Mastering AI quality for successful adoption of AI in manufacturing
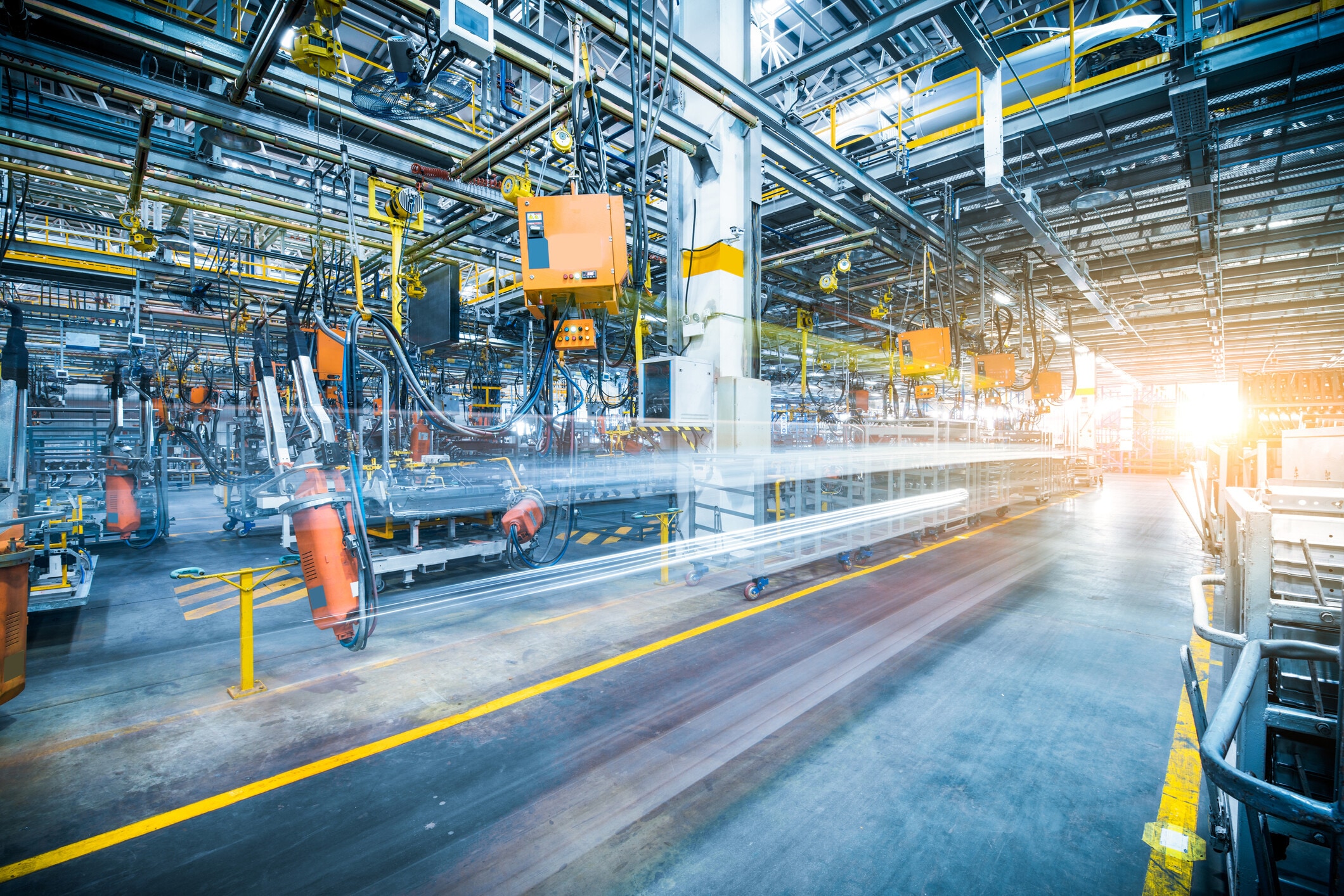
Managing AI quality enables industrial organizations to increase their productivity and sustainability. Image: iStockphoto.
