What are the dangers of unregulated AI? An expert explains
If unregulated, AI technologies could generate adverse social consequences.
Image: REUTERS/Aly Song TPX IMAGES OF THE DAY
Daron Acemoglu
Professor of Applied Economics, Department of Economics, Massachusetts Institute of Technology (MIT)Stay up to date:
Data Science
- Daron Acemoğlu, Professor of Applied Economics at MIT explains why greater regulation is needed for current AI technologies.
- Without it, he argues that AI technologies could generate adverse social consequences.
- The main problem is not AI itself, but the way leading firms are approaching data and their use.
- Acemoğlu concludes that policy should focus on redirecting technological change to create new capabilities and opportunities for workers and citizens.
Artificial intelligence (AI) is often touted as the most exciting technology of our age, promising to transform our economies, lives, and capabilities. Some even see AI as making steady progress towards the development of ‘intelligence machines’ that will soon surpass human skills in most areas. AI has indeed made rapid advances over the last decade or so, especially owing to the application of modern statistical and machine learning techniques to huge unstructured data sets. It has already influenced almost all industries: AI algorithms are now used by all online platforms and in industries that range from manufacturing to health, finance, wholesale, and retail. Government agencies have also started relying on AI, particularly in the criminal justice system and in customs and immigration control.
In a recent paper (Acemoglu 2021), I argue that current AI technologies — especially those based on the currently dominant paradigm relying on statistical pattern recognition and big data — are more likely to generate various adverse social consequences, rather than the promised gains.
These harms can be seen in product markets and advertising, in terms of inequality, wage suppression and job destruction in labour markets, and in the broader societal effects of AI in the context of social communication, political discourse, and democracy.
AI, control of information, and product markets
In all of these cases, the main problem is not AI technologies per se but the way that leading firms, which have an overwhelming effect on the direction of AI technology, are approaching data and its use.
Take the use of machine learning and big data methods in advertising and product design. Although, in principle, these methods can benefit consumers – for instance, by improving product quality and enabling customisation – they can ultimately have various adverse effects on consumer welfare. To start with, firms that acquire more information about their customers may use this knowledge for price discrimination, potentially capturing more of the rents that would have otherwise gone to consumers. In an oligopolistic market, harvesting of consumer data can relax price competition as well. Intuitively, this can happen when price discrimination by a firm that has superior knowledge makes its core clientele less attractive to other businesses, encouraging them to raise their prices. This upward pressure on prices would, of course, further damage consumer welfare.
Other uses of these new techniques could be even more detrimental to consumers. For one, online platforms may come to control excessive amount of information about their users, because when they buy or acquire the data of some users, this also provides information about other users. This type of ‘data externality’ is more likely to arise when users directly reveal information about their friends and contacts, or when they are sharing information correlated with the information of others are in the same narrow demographic group. Data externalities can contribute to too much data being concentrated in the hands of companies, with adverse implications for privacy and consumer surplus (Acemoglu et al. 2021b).
Even worse, companies can use their superior information about consumer preferences to manipulate their behaviour (e.g. Zuboff 2019). Behavioural manipulation is not common in models in which consumers are fully rational. However, it is quite likely when consumers do not fully understand how much new data collection and processing methods used to track and predict their behaviour. The basic idea of such manipulation was understood by legal analysts of antitrust, such as Hanson and Kysar who observed that “once one accepts that individuals systematically behave in non-rational ways, it follows from an economic perspective that others will exploit those tendencies for gain” (1999: 630). Indeed, advertising has always involved some element of manipulation. However, the extent of such manipulation may have become amplified by AI tools. There are already several examples of AI-based manipulation. These include the chain store Target successfully forecasting whether women are pregnant and sending them hidden ads for baby products, or various companies estimating ‘prime vulnerability moments’ and advertising for products that tend to be purchased impulsively during such moments. They may also include platforms such as YouTube and Facebook using their algorithms to estimate and favour more addictive videos or news feeds to specific groups of users.
