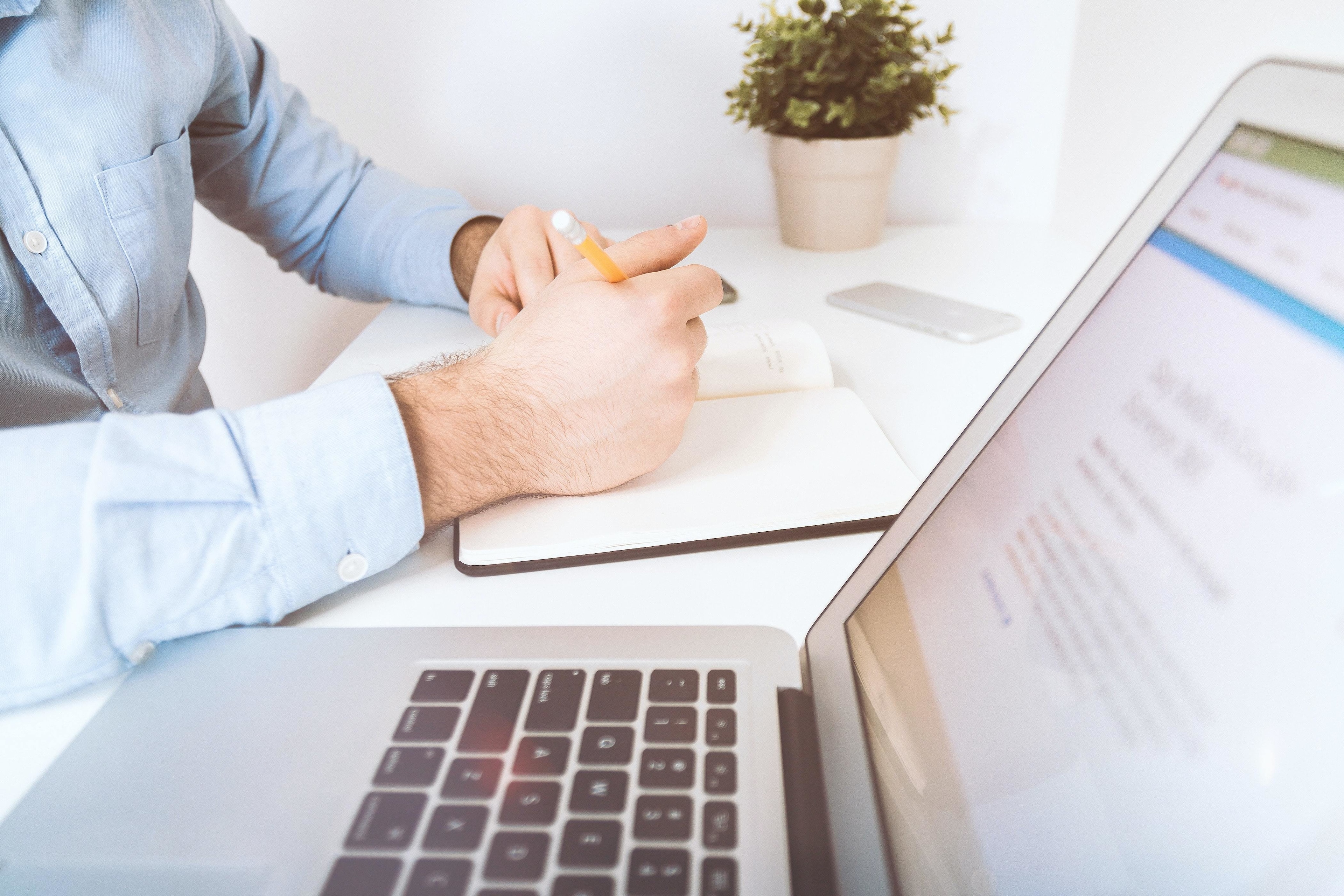
MedKnowts is 'a system that unifies the processes of looking up medical records and documenting patient information into a single, interactive interface.' Image: UNSPLASH/JESHOOTS.COM
- Researchers at MIT and the Beth Israel Deaconess Medical Center are using machine learning and human-computer interaction to create an electronic health record (EHR) called MedKnowts.
- This is a system that combines the process of looking up medical records and documenting patient information into a single, interactive interface.
- It is hoped this technology can become more widespread and be developed further, to improve the quality of patient care.
Electronic health records have been widely adopted with the hope they would save time and improve the quality of patient care. But due to fragmented interfaces and tedious data entry procedures, physicians often spend more time navigating these systems than they do interacting with patients.
Researchers at MIT and the Beth Israel Deaconess Medical Center are combining machine learning and human-computer interaction to create a better electronic health record (EHR). They developed MedKnowts, a system that unifies the processes of looking up medical records and documenting patient information into a single, interactive interface.
Driven by artificial intelligence, this “smart” EHR automatically displays customized, patient-specific medical records when a clinician needs them. MedKnowts also provides autocomplete for clinical terms and auto-populates fields with patient information to help doctors work more efficiently.
“In the origins of EHRs, there was this tremendous enthusiasm that getting all this information organized would be helpful to be able to track billing records, report statistics to the government, and provide data for scientific research. But few stopped to ask the deep questions around whether they would be of use for the clinician. I think a lot of clinicians feel they have had this burden of EHRs put on them for the benefit of bureaucracies and scientists and accountants. We came into this project asking how EHRs might actually benefit clinicians,” says David Karger, professor of computer science in the Computer Science and Artificial Intelligence Laboratory (CSAIL) and senior author of the paper.