Kyriakos Triantafyllidis
Head of Growth and Strategy, Centre for Advanced Manufacturing and Supply Chains, World Economic Forum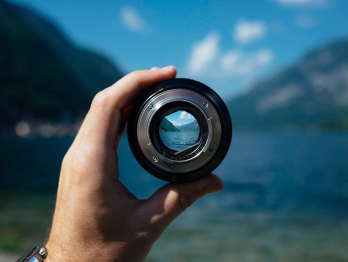
Get involved with our crowdsourced digital platform to deliver impact at scale
Stay up to date:
Artificial Intelligence
- AI is expected to transform manufacturing and supply chains, making it critical for businesses to stay competitive.
- Concerns about security, data protection, and regulatory uncertainties present serious challenges to AI adoption.
- Managing the quality of AI systems offers a systematic approach to evaluating risks and determining whether an AI system satisfies key requirements throughout its life cycle.
Artificial intelligence (AI) has emerged as the cornerstone of a reimagined manufacturing landscape, with 89% of executives across industries regarding AI as essential to achieve their growth objectives and aiming to implement it in their operations. Yet, concerns about security, data protection, regulatory compliance or performance issues present serious challenges to AI adoption in manufacturing and supply chains. AI also comes with intrinsic risks that may be exacerbated if not carefully considered. The lack of standardised processes to qualify AI products and assess risks when integrating and operating AI solutions results in a complex and often unclear path.
Effectively managing the quality of AI systems enables industrial organizations to overcome these challenges and harness the full potential of AI for greater productivity, flexibility, sustainability and workforce engagement while mitigating the associated risks.
What is AI quality and why is it important?
Quality has always been a key aspect of industrial operations. Quality assurance, essential in every stage of a manufactured product’s life cycle, parallels AI quality, which is a systematic approach to evaluating the degree to which an AI system meets specific requirements throughout its life cycle.
In assessing the quality of an AI system, six critical pillars are considered:
- Safety: focusing on potential harm to people or property.
- Security: evaluating cybersecurity risks and AI-specific threats.
- Legal: ensuring compliance with regulations and contractual obligations.
- Ethics: aligning the system with the company’s values and ethical principles affecting stakeholders.
- Performance: confirming the system’s effectiveness and accuracy.
- Sustainability: examining whether its development and operations have been conducted with environmental considerations.
Collectively, these elements determine the AI system's overall suitability and impact.
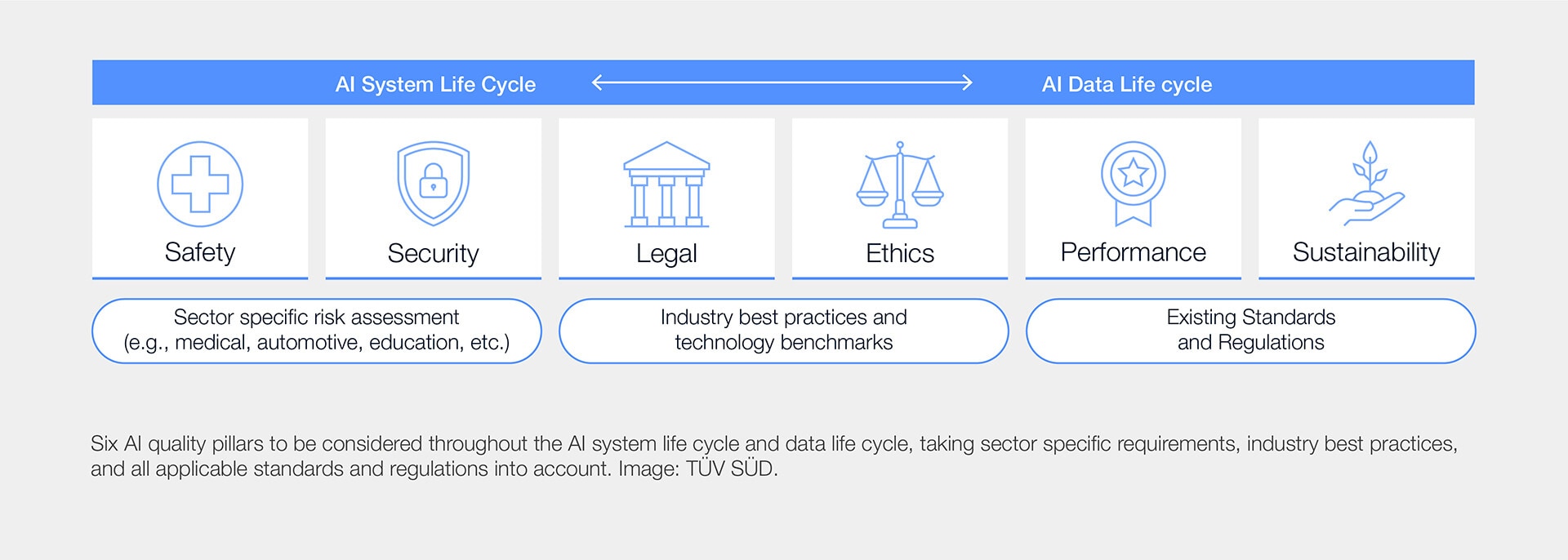
AI quality in manufacturing: where do we currently stand?
