Apps know where you live, where you work, where you shop. So what does trust mean today?
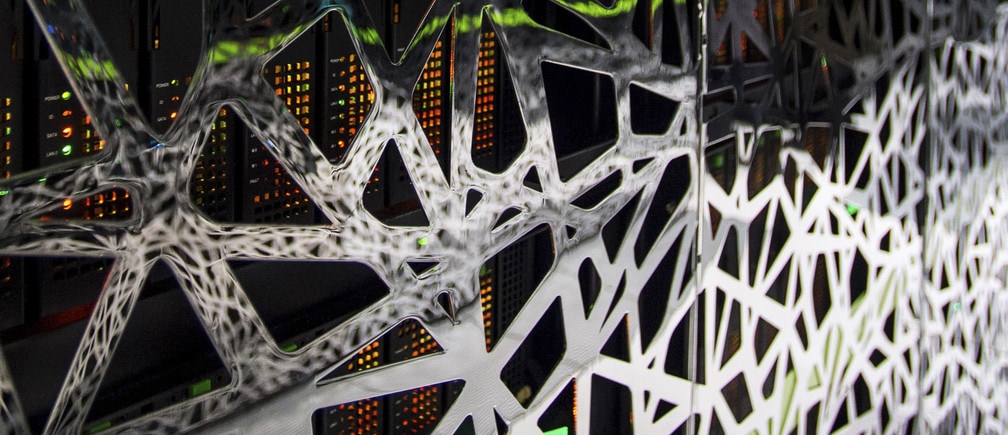
"While humans can handle around a dozen variables at a time, algorithms can handle thousands." Image: REUTERS/Fred Lancelot
It is no secret that data from just about everything is being captured, stored, analysed, shared and analysed again.
According to a recent Cisco report, the data generated by internet-of-everything devices will reach over 500 ZB (zettabytes) per year by 2019. Technology advances have made it easier to acquire, manage and extract value from this explosive volume of data – of all types and origins, from business systems, social media, wearable technologies, web clicks, and mobile apps usage, to the main emerging contributor – the growing number of ubiquitous sensors in IoT devices.
As an industry, we have learned a lot about large-scale data challenges from building things like internet search and large-scale e-commerce businesses, and from combating cybercrime.
One of the things we have learned is that we need to apply machine learning techniques to very large and evolving data sets in order to get the real value from the data. Innovative technology solutions that do this well are creating new data-driven business models.
Indeed, we have seen a crop of next-generation companies built around the use of data, such as Uber, LinkedIn, Airbnb and Concur. We know that real value lies with applications that derive processes and insights from digitization of anything and everything. And we need machines for this, since it is impossible for humans to extract insights from the vast amount of data available by just applying brute force.
While humans can handle around a dozen variables at a time, even the most basic machine learning algorithms can handle thousands – and this is not even talking about volumes and rates of data.
We are already seeing benefits from sophisticated and evolving intelligent systems – from new products and services, to changes in existing applications. We are now seeing bots making their entrance with use cases way beyond what we first imagined. For example, financial reports are being written by journalism-bots, and the Associated Press is using AI bots to turn quarterly earnings statements into news articles.
Even a space we normally think of as far from the centre of technology, farming, is gaining value from AI. Through deep learning, farmers are identifying disease in plants. By uploading very large sample sets of photos of diseased and healthy plants, a machine learning algorithm has enabled a computer to learn and distinguish the health of plants. It can correctly identify 14 crop species and 26 diseases (or absence thereof) 99.35% of the time from the new images uploaded; and it continues learning from each new image it receives. This is significant, because each time a farmer misdiagnoses why a plant is not doing well, the farmer may end up wasting money and time on the wrong pesticides or herbicides. In the future, AI could help farmers quickly and accurately pinpoint the problem – ultimately doing this in real-time and automatically on the field equipment.