AI and labour market inequality
The effects of AI-based technologies in the context of the labour market may be even more pernicious. Labour market inequality has increased in the US and several other advanced economies, and much evidence suggests that this is caused in part by rapid adoption and deployment of automation technologies that displace low and middle-skill workers from the tasks they used to perform (Acemoglu and Restrepo 2021). Such automation and its adverse inequality consequences predate AI. Nevertheless, Acemoglu et al. (2021a) find that the acceleration of AI in the US since 2016 has targeted automation and has had similar effects to other automation technologies. AI and extensive use of data are likely to multiply automation possibilities, and thus can exacerbate the inequality trends the US and other advanced economies have experienced over the last several decades.
In principle, automation can be efficiency-enhancing. However, there are also reasons to expect that it can take place inefficiently. An important reason for this is the presence of labour market imperfections, which increase the cost of labour to firms above its social opportunity cost. Under this scenario, firms will automate in order to shift rents away from workers to themselves, even when such automation reduces social surplus.
Other uses of AI can have even more powerfully negative consequences. These include the use of AI and workplace data in order to intensify worker monitoring. Once again, when there are worker rents (either because of bargaining or efficiency wage considerations), greater monitoring can be beneficial for firms in order to claw these rents back from the workers. But with the same reasoning, such rent-shifting is socially inefficient and excessive – at the margin, it is a costly activity that does not contribute to social surplus but transfers it from one set of agents to another.
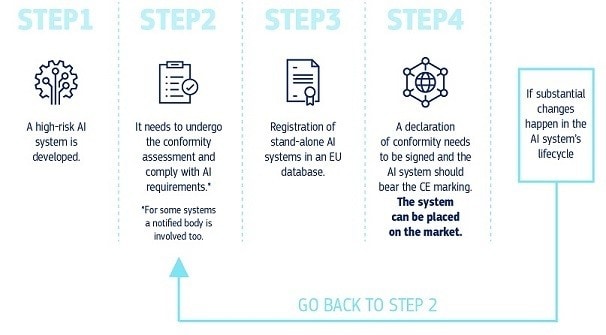
AI, social discourse, and democracy
AI-based automation can have other negative effects as well. Although it is not likely to lead to mass unemployment anytime soon (and disemployment effects from other automation technologies have so far been modest), worker displacement has various socially disruptive effects. Citizens with lower attachments to jobs may participate less in civic activities and politics (Sandel 2020). Even more importantly, automation shifts the balance of power away from labour towards capital, and this can have far-ranging implications on the functioning of democratic institutions. Put differently, to the extent that democratic politics depends on different labour and capital having countervailing powers against each other, automation may damage democracy by making labour dispensable in the production process.
AI’s effects on democracy are not confined to its impact by automation. One of the domains that has been most radically transformed by AI so far is communication and news consumption, especially via the products and services offered by various social media platforms. The use of AI and harvesting of user data have already changed social discourse, and existing evidence is that they have contributed to polarisation and diminished the shared understanding of facts and priorities that is critical for democratic politics (e.g. Levy 2021). As Cass Sunstein anticipated 20 years ago, “fragmentation and extremism… are predictable outcomes of any situation which like-minded people speak only with themselves”. He stressed that “without shared experiences, a heterogeneous society will have a much more difficult time in addressing social problems” (Sunstein 2001: 9). Indeed, AI-powered social media appears to have contributed both to this type of fragmentation and extremism on the one hand, and the spread of misinformation on the other (e.g. Vosoughi et al. 2018).
How is the World Economic Forum ensuring the responsible use of technology?
A problem of direction of technology
The tone of this essay so far may create the impression that AI is bound to have disastrous social consequences, and that I am staunchly against this technology. Neither is true. AI is a promising technological platform. The problem lies with the current direction in which this technology is being developed and used: to empower corporations (and sometimes governments) at the expense of workers and consumers. This current approach is a consequence of the business practices and priorities of the corporations controlling AI, and in the incentives that this creates for AI researchers.
Take social media. A major reason for the problems I emphasised is that platforms are trying to maximise engagement by ensuring that users are ‘hooked’. This objective is rooted in their business model, which is centred on monetising data and consumer traffic by advertising. It is further enabled by the fact that they are unregulated.
The same is true when it comes to the negative effects of automation. AI can be used for increasing human productivity and for generating new tasks for workers (Acemoglu and Restrepo 2018). The fact that it has been used predominantly for automation is a choice. This choice of the direction of technology is driven by leading tech companies’ priorities and business models centred on algorithmic automation.
