Why AI bias may be easier to fix than humanity’s
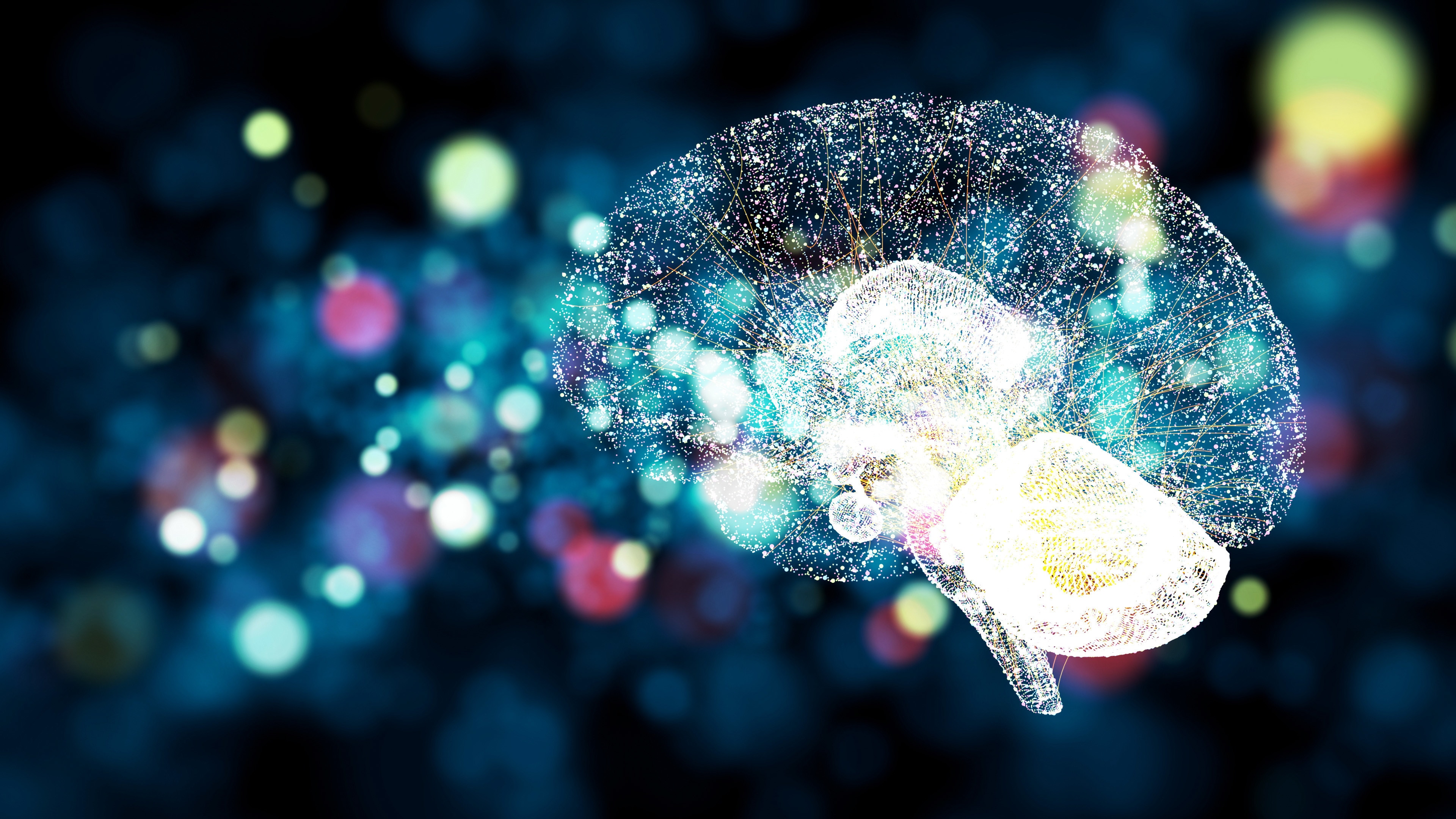
Mistakes on AI bias are most often made when those evaluating algorithms focus on data going into decision-making rather than whether the outcomes are fair. Image: Getty Images/iStockphoto
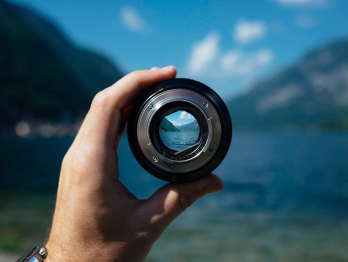
Get involved with our crowdsourced digital platform to deliver impact at scale
Stay up to date:
Artificial Intelligence
Listen to the article
- Artificial intelligence (AI) can produce biased outcomes as its algorithms are based on design choices made by humans that are rarely value-neutral.
- However, this should not put people off as recognizing that AI is inclined to perpetuate inequities may give us an advantage in the fight for fairness.
