Why economic forecasts are slow to change
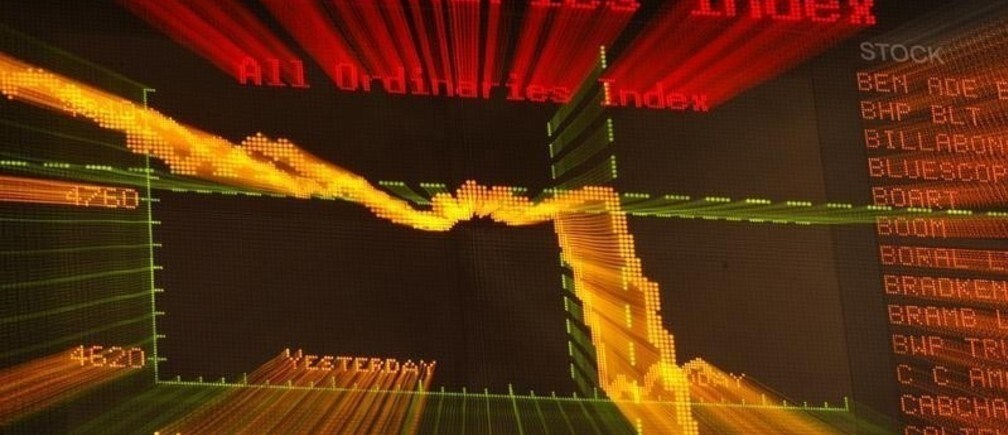
Forecasts of many macroeconomic variables tend to be serially correlated, which is inconsistent with rational expectations. This column presents new evidence from a two-decade panel of individual forecasts from 36 different nations. While there is evidence of sluggish behaviour in average forecasts, individual forecasts are revised quite often. Sticky information theory might not be an adequate description of the expectations formation of forecasters.
The 1970s saw the development of the concept of rational expectations. To be consistent with rational expectations, revisions to forecasts should be serially uncorrelated (Nordhaus 1987). If this is not the case, revisions to forecasts would have a predictable component, in turn implying that all available information was not being used fully.
Empirical work, however, suggests that forecasters deviate from this benchmark of full information rational expectations. Forecasts of real GDP – and indeed forecasts of many other macroeconomic variables – are characterised by sluggishness; that is, forecast revisions tend to be serially correlated.
Two major theories have been put forward to explain this finding.
- The first, due to Mankiw and Reis (2002), states that forecasters update their information sets – and hence their forecasts – infrequently because acquisition of information has fixed costs.
- In the second, developed by Woodford (2002) and Sims (2003), forecasters update their information sets continually, but receive imperfect signals about the true state of the economy.
Both theories of rational inattention – which have been dubbed ‘sticky information’ and ‘imperfect information’, respectively, in the literature – are consistent with the observed sluggish behaviour of forecasters (Coibion and Gorodnichenko 2012).
However, nearly all the empirical documentation of sluggish behaviour is based on the use of forecasts that are averaged across many individual forecasters. For example, the widely-used ‘consensus’ forecasts are the simple mean of many individual forecasts. Our recent work (Dovern et al. forthcoming), shows that the properties of average (or consensus) forecasts differ significantly from those of individual forecasters. While confirming the sluggish behaviour of average forecasts, we show using a large panel data set of individual forecast revisions that forecasts are updated frequently and that forecast revisions are serially correlated. Both features are inconsistent with the model of sticky information, which implies that forecasts are updated infrequently but fully (i.e., revised to match the full information rational expectation). Instead, our results support the idea that models of imperfect information are a useful tool to describe how forecasts are formed.
Sluggish behaviour of average forecasts
Our analysis is based on forecasts of annual GDP growth compiled by Consensus Economics, Inc. Each month, forecasters are asked to report their forecasts for annual GDP growth rates for the current and the next calendar year. Hence, for each target year, the data set contains a sequence of 24 forecasts from each panelist, starting in January of the year before the target year, and ending in the December of the target year. We have over 35,000 individual forecasts for target years between 1989 and 2011 from 36 different countries, of which about half are for 14 advanced economies. We can use this information to compute forecast revisions at the level of the individual forecasters, as well as the forecast revisions after taking the average of the individual forecasts (that is, we can compute the forecast revisions of the consensus).
Under the null of full information rational expectations, the sequence of revisions to forecasts for a particular event is uncorrelated at all leads and lags of revisions. Therefore, regressing forecast revisions on own lags yields zero coefficient estimates under the null. In particular, it can be shown that in a regression of the average forecast revision on its first lag, the regression coefficient translates directly into the degree of information rigidity both in the sticky information framework and in the imperfect information framework (Coibion forthcoming).
We find strong and consistent evidence of information rigidities in consensus forecasts (Figure 1). The current forecast revision is highly and positively correlated with its first lag for all country groups. The coefficients on the lagged revisions are statistically significant in all cases. The extent of information rigidities appears to be broadly similar in forecasts for advanced and emerging economies. Under the assumptions of the sticky information model, these estimates imply that forecasts are updated about every six months.
Figure 1. Comparing sluggishness in consensus and individual forecasts
Notes: The bars represent the estimated coefficients from regressions on forecast revisions on their first lag. A higher coefficient indicates greater sluggishness in forecast revisions (that is, lower frequency of updating forecasts). See Dovern et al. (forthcoming) for details.
