How organizations can bridge the gap between AI enthusiasm and AI implementation
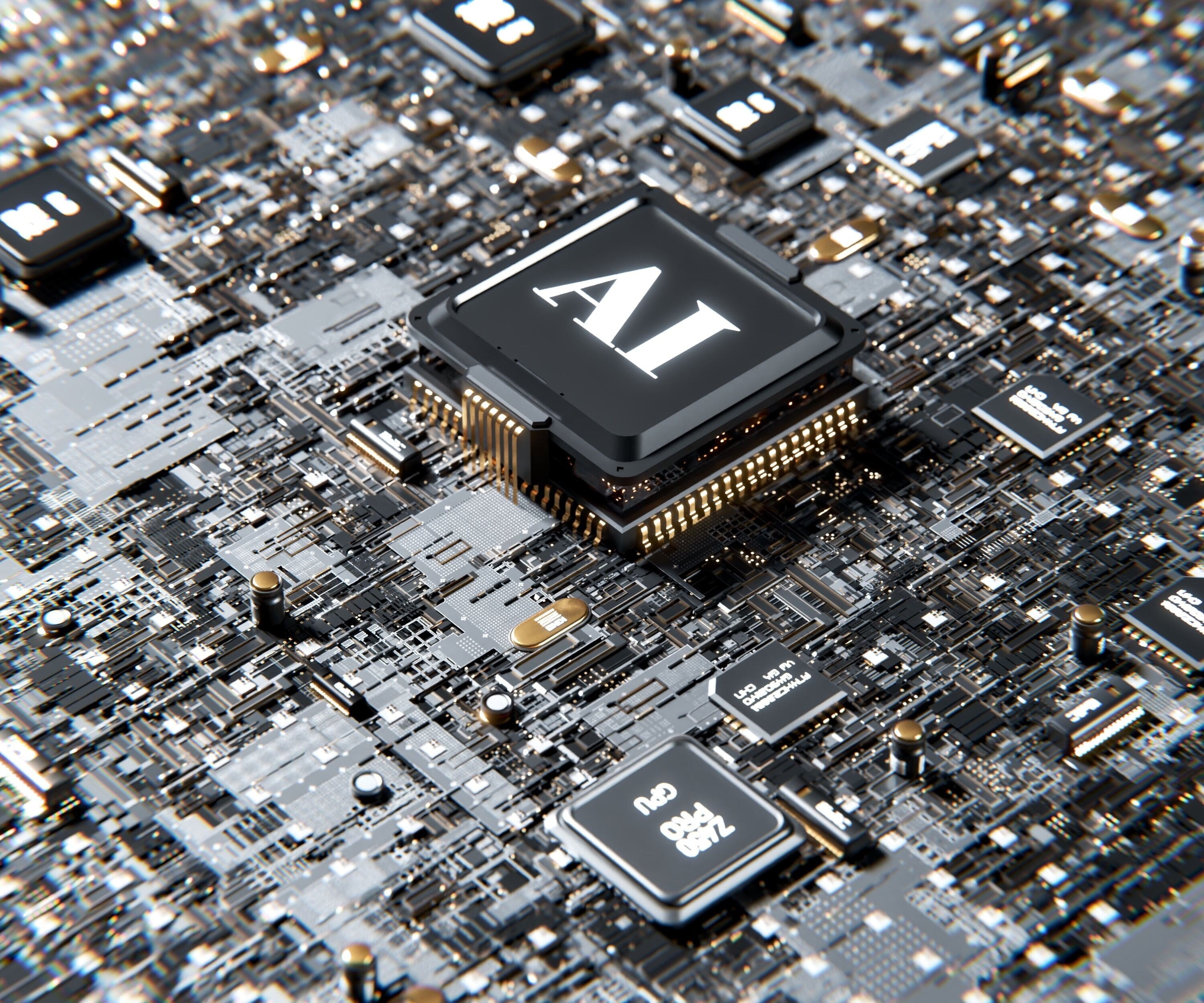
Responsible AI is what is called for now
Image: Photo by Igor Omilaev on Unsplash
Stay up to date:
Emerging Technologies
- Artificial Intelligence (AI) promises to be our era's most profound and consequential technological innovation.
