2016 might seem like the year of AI, but we could be getting ahead of ourselves
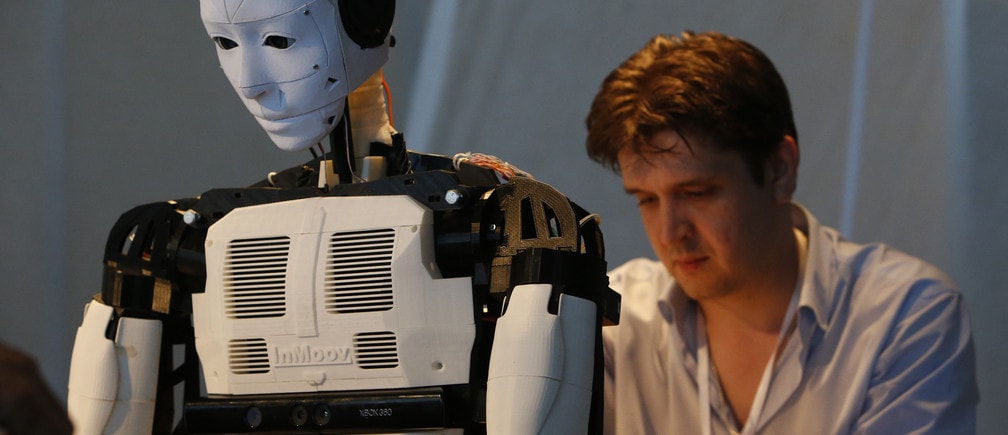
AI is still highly dependent on humans, argues PV Kannan
Image: REUTERS/Sergei Karpukhin
Stay up to date:
Innovation
This year we’ve seen a spate of announcements from Google to Facebook to IBM and scores of smaller companies, announcing Artificial Intelligence, or “AI” initiatives. Fueled by technology advancements (big data processing power), IBM Watson’s marketing and sales engine and Facebook’s marketing engine, media are latching onto AI as the next big technology trend. At first, it seemed like 2016 was the year that that this technology finally arrived, but the reality is that today’s AI solutions are still critically dependent on humans.
AI enables computers to mimic human learning, understand the user and perform tasks that normally require human intelligence (e.g. visual perception, speech recognition, decision-making, and translation between languages). The good news is that with this rising interest, more companies have demonstrated a willingness to invest in this technology. The bad news is that the hype around AI has led to misguided expectations about what’s actually possible, especially in the realm of customer engagement, which is the business of the company I run, [24]7.
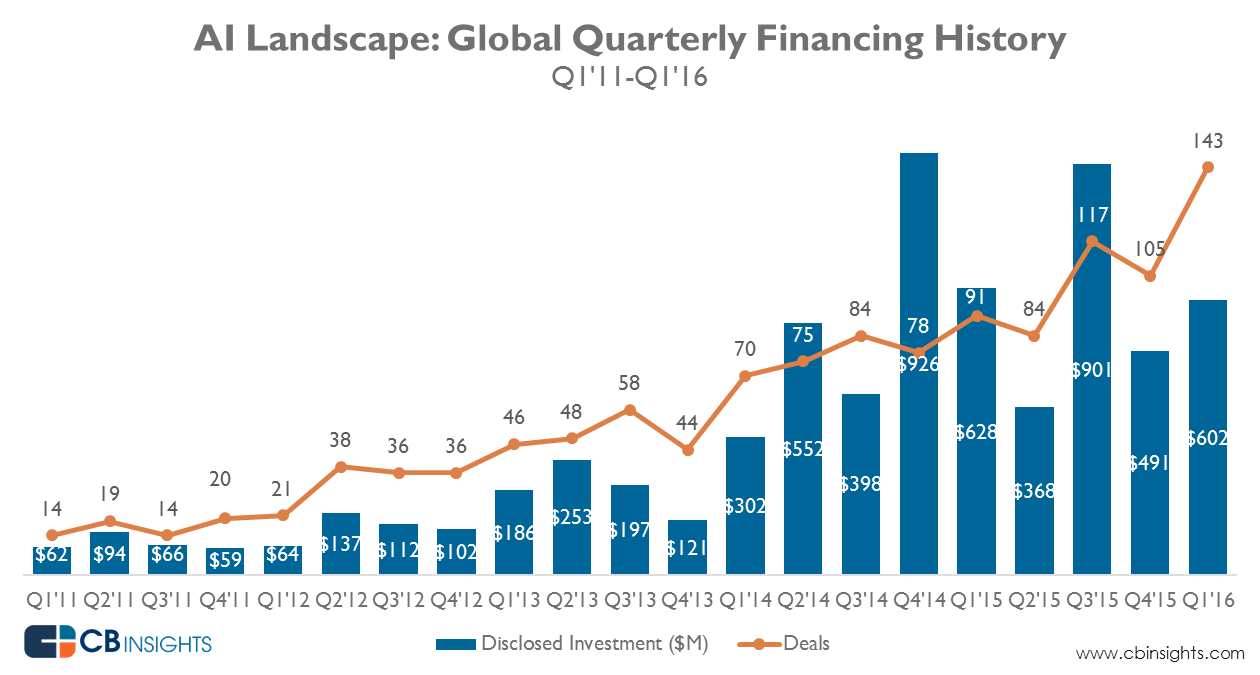
AI is great for things such as deciding whether to increase the credit limit on a card, where the steps are clear and the text of the interaction can be culled from actual human conversations that took place previously with call center operators. Companies have histories of these interactions and can cherry pick the ones that had very high customer satisfaction scores. That way the system keeps getting better based on interactions. Every time something in an automated conversation goes wrong, it gets routed to a human who teaches the system a little bit more. That’s how learning happens over time.
