Are businesses ready for AI? This is what more than 3,000 executives think
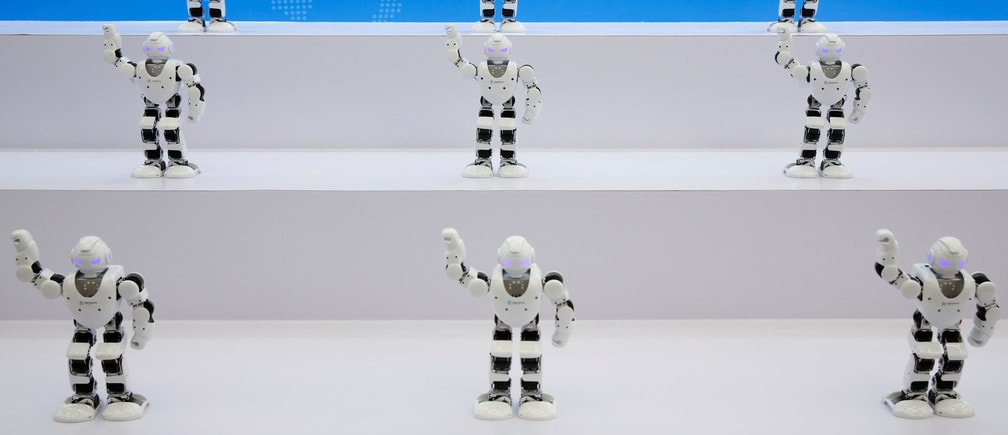
Today, businesses expect AI to lead to new activities and sources of employment.
Image: REUTERS/Thomas Peter
Stay up to date:
Emerging Technologies
Artificial intelligence has become one of the biggest technological developments in business in recent years, but the field is still largely shrouded in uncertainty. While expectations run sky-high, what are businesses actually doing now? A new report by BCG and MIT Sloan Management Review aims to demystify AI in business and take stock of current industry adoption. The report is based on a global survey of more than 3,000 executives and in-depth interviews with more than 30 technology experts and executives. Its goal is to present a realistic baseline that allows companies to compare their AI efforts and ambitions and to provide guidance for things to come.
The gap between ambition and execution is large at most companies. Only about one in five companies has incorporated AI into some offerings or processes. Only one in 20 has extensively incorporated AI into offerings or processes. The research also reveals large gaps between leaders—companies that understand and have adopted AI—and laggards in every industry. One sizable difference is their understanding of, and approach to, AI data and training. For many, the differences will likely magnify over the next five years, but nearly all companies still have the ability to establish competitive advantage and shape value creation from AI in their sectors.
High expectations
The high expectations for AI cross geographies, industries, and companies, regardless of size. While most executives have not yet seen substantial effects from AI on their offerings and processes, they clearly expect to in the next five years. (See Exhibit 1.) Most organizations foresee sizable effects on IT, operations and manufacturing, supply chain management, and customer-facing activities. Business-process-outsourcing companies, for example, expect many of the jobs that moved to low-labor-cost countries in recent years to be automated. However, they also expect AI to lead to new activities and sources of employment. Executives at industrial companies expect the largest effect in operations and manufacturing. In connection with its new A350 program, for example, Airbus is using AI to speed and improve production. The company has combined data from past production programs, continuing input from the A350 program, fuzzy matching, and a self-learning algorithm to identify patterns in production problems. In some areas, the system matches about 70% of seemingly unrelated production disruptions to solutions used previously—in near-real time.
Ambition and execution
Expectations aside, the gap between ambition and execution is large at most companies. Three-quarters of executives believe that AI will enable their companies to move into new businesses. And almost 85% believe AI will allow their companies to obtain or sustain a competitive advantage. But while more than 60% of respondents said that a strategy for AI is urgent for their organizations, only half of those said that their organizations have a strategy in place. The largest companies (those with more than 100,000 employees) are the most likely to have an AI strategy, but only half have one.
There are also large gaps between leaders and laggards. Among leaders, three-quarters have identified business cases for AI. About 80% say that senior leaders are onboard. The biggest hurdles at these companies are hiring and developing talent and establishing priorities for AI investments; they are also starting to worry about security issues related to AI. Laggards, on the other hand, have not identified business cases. More than 50% report that their senior leaders are generally not involved in AI, and most have yet to encounter the difficulties of sourcing AI talent.