- Businesses must rapidly adjust to an evolving regulatory environment and establish responsible AI governance frameworks aligned with their values and goals.
- The essence of responsible AI involves thoughtfully navigating numerous tradeoffs to deliver value while avoiding risks and protecting reputational capital.
Artificial intelligence (AI) promises to be our era's most profound and consequential technology innovation, evolving and advancing at breakneck speed.
With its latest advances, particularly generative AI (GenAI), it is bound to reshape entire industries, create new business models and impact every business function and our personal lives.
Like all groundbreaking technologies, AI comes with its own set of serious risks, including risks relating to data quality and privacy, the security and safety of confidential information, misleading outputs and, worse yet, deliberate misuse of the technology in misinformation campaigns and the hands of malicious actors.
Recognizing these challenges, governments worldwide have started establishing regulatory frameworks to ensure responsible development of AI-based technologies. The European Union has produced the EU AI Act, the first-ever dedicated law on AI. This aims to ensure the safe, legal use of AI and respect for fundamental rights within AI systems.
Looking ahead, businesses will need to set the foundations to rapidly adjust to an evolving regulatory environment, and they will need to establish responsible AI governance frameworks that are aligned with their values and business goals.
A huge responsibility falls on the private sector to ensure they have holistic and dynamic AI frameworks that help bridge the gap between innovation and responsible deployment.
So, where can businesses start?
How is the World Economic Forum creating guardrails for Artificial Intelligence?
Assess preparedness
Assessing preparedness is the first step in creating the optimal environment for AI governance. This means looking at AI holistically and answering some fundamental questions about the use of AI within an organization. For example, what is a company's vision of responsible AI based on its industry and regulatory environment? Given the organization's business model, how does this vision align with existing values, and what risks is AI likely to bring? Based on these considerations, organizations must evaluate their existing governance construct and decide whether their existing governance model is sufficient to deal with these risks.
Many organizations now consider the three lines of defence approach well suited to AI. This approach involves three layers of defence against risks:
1. Operational managers and employees who control and manage daily risks.
2. Specialized risk management and compliance functions for oversight and support.
3. Internal audits to provide independent assurance that risk management and governance processes are effective.
While this is a widely accepted approach, each organization must evaluate how it applies to its environment and determine the parameters and conditions suitable for each line of defence.
Preparedness also means looking at applicable legislation and identifying cost-effective solutions to meet compliance requirements.
The EU AI Act, for example, requires companies considered developers of high-risk AI to produce a conformity assessment and maintain thorough documentation to demonstrate their compliance with the regulation. Organizations that fall into this category will have to produce a Fundamental Rights Impact Assessment to evaluate the impact of their AI systems on individuals and their rights. This will involve producing records of programming and training methodologies, data sets used and measures taken for oversight and control.
At the same time, the expanded use of large language models (LLMs) that underly GenAI will create new security risks for organizations. LLMs have made bad actors more efficient and defenders have yet to catch up with these new techniques. As such, leveraging AI technology too early across multiple workflows may increase the risk exposure surface.
To get ahead of cyber risks, businesses will need to reassess their security approach and increasingly introduce red teaming — testing of cybersecurity systems by adopting an adversarial approach leveraging AI — as well as risk management frameworks and controls.
How is the World Economic Forum creating guardrails for Artificial Intelligence?
Establish a multidisciplinary team
AI is more than technology. It will reshape and transform our relationships and how we work together and alongside technology.
One of the greatest challenges for organizations will be to synchronize viewpoints and create a shared vocabulary around AI. Data scientists, for instance, might not fully comprehend AI's legal and ethical implications. In contrast, legal teams might have the legal and ethical background but not the competency to convert those considerations into computational calculations.
Creating a common language across all domains is a complex task that requires teams to cooperate when transcribing legal prerequisites into computational elements and vice versa. It is possible that data science could become a sought-after skill in legal teams, while engineers might be required to become well-versed in AI regulations enacted in different parts of the world.