Rapid revision of individual forecasts
When we use the same framework to analyse information rigidities in individual forecast revisions, the results are quite different. While we still get a positive and statistically significant coefficient on the first lag, the magnitude is much smaller than that of the consensus. Thus, the degree of sluggishness in forecasts is much smaller. Instead of the estimate of an update every six months implied by use of consensus forecasts, our use of individual forecasts suggests an update about every two months.1 This suggests that the process of averaging forecasts introduces additional stickiness that is not present at the individual level.
The distribution of the information rigidity coefficients across countries substantiates the view that the process of aggregation introduces sluggishness. As shown in Figure 2, for both advanced and emerging economies the use of average forecasts suggests considerable sluggishness; the coefficients cluster around 0.5 and 0.6. But when individual data is used, the coefficients are much lower, clustering around 0.2 for both advanced and emerging economies.
Figure 2. Distribution of information rigidity coefficients across countries
Notes: These are kernel density estimates for the distribution of coefficients from country-by-country regressions of forecast revisions on the past revision. The estimated coefficients show the degree of information rigidity in each country: a larger number indicates greater sluggishness in revising forecasts.
Frequency of updating forecasts
An advantage of our use of individual-level forecasts is that we can directly measure the frequency of updates. Again, we find that individual forecasts are revised quite often. The share of forecasters who update their forecasts at least once in the three months prior to a given forecast horizon ranges between 0.8 and 0.9 over the entire forecast horizon (Figure 3). These estimates are close to those obtained by Andrade and Le Bihan (2013) for the European Survey of Professional Forecasters, and suggest a smaller role of sticky information in explaining the overall degree of information rigidity in growth forecasts.
Figure 3. Average fraction of revised individual forecasts by forecast horizon
Notes: The upper and lower bounds refer to the extreme country-specific estimates within each country group. The black line refers to the average across all individual forecasts for countries from a particular country group.
Bottom line
Using individual professional forecasts of economic growth in advanced and emerging economies, we find strong evidence against the usefulness of the sticky information model for describing the formation of expectations about the future state of the economy. The degree of forecasters’ inattentiveness is much less pronounced in individual data than in average data, which previous studies tended to use. Direct estimation of the updating frequency for individual forecasts also suggests only a minor role for sticky information in explaining the overall degree of information rigidity in economic forecasts. This does not imply that the sticky information theory may not be an adequate description of the expectation formation process for other agents in the economy (for example, households who devote fewer resources to information collection and do not update their forecasts as frequently as professional forecasters do). In short, professional forecasters are not as inattentive as average forecasts might suggest, and the jury is still out on how other economic agents form their expectations.
References
Andrade, P and H Le Bihan (2013), “Inattentive professional forecasters”, Journal of Monetary Economics, 60(8), 967–998.
Coibion, O (forthcoming), “Comments on Dovern, Fritsche, Loungani and Tamarisa”, International Journal of Forecasting.
Coibion, O, and Y Gorodnichenko (2010), “Information rigidity and the expectations formation process: a simple framework and new facts”, NBER working papers 16537, National Bureau of Economic Research, Inc.
Coibion, O, and Y Gorodnichenko (2012), “What can survey forecasts tell us about information rigidities?” Journal of Political Economy, 120(1), 116–159.
Dovern, J, U Fritsche, P Loungani and N Tamirisa (forthcoming), “Information rigidities: Comparing average and individual forecasts for a large international panel”, International Journal of Forecasting, (see also Working Paper version)
Mankiw, G and R Reis (2002), “Sticky information versus sticky prices: a proposal to replace the new Keynesian Phillips curve”, Quarterly Journal of Economics, 117(4), 1295–1328.
Nordhaus, W (1987), “Forecasting efficiency: concepts and applications”, The Review of Economics and Statistics, 69, 667–674.
Sims, C (2003), “Implications of rational inattention”, Journal of Monetary Economics, 50(3), 665–690.
Woodford, M (2002), “Imperfect common knowledge and the effects of monetary policy”, In P. Aghion, R. Frydman, J. Stiglitz, & M. Woodford (Eds.), Knowledge, information, and expectations in modern macroeconomics: in honor of Edmund S. Phelps, Princeton University Press.
Footnote
1 When estimated on individual forecasts, the coefficient on lag revisions should be interpreted as a general measure of the degree of forecast smoothing, which reflects behavioural features or deviations from efficiency.
Published in collaboration with VoxEU
Authors: Jonas Dovern is an assistant professor of macroeconomics at Heidelberg University. Ulrich Fritsche is a Professor of Economics at Hamburg University. Prakash Loungani is a Senior resource manager and advisor in the IMF’s Research Department. Natalia Tamirisa is the Assistant to the Director of the Research Department at the International Monetary Fund.
Image: The September 17 and 18 curves are seen on the board at the Australian Securities Exchange (ASX) in Sydney September 18, 2008. REUTERS/Daniel Munoz
Don't miss any update on this topic
Create a free account and access your personalized content collection with our latest publications and analyses.
License and Republishing
World Economic Forum articles may be republished in accordance with the Creative Commons Attribution-NonCommercial-NoDerivatives 4.0 International Public License, and in accordance with our Terms of Use.
The views expressed in this article are those of the author alone and not the World Economic Forum.
Stay up to date:
Financial and Monetary Systems
Forum Stories newsletter
Bringing you weekly curated insights and analysis on the global issues that matter.
More on Financial and Monetary SystemsSee all
Emmalina Glinskis and Daniel Murphy
January 8, 2025