To understand the most common quality gaps when implementing AI in manufacturing, and collectively identifying solutions to address them for long-term successful, responsible, and sustainable outcomes, the World Economic Forum, in collaboration with TÜV SÜD, conducted an in-depth analysis of AI quality as part of the AI-Powered Industrial Operations Initiative. TÜV SÜD’s AI quality framework was leveraged to conduct an "AI Quality Readiness Analysis" – consisting of a risk analysis and a maturity profiling – for five manufacturing use cases. After identifying the relevant quality pillars, 60 risk characteristics were analysed, out of which 21 were highlighted based on their frequency of occurrence and maximum level of risk observed.
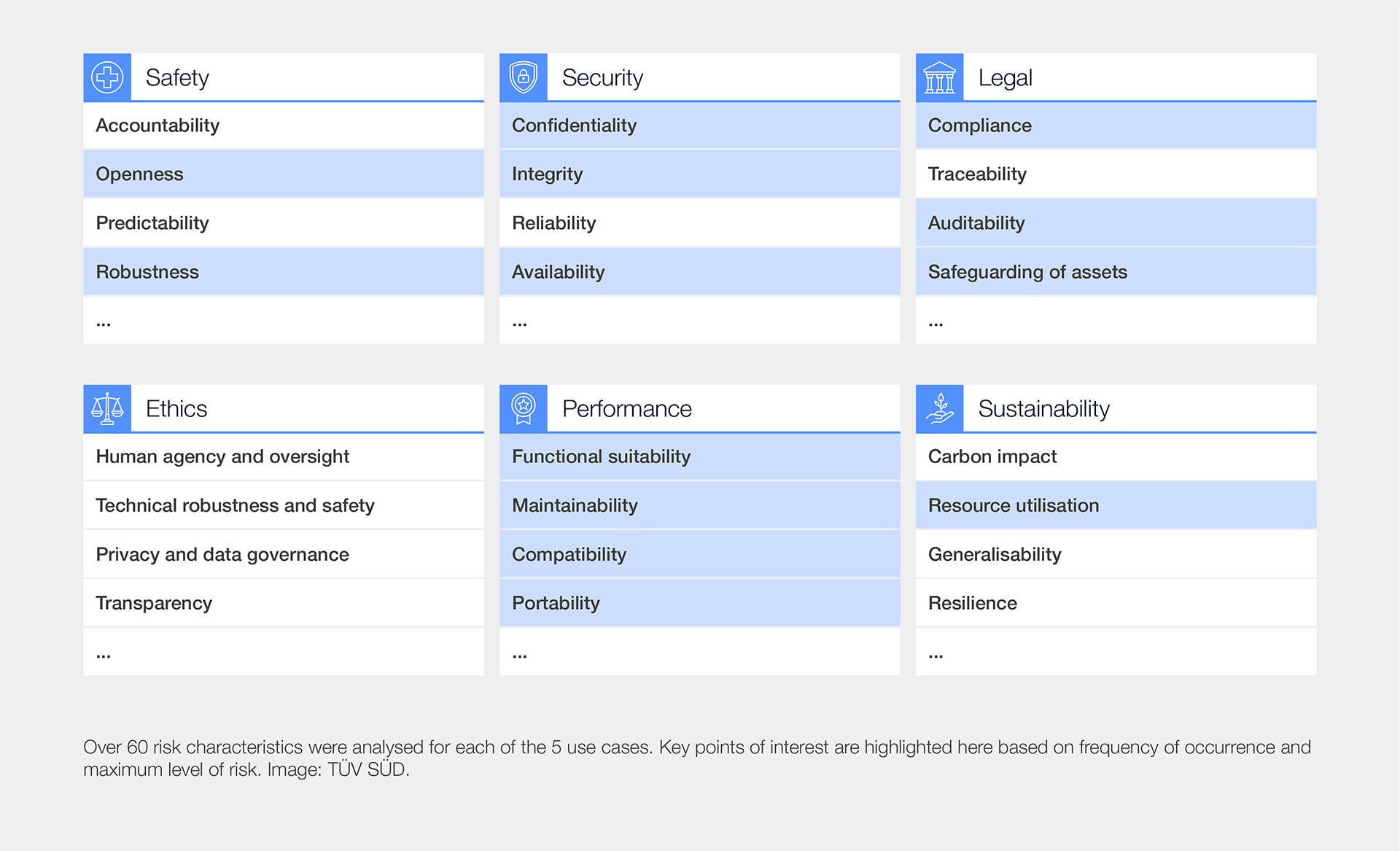
The assessments revealed that the highest percentage of risks were in the performance and security pillars. Risk criteria such as functional suitability, maintainability, and confidentiality were highlighted. Each risk characteristic represents a quality consideration that – if not met – introduces risk in the application of the AI system. For instance, ensuring functional suitability is paramount to guaranteeing that AI systems meet their intended objectives and perform effectively within their designated contexts. This is not only key for achieving reliable and accurate outcomes, but also for avoiding unintended consequences and maintaining user trust.
The security pillar reported comparatively higher levels of risk due to the sensitivity of the data processed by some of the assessed use cases. However, residual risks across all pillars were found to be generally well-managed, with effective risk mitigation strategies – such as robust security measures, proactive monitoring, and a culture of security awareness – bringing the maximum risk down to an acceptable level.
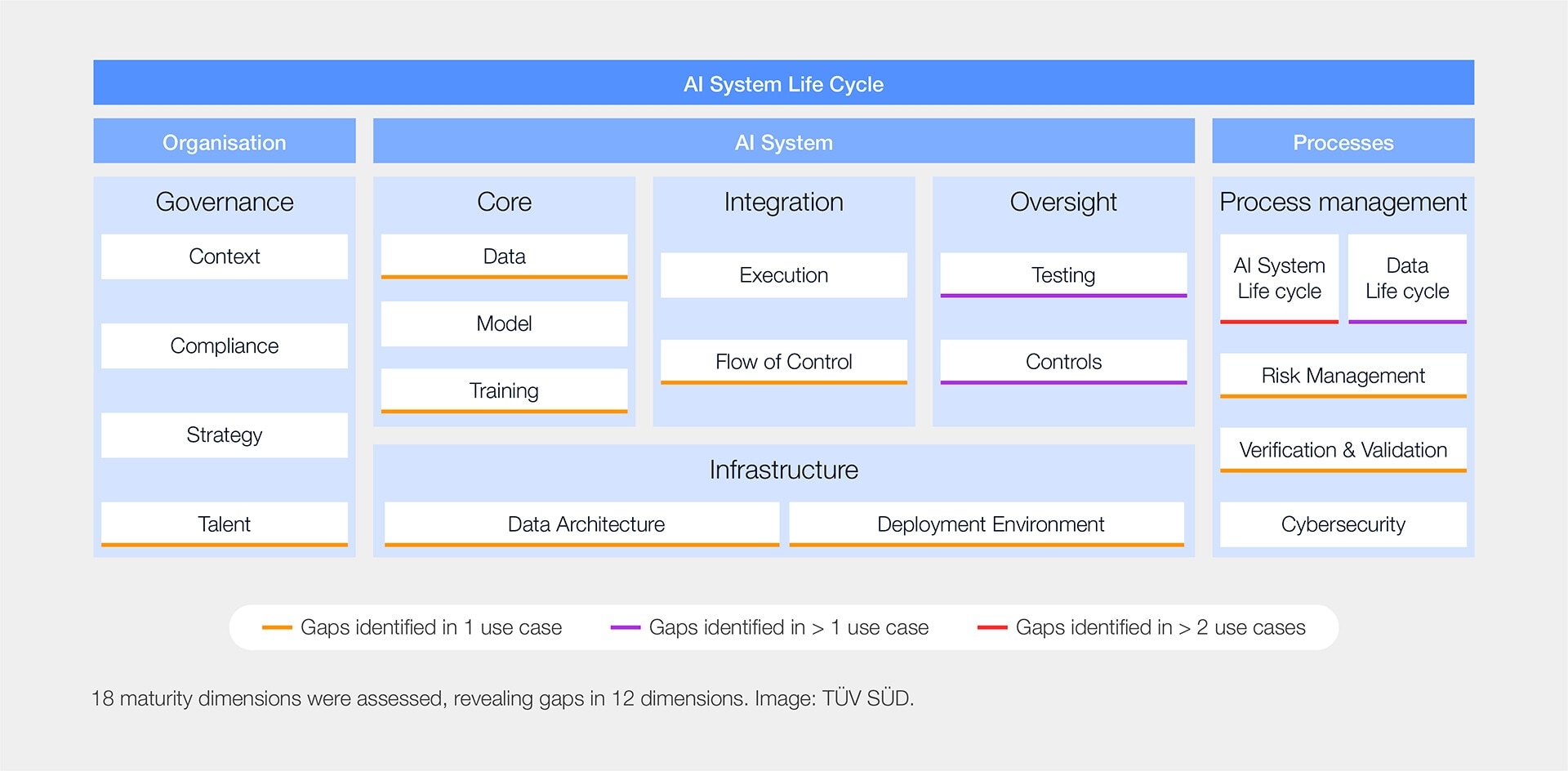