This is a challenge that requires investment in cross-skilling across organizations. At some point, every organization will need to roll out a company-wide training programme to provide employees with foundational and cross-disciplinary AI skills. Additionally, teams will need to actively pursue specific upskilling in privacy-enhancing technology and develop more comprehensive technical solutions to comply with the legal requirements surrounding AI.
Prepare your data for AI
Data is the underlying foundation for the LLMs behind GenAI. These LLMs use significant amounts of data and create their own data based on the original inputs. The quality of the data fed into the models and the method by which that data is collected will factor into outputs. Ultimately, poor data will lead to poor outputs, undermining the value of AI or, worse yet, reinforcing biases and leading to incorrect conclusions or 'hallucinations.'
As the volume of data used by AI models continues to increase, some models will need to be retrained on specific subsets of data for higher accuracy. Overall, governance and management of data will become paramount for the effective deployment of AI.
Ultimately, preparing and training AI models will allow organizations to produce more precise forecasts, discover fresh data connections and generate better insights. From data quality, labelling and augmentation to data protection and governance, companies must enhance their data management processes across the entire data lifecycle. Advanced data analytics tools, stringent data quality checks and a culture of continuous data refinement and evaluation will all be integral to success.
Mind the bias
Data quality is also a priority for addressing biased and unfair practices in AI. This can occur when seemingly neutral programming reflects the biases of its developers, trainers or data inputs.
A biased algorithm presents a considerable problem. Discrimination driven by algorithmic models threatens to create an even greater disadvantage for underrepresented groups within the population. Studies have shown that such biases in AI-enabled recruitment can lead to discriminatory practices based on gender, race and personality traits, often stemming from limited raw data sets and the biases of algorithm designers.
The issue of bias sits at a crossroads of many disciplines and is particularly relevant in the context of privacy and equality laws. Addressing biases in AI requires technological and human interventions and a robust governance model.
Leverage technology, but don't rely solely on technology
In many instances, technology will come to the rescue and help companies address the challenges associated with transparency, privacy and data quality.
For example, zero-knowledge proofs — a type of cryptographic protocol — can allow verification of confidential attributes or facts using synthetic data without exposing the data itself. As synthetically produced data continues to improve, such solutions will increasingly help address the issues around data transparency.
Further, using privacy-enhancing technologies, such as encryption and differential privacy — a mathematical framework for ensuring the privacy of individuals in data sets — can help mitigate data leakage issues and create a more secure environment to experiment with data and build new solutions.
While technology is crucial, it is essential to remember that concerns around AI will require human intervention to navigate to positive outcomes. The role of humans in this context will help provide the necessary oversight, ethical decision-making and understanding of social and cultural contexts that machines cannot replicate. Companies will need human expertise to interpret AI outputs, ensure adherence to ethical standards and continuously refine AI systems to align with corporate and societal values.
Balance innovation with responsible AI
Ultimately, business leaders are responsible for fostering responsible innovation and ensuring that this groundbreaking technology is not slowed by regulation and applied in a way that offers a net benefit to businesses and societies at large.
How we deploy AI will speak to our values as companies. Laws aside, the essence of responsible AI involves thoughtfully navigating numerous tradeoffs to deliver value while avoiding risks and protecting reputational capital. If we do this transparently, involving our workforce and training our people so they come along in this journey, we will be playing a vital role in ensuring AI works for humanity.
Accept our marketing cookies to access this content.
These cookies are currently disabled in your browser.
Don't miss any update on this topic
Create a free account and access your personalized content collection with our latest publications and analyses.
License and Republishing
World Economic Forum articles may be republished in accordance with the Creative Commons Attribution-NonCommercial-NoDerivatives 4.0 International Public License, and in accordance with our Terms of Use.
The views expressed in this article are those of the author alone and not the World Economic Forum.
Related topics:
Forum Stories newsletter
Bringing you weekly curated insights and analysis on the global issues that matter.
More on Emerging TechnologiesSee all
Sandeep Chandna
June 20, 2025
Saket Singh
June 20, 2025
Joseph Fowler and Kadine James
June 20, 2025
Robert Falck
June 19, 2025
Marco Túlio Moraes
June 18, 2025
Sheikh Tanjeb Islam
June 17, 2025