The differences in adoption can be striking, particularly within the same industry. Ping An Insurance Company of China, one of that country’s largest insurers, employs 110 data scientists and has launched about 30 CEO-sponsored AI initiatives that support, in part, its vision “that technology will be the key driver to deliver top-line growth for the company in the years to come,” says the company’s chief innovation officer, Jonathan Larsen. Elsewhere in the insurance industry, AI initiatives are at the other end of the spectrum, limited to such efforts as “experimenting with chatbots,” as a senior executive at a large Western insurer described his company’s AI program.
Data, training and algorithms
One of the most telling differences between leaders and laggards is their understanding of the importance of data, training, and algorithms. AI algorithms are not natively “intelligent.” AI starts with “naked” algorithms that become intelligent only upon being trained on large amounts of data and, for most business applications, large amounts of company-specific data. Successful training requires fully understanding this process and the role of data, which is far more significant than it is in big data and advanced analytics applications. Success also depends on having well-developed systems that can pull together relevant training and continue to integrate findings from later data collected over time. Data collection and preparation are often the most time-consuming activities in developing AI applications.
Thus, even if the organization owns the data it needs, fragmentation across multiple systems can hinder the process of training AI algorithms. Agus Sudjianto, executive vice president of corporate model risk at Wells Fargo, put it this way: “A big component of what we do is dealing with unstructured data, such as text mining, and analyzing enormous quantities of transaction data, looking at patterns. We work on continuously improving our customer experience as well as decision making in terms of customer prospecting, credit approval, and financial crime detection. In all these fields, there are significant opportunities to apply AI, but in a very large organization, data is often fragmented. This is the core issue of the large corporation—dealing with data strategically.” Less than half of our survey respondents said that their organization understands the data needs of algorithms or the processes required to train algorithms.
Make versus buy
The need to train AI algorithms with appropriate data has wide-ranging implications for the traditional make-versus-buy decision that companies face with new-technology investments. Generating value from AI is more complex than simply making or buying AI for a business process. Training AI algorithms involves a variety of skills, including understanding how to build algorithms, how to collect and integrate the relevant data for training purposes, and how to supervise the training of the algorithm.
The CIO of a large pharma company described the products and services that AI vendors provide as “very young children.” The AI tech suppliers “require us to give them tons of information to allow them to learn,” he said. “The amount of effort it takes to get the AI-based service to age 17 or 18 or 21 does not appear worth it yet. We believe the juice is not worth the squeeze.” Using AI for competitive advantage requires companies to build up their internal skills.
Management challenges
AI requires more than data mastery. Companies also face many managerial challenges common to other technology-driven transformations. These include vision and leadership, openness and the ability to change, long-term thinking, close alignment between business and technology strategy, and effective collaboration. Companies also face AI-specific challenges.
Most basic—and most important—is developing an intuitive understanding of AI. J.D. Elliott, director of enterprise data management at TIAA, a Fortune 100 financial services organization with nearly $1 trillion in assets under management, said, “I don’t think that every frontline manager needs to understand the difference between deep and shallow learning within a neural network. But I think a basic understanding that—through the use of analytics and by leveraging data—we do have techniques that will produce better and more accurate results and decisions than gut instinct is important.”
A second challenge is organizing for AI. Adopting AI broadly will likely place a premium on soft skills and organizational flexibility. There are different models—such as centralized, distributed, and hybrid—but ultimately, a hybrid model emphasizing cross-functional collaboration may make the most sense. “We have to bring in people from different disciplines. And then, of course, we need the machine learning and AI people,” said Wells Fargo’s Sudjianto. “Somebody who can lead that type of team holistically is very important.” Organizational flexibility is a centerpiece of all the AI models. For large companies, the culture change required to implement AI will be daunting, according to several of these executives.
A third challenge is figuring out how humans and computers can build off each other’s strengths. This is not easy. Amy Hoe, chief technology and operations officer of insurer FWD Group, says that companies need privileged access to data (which, according to our findings, many don’t have), they need to put in place flexible organizational structures, and they must learn how to make people and machines work effectively together. All of which means tough cultural changes for both company and employee.
Managers also need to realize that employing AI goes beyond improving upon the status quo. The real hard work is to understand the potential shift of entire value pools—as is expected in the health care industry, for example—and how to build sustainable competitive advantage in a changing environment. (See “