The aggregated results of the maturity profiling indicate well-managed organizational maturity across the assessed use cases. High maturity levels were reported in dimensions such as compliance and strategy, as well as in cybersecurity, with organizations implementing robust policies and processes for managing AI-related cybersecurity threats.
Some maturity gaps were identified in the oversight and process management dimensions. Organizations displayed a tendency to overlook certain aspects of the AI life cycle, particularly in outlining a robust decommissioning plan. Testing approaches did not always account for AI-specific risks, such as adversarial attacks. While several testing tools were adopted across the assessed use cases, these tools were not always systematically employed. Adopting a structured approach towards testing and controls is necessary for ensuring the overall reliability and robustness of AI applications and minimising the impact of any AI failures.
How is the World Economic Forum creating guardrails for Artificial Intelligence?
Consultation with our broader community of industry and technology experts led to the elicitation of best practice solutions to address the identified maturity gaps. Key considerations include upskilling talent through training and development programmes, aligning business strategy with technical products, and implementing a robust failure management system.
The community also highlighted the importance of viewing AI quality as a continuous process rather than a one-off assessment. Routine evaluations of AI systems, their associated risks and risk mitigation strategies, as well as ongoing documentation and updates, are essential to ensuring the quality of AI systems and are to be operationalised as part of a robust quality management system.
The way forward