The research was co-authored by CSAIL graduate students Luke Murray, who is the lead author, Divya Gopinath, and Monica Agrawal. Other authors include Steven Horng, an emergency medicine attending physician and clinical lead for machine learning at the Center for Healthcare Delivery Science of Beth Israel Deaconess Medical Center, and David Sontag, associate professor of electrical engineering and computer science at MIT and a member of CSAIL and the Institute for Medical Engineering and Science, and a principal investigator at the Abdul Latif Jameel Clinic for Machine Learning in Health. It will be presented at the Association for Computing Machinery Symposium on User Interface Software and Technology next month.
A problem-oriented tool
To design an EHR that would benefit doctors, the researchers had to think like doctors.
They created a note-taking editor with a side panel that displays relevant information from the patient’s medical history. That historical information appears in the form of cards that are focused on particular problems or concepts.
For instance, if MedKnowts identifies the clinical term “diabetes” in the text as a clinician types, the system automatically displays a “diabetes card” containing medications, lab values, and snippets from past records that are relevant to diabetes treatment.
Most EHRs store historical information on separate pages and list medications or lab values alphabetically or chronologically, forcing the clinician to search through data to find the information they need, Murray says. MedKnowts only displays information relevant to the particular concept the clinician is writing about.
“This is a closer match to the way doctors think about information. A lot of times, doctors will do this subconsciously. They will look through a medications page and only focus on the medications that are relevant to the current conditions. We are helping to do that process automatically and hopefully move some things out of the doctor’s head so they have more time to think about the complex part, which is determining what is wrong with the patient and coming up with a treatment plan,” Murray says.
Pieces of interactive text called chips serve as links to related cards. As a physician types a note, the autocomplete system recognizes clinical terms, such as medications, lab values, or conditions, and transforms them into chips. Each chip is displayed as a word or phrase that has been highlighted in a certain color depending on its category (red for a medical condition, green for a medication, yellow for a procedure, etc.)
Through the use of autocomplete, structured data on the patient’s conditions, symptoms, and medication usage is collected with no additional effort from the physician.
Sontag says he hopes the advance will “change the paradigm of how to create large-scale health datasets for studying disease progression and assessing the real-world effectiveness of treatments.”
In practice
After a year-long iterative design process, the researchers tested MedKnowts by deploying the software in the emergency department at Beth Israel Deaconess Medical Center in Boston. They worked with an emergency physician and four hospital scribes who enter notes into the electronic health record.
Deploying the software in an emergency department, where doctors operate in a high-stress environment, involved a delicate balancing act, Agrawal says.
“One of the biggest challenges we faced was trying to get people to shift what they currently do. Doctors who have used the same system, and done the same dance of clicks so many times, form a sort of muscle memory. Whenever you are going to make a change, there is a question of is this worth it? And we definitely found that some features had greater usage than others,” she says.
The Covid-19 pandemic complicated the deployment, too. The researchers had been visiting the emergency department to get a sense of the workflow, but were forced to end those visits due to Covid-19 and were unable to be in the hospital while the system was being deployed.
Despite those initial challenges, MedKnowts became popular with the scribes over the course of the one-month deployment. They gave the system an average rating of 83.75 (out of 100) for usability.
Scribes found the autocomplete function especially useful for speeding up their work, according to survey results. Also, the color-coded chips helped them quickly scan notes for relevant information.
Those initial results are promising, but as the researchers consider the feedback and work on future iterations of MedKnowts, they plan to proceed with caution.
“What we are trying to do here is smooth the pathway for doctors and let them accelerate. There is some risk there. Part of the purpose of bureaucracy is to slow things down and make sure all the i’s are dotted and all the t’s are crossed. And if we have a computer dotting the i’s and crossing the t’s for doctors, that may actually be countering the goals of the bureaucracy, which is to force doctors to think twice before they make a decision. We have to be thinking about how to protect doctors and patients from the consequences of making the doctors more efficient,” Karger says.
A longer-term vision
The researchers plan to improve the machine learning algorithms that drive MedKnowts so the system can more effectively highlight parts of the medical record that are most relevant, Agrawal says.
They also want to consider the needs of different medical users. The researchers designed MedKnowts with an emergency department in mind — a setting where doctors are typically seeing patients for the first time. A primary care physician who knows their patients much better would likely have some different needs.
In the longer-term, the researchers envision creating an adaptive system that clinicians can contribute to. For example, perhaps a doctor realizes a certain cardiology term is missing from MedKnowts and adds that information to a card, which would update the system for all users.
The team is exploring commercialization as an avenue for further deployment.
How is the World Economic Forum bringing data-driven healthcare to life?
“We want to build tools that let doctors create their own tools. We don’t expect doctors to learn to be programmers, but with the right support they might be able to radically customize whatever medical applications they are using to really suit their own needs and preferences,” Karger says.
This research was funded by the MIT Abdul Latif Jameel Clinic for Machine Learning in Health.
Don't miss any update on this topic
Create a free account and access your personalized content collection with our latest publications and analyses.
License and Republishing
World Economic Forum articles may be republished in accordance with the Creative Commons Attribution-NonCommercial-NoDerivatives 4.0 International Public License, and in accordance with our Terms of Use.
The views expressed in this article are those of the author alone and not the World Economic Forum.
Stay up to date:
Global Health
The Agenda Weekly
A weekly update of the most important issues driving the global agenda
You can unsubscribe at any time using the link in our emails. For more details, review our privacy policy.
More on Emerging TechnologiesSee all
Filipe Beato and Jamie Saunders
November 21, 2024