The more general point is that the current path of AI empowers corporations at the expense of workers and citizens, and often also provides additional tools for control to governments for surveillance and sometimes even repression (such as new censorship methods and facial recognition software).
Conclusion: The need for regulation
This reasoning leads to a simple conclusion: the current problems of AI are problems of unregulated AI, which ignores its broader societal and distributional consequences. In fact, it would be naïve to expect that unregulated markets would make the right trade-offs between societal ills and profits from monopolisation of data.
This perspective also suggests that the problem is not just one of monopoly power. If there were more than a few large tech companies, there is no guarantee that they would have different business models and different approaches to AI. Hence, anti-trust is not the most potent, and certainly not a sufficient, tool for dealing with the potential harms of AI. Instead, policy should focus on redirecting technological change away from automation and harvesting of data to empower corporations and towards those that create new capabilities and opportunities for workers and citizens. It should also prioritise the systematic regulation of collection and harvesting of data and use of new AI techniques for manipulating user behaviour and online communication and information exchange.
Given the pervasive nature of AI and data, I would also suggest a new regulatory approach, which can be termed a ‘precautionary regulatory principle’: ex-ante regulation should slow down the use of AI technologies, especially in domains where redressing the costs of AI become politically and socially more difficult after large-scale implementation.
AI technologies impacting political discourse and democratic politics may be prime candidates for the application of such a precautionary regulatory principle. To the extent that (excessive) automation and its social consequences would also be hard to reverse, so is the use of AI for automation and labour market monitoring.
Accept our marketing cookies to access this content.
These cookies are currently disabled in your browser.
References
Acemoglu, D (2021), “Harms of AI”, Oxford Handbook of AI Governance, forthcoming.
Acemoglu, D and P Restrepo (2018), “The Race Between Man and Machine: Implications of Technology for Growth, Factor Shares and Employment”, American Economic Review 108(6): 1488--1542.
Acemoglu, D and P Restrepo (2019), “Automation and New Tasks: How Technology Changes Labor Demand”, Journal of Economic Perspectives 33(2): 3--30.
Acemoglu, D and P Restrepo (2021), “Tasks, Automation and the Rise in US Wage Inequality”, NBER Working Paper No. 28920.
Acemoglu, D, D H Autor, J Hazell and P Restrepo (2021a), “AI and Jobs: Evidence from Online Vacancies”, NBER Working Paper No. 28257, forthcoming Journal of Labor Economics.
Acemoglu, D, A Makhdoumi, A Malekian and A Ozdaglar (2021b), “Too Much Data: Prices and Inefficiencies in Data Markets”, American Economic Journal: Micro, forthcoming.
Hanson, J D and D A Kysar (1999), “Taking Behavioralism Seriously: Some Evidence of Market Manipulation”, New York University Law Review 74: 630.
Levy, R (2021), “Social Media, News Consumption, and Polarization: Evidence from a Field Experiment”, American Economic Review 111(3): 831-870.
Sandel, M J (2020), The Tyranny of Merit: What's Become of the Common Good?, New York, NY: Penguin Press.
Sunstein, C (2001), Republic.com, Princeton, NJ: Princeton University Press.
Vosoughi, S, D Roy and S Aral (2018), “The Spread of True and False News Online”, Science 359: 1146--1151.
Zuboff, S (2019), The Age of Surveillance Capitalism: The Fight for a Human Future at the New Frontier of Power, London, UK: Profile Books.
Accept our marketing cookies to access this content.
These cookies are currently disabled in your browser.
Don't miss any update on this topic
Create a free account and access your personalized content collection with our latest publications and analyses.
License and Republishing
World Economic Forum articles may be republished in accordance with the Creative Commons Attribution-NonCommercial-NoDerivatives 4.0 International Public License, and in accordance with our Terms of Use.
The views expressed in this article are those of the author alone and not the World Economic Forum.
Forum Stories newsletter
Bringing you weekly curated insights and analysis on the global issues that matter.
More on Emerging TechnologiesSee all
Ibrahim Abdullah Alshunaifi and Caroline Tasse
April 3, 2025
David Elliott
April 2, 2025
Eli Joseph
March 28, 2025