To successfully capture the full potential from AI in manufacturing in driving productivity, agility, sustainability and workforce augmentation, and achieve long-lasting results, it is essential for organizations to recognise that AI solutions, distinct from conventional automation systems, come with their own set of inherent risks that require systematic management.
A robust approach for identifying and evaluating these risks in the development and deployment of AI systems enables manufacturers to effectively integrate AI into their industrial processes, for a smooth and efficient implementation and operation. This not only facilitates AI adoption and compliance with regulations but also cultivates trust in AI technology. Furthermore, ongoing assessment and adaptation helps organizations remain agile and responsive to new opportunities and challenges in this rapidly evolving field.
With a holistic AI quality strategy, AI's transformative potential can be responsibly and effectively leveraged, unlocking the next wave of value for businesses, workers, society, and the environment.
Don't miss any update on this topic
Create a free account and access your personalized content collection with our latest publications and analyses.
License and Republishing
World Economic Forum articles may be republished in accordance with the Creative Commons Attribution-NonCommercial-NoDerivatives 4.0 International Public License, and in accordance with our Terms of Use.
The views expressed in this article are those of the author alone and not the World Economic Forum.
The Agenda Weekly
A weekly update of the most important issues driving the global agenda
You can unsubscribe at any time using the link in our emails. For more details, review our privacy policy.
More on Emerging TechnologiesSee all
Bosun Tijani
September 18, 2024
Gareth Francis
September 18, 2024
Kate Whiting and Linda Lacina
September 16, 2024
Madeleine North
September 16, 2024
Minoo Rathnasabapathy and Nikolai Khlystov
September 16, 2024
Robin Pomeroy and Sophia Akram
September 16, 2024