- By analysing the common characteristics of inequitable outcomes, and by putting sensitive information back into datasets, we can help address AI bias.
The fact that artificial intelligence (AI) can produce biased outcomes should not surprise us. Its algorithms are based on design choices made by humans that are rarely value-neutral.
We also ask the algorithms to produce outcomes that replicate past decision-making patterns where our preconceptions may come to play as well. But what if we don’t want the future to look the same as the past, especially if fairness is in question?
The mere probability that using AI can lead to unfair outcomes shouldn’t require us to swear off it — or put it on hold, as several prominent technologists have suggested. Just the opposite.
Recognizing that AI is inclined to perpetuate inequities may give us a leg up in the fight for fairness. At the end of the day, it would no doubt be easier to mitigate AI’s biases than it has been to remedy those perpetuated by people.
That’s because a lack of fairness in AI can be systematized and quantified in a way that makes it more transparent than human decision-making, which is often plagued by unconscious prejudices and myths.
AI doesn’t create bias. Rather, it serves as a mirror to surface examples of it — and it’s easier to stop something that can be seen and measured.
AI fairness must be a priority
But first, we must look in that mirror. Governments and companies need to make AI fairness a priority, given that algorithms are influencing decisions on everything from employment and lending to healthcare.
Currently, the United States and European Union are driving efforts to limit the rising instances of artificial intelligence bias through Equal Employment Opportunity Commission oversight in the US and the AI Act and AI Liability Directive in the EU.
The focus initially should be on certain sectors where AI bias can potentially deny access to vital services. The best examples include credit, healthcare, employment, education, home ownership, law enforcement and border control. Here, stereotypes and prejudices regularly propagate an inequitable status quo that can lead to shorter life expectancy, unemployment, homelessness and poverty.
Control of artificial intelligence bias must begin with testing algorithm outcomes before they are implemented. Mistakes on AI bias are most often made when those evaluating algorithms focus on data going into decision-making rather than whether the outcomes are fair.
In most cases, because of the complexity of AI models and the lives of the people they touch, we can’t always anticipate the potential disparate impacts from AI’s recommendations which is where the bias manifests.
To do this reliably, central databases of such sensitive data as age, gender, race, disability, marital status, household composition, health and income would need to be created by the private sector or government against which AI-driven models can be tested and corrected for bias.
Such “AI fairness” datasets would allow employers to check for bias in job eligibility requirements before deploying them and universities could proactively analyse AI recommendations for the influence of an applicant’s economic status, gender, race or disability on acceptance.
Data isn’t always neutral
Until recently, many felt the answer to eliminating bias was to delete gender and ethnic identifiers from algorithms altogether. If the algorithm didn’t know the race or gender of candidates, decisions wouldn’t be made on that basis. That assumption proved wrong, with numerous instances of algorithms still being able to determine the race and gender of candidates from anonymized data.
Take lending. If gender and race are removed, artificial intelligence will still favour white males who statistically have more consistent income histories and more considerable assets — which are themselves results of unfair employment practices.
How is the World Economic Forum ensuring the responsible use of technology?
Because a credit algorithm attempts to replicate past lending patterns, it will deny loans disproportionately to those not white and male, as it underestimates their likelihood to repay loans based on past biased results and less data.
Another example: Banks also use willingness to provide mobile phone numbers as an indicator that loan recipients will repay debt. Since women are statistically more reluctant to relinquish mobile phone numbers, they immediately are at a disadvantage to men looking for loans.
AI accuracy matters too
Outcomes also need to be tested for accuracy, the lack of which can also bias results. For instance, when it comes to generative AI, such as ChatGPT, we are currently not seeing, nor are we demanding, a level of accuracy and truthfulness in outcomes, creating another avenue for AI bias to propagate. Chat AI can’t test the factual basis of inputs and simply mimics patterns, desirable or not.
If we analyse the common characteristics of inequitable outcomes by putting sensitive information back into datasets, we can more effectively address AI bias. But it will mean using artificial intelligence to find its own shortcomings when it comes to fairness.
Don't miss any update on this topic
Create a free account and access your personalized content collection with our latest publications and analyses.
License and Republishing
World Economic Forum articles may be republished in accordance with the Creative Commons Attribution-NonCommercial-NoDerivatives 4.0 International Public License, and in accordance with our Terms of Use.
The views expressed in this article are those of the author alone and not the World Economic Forum.
The Agenda Weekly
A weekly update of the most important issues driving the global agenda
You can unsubscribe at any time using the link in our emails. For more details, review our privacy policy.
More on Emerging TechnologiesSee all
Henry Ajder
July 19, 2024
Sebastian Buckup
July 18, 2024
Nii Simmonds and Ayodele Okeowo
July 17, 2024
Jerome Desbonnet and Oded Vanunu
July 16, 2024
Tariq Malik and Prerna Saxena
July 12, 2024
Stefanie Ólives
July 12, 2024