The amount of human labor behind it remains considerable, and the more business leaders understand how these sorts of systems work, the more they can use them with confidence. Unlike with personal assistants such as Google, Siri, Echo or Cortana, where there isn’t a big downside if the system misunderstands or returns the wrong answer, it’s critical for businesses to get this right. No business can afford the customer churn that comes from providing bad or inaccurate information to consumers.
How Machine Learning Works
Machine learning is a type of AI in which computers can learn without being explicitly programmed. It focuses on the development of programs that can teach themselves to grow and change when exposed to new data. It often comes into play when there’s a need to process huge amounts of data that are beyond the scope of humans to process on their own. That data can be used to learn about human patterns and behavior. For example, in Natural Language Processing (NLP), the system is learning about language. It may need to determine, for example, the difference between “available balance” and “account balance.”
There are two main human-assisted machine learning techniques: supervised and semi-supervised. Supervised learning, the most common technique, uses collaborative tagging by humans so that models can identify consumer intent. With semi-supervised learning, some data is processed by the system and some is manually tagged. Supervised or semi-supervised techniques perform well for the majority of enterprise applications with complex business requirements.
Unsupervised learning, by contrast, is much harder. It is best thought of as a continuum between (a) the entire system being one gigantic, autonomous, self-learning machine and (b) solving certain problems within a much larger system that also involves humans and supervised learning techniques. For many enterprise solutions we are very close to (b). For personal assistants like Siri, we are a little closer to (a), but even in such applications true autonomous AI is still quite far away. Imagine the amount of human intervention that will need to happen on the back-end, or how many special cases must be handled by editors or trainers in teaching the system.
For informational tasks (e.g. answering the question, “What’s the highest rated smartphone?”), a machine could automatically assemble answers from agent responses, social responses, and other knowledge sources (the way IBM’s Watson figured out how to win at Jeopardy). Data needs to be consolidated into a consistent format, and supervised learning is required to bootstrap the models. But over time, autonomous learning can refine answers or create new ones.
For transactional tasks (e.g. a consumer making a purchase), it is difficult for a machine to generate logic through autonomous learning, and therefore machines are much more reliant on humans. In the medium term, the approach is to automatically generate code from concepts and actions defined by developers. Systems could someday automate the conversation, enabling the user to execute the transaction.
There’s a spectrum of AI capabilities that differ in their use of machine learning, but we’re in the inflated expectations stage of the AI hype cycle. In most near-to-mid-term scenarios, businesses will be best served by supervised or semi-supervised machine learning.
Don't miss any update on this topic
Create a free account and access your personalized content collection with our latest publications and analyses.
License and Republishing
World Economic Forum articles may be republished in accordance with the Creative Commons Attribution-NonCommercial-NoDerivatives 4.0 International Public License, and in accordance with our Terms of Use.
The views expressed in this article are those of the author alone and not the World Economic Forum.
Related topics:
Forum Stories newsletter
Bringing you weekly curated insights and analysis on the global issues that matter.
More on Emerging TechnologiesSee all
Kelly Ommundsen
July 4, 2025
Michael Donatti and Laura Fisher
July 3, 2025
Benjamin Hertz-Shargel
July 1, 2025
Ravi Kumar S. and Rohan Murty
July 1, 2025
Abayomi Olusunle
July 1, 2025
Stacy Greiner
July 1, 2025