In the USA, farmers use millions of pounds of herbicide with a “spray and pray” approach. Now there is a machine-learning-powered tractor, the LettuceBot, which takes 5,000 pictures every minute of young lettuce plants. The algorithms used by the LettuceBot can discern a lettuce sprout from a weed, and can spray individual weeds with herbicide with an accuracy of a quarter inch – reducing the overall use of chemicals by 90%.
On a more personal side, consumer apps on our mobile devices have changed how we navigate through a city with Google Maps or Waze; how we socialize through apps like WeChat, Facebook, and Twitter; and how we shop online and on the go. What many consumers don’t know, is that when they use these apps, they are very innocently giving away incredible amounts of personal information – both directly and in what is inferred from the data. Many applications are tracking where you are all the time, even when you are not using the app. Even casual analysis of this data can determine where you live, where you work, where you shop, and even what you are shopping for.
A May 2015 Harvard Business Review article revealed that 75% of the people surveyed did not know that they were giving out their location information and 86% did not know companies were collecting their web-surfing history.
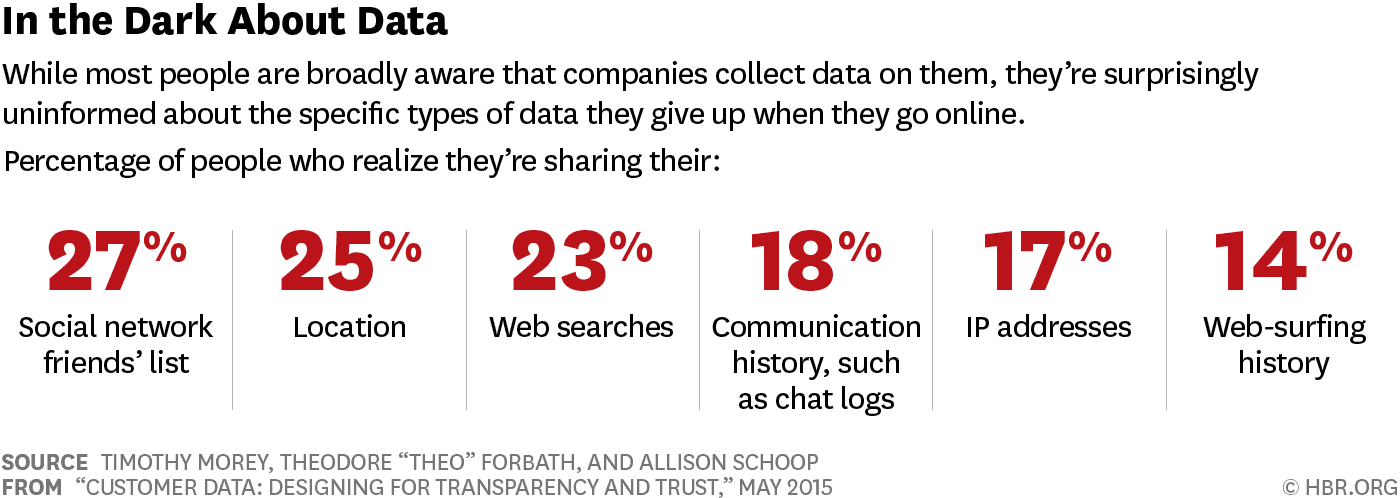
Companies who own these apps are using the data for legitimate reasons – they develop algorithms to gain insights from the collective user data to build better products and services for their users. Many companies have looked closely at this, and it is the case that consumers are willing to share their data if they get value in return. On top of that, few consumers are willing to give up the use of these technologies in exchange for greater privacy. Can you think of anyone who would rather go back to using paper maps instead of using GPS-enabled apps like Google Maps?
But there is more. Some companies collect the data, do the analytics, learn about you, and then sell the data to someone else. So, who owns the data in those cases? And what are we doing to prevent abuse of use?
We are in uncharted territories – new technology capabilities, new business models, and infinite possibilities. Whether you are in the private or public sector, the value from the insights gained is obvious. Those who harvest insights from big data and turn them into better products, services or experiences will be leaders in the digital economy. But this value comes with the responsibility of respecting people’s privacy and security, and understanding that abuse or carelessness with their data can affect real people’s lives.
We are early in this journey, and we don’t have much in terms of rules or guidelines. Companies like Facebook pushed the boundaries of privacy and personal data use – believing that people will embrace transparency. But even they don’t have perfect loyalty and trust from their customers – two essential qualities for survival in any business.
It’s clear that leadership in nearly every business will be dependent on leveraging data in ways never envisioned even a few years ago. Forward-looking companies who chose to lead this change will gain a competitive advantage and a point of differentiation for their brand. But doing so opens new challenges in making the most of this opportunity while at the same time safeguarding privacy and security. That leads to this question: how do we design trust into intelligent applications?
The answer starts by agreeing that across all industries, we need to align the interests of companies and their customers, and ensure that both parties benefit from personal data collection. I think there are things companies can do to ensure that we start to strike this balance between the value extracted from data with data privacy and security. They follow four themes:
Ethics
Companies who use people’s data must earn customer trust, and as a data practitioner they must instill an ethical culture company-wide regarding data usage. That is for all types of data captured, with clear policies on when and how it is used directly, in aggregate by the company or by others. They should develop a code of conduct for data, shift from a compliance mindset to stewardship, where “data stewards” represent the customer’s voice. To do this, we can draw on the best practices seen in the Data Science Association’s Data Science Code of Professional Conduct.
Protection
Security of the computing environment is everyone’s job, not just the IT department’s. It goes back to the culture of the organization to be vigilant and sensitive to data governance, data privacy and security policies. Attacks on the enterprise are growing more sophisticated every day, both in technology and in social engineering. We must design data privacy and security early into the development process, and give consumers control on how much they can share.
Value
Companies must focus on the value they give back to the customers in exchange for access to their data, and hence create a virtuous cycle of trust. This starts with an approach whereby we collect only the data that is needed to run the products and the business, improve a customer experience, or create new opportunities for users to benefit.
Transparency
Companies need to be transparent with customers as to what data is being collected, why and how it will be used. Transparency is a must as policy-makers begin to proactively monitor and audit the usage of data, and as legislation takes shape on how and for what purpose businesses can use the data. It is also up to companies to help consumers understand their choices, and the risks associated with the digital footprint they are leaving behind.
Beyond actions data practitioners can take to address data privacy and security, there is the whole topic of governance and policy on the use of data with technology innovations using AI. Policy-makers have the challenge of creating a balanced regulatory environment for technological advancements – one that ensures stakeholder trust and security while encouraging innovation.
The World Economic Forum’s Intelligent Assets- Unlocking the Circular Economy Potential report published earlier this year outlines actions policy-makers in the public and private sector can do to mitigate the risk as we transition into these uncharted territories. The answer lies in taking action across-industries, educating and increasing awareness for consumers, supporting investment schemes, and creating fiscal and regulatory frameworks to ensure secure enabling environments for the development of these intelligent applications.
While the phrase is borrowed from Winston Churchill, the message applies: with the great power of big data, comes great responsibility. Enterprises and government alike must earn the trust of consumers for them to share their data. This will be determined by whether public and private stakeholders manage to successfully design and implement the enabling condition listed above. Extracting value from data while improving people’s lives and safeguarding personal privacy and security are all key to success.
Industry leaders must guide the way in the development of more stringent data privacy policies that provide transparency on how data is being used and not abused.
Don't miss any update on this topic
Create a free account and access your personalized content collection with our latest publications and analyses.
License and Republishing
World Economic Forum articles may be republished in accordance with the Creative Commons Attribution-NonCommercial-NoDerivatives 4.0 International Public License, and in accordance with our Terms of Use.
The views expressed in this article are those of the author alone and not the World Economic Forum.
Stay up to date:
Data Science
Related topics:
The Agenda Weekly
A weekly update of the most important issues driving the global agenda
You can unsubscribe at any time using the link in our emails. For more details, review our privacy policy.
More on Emerging TechnologiesSee all
David Elliott
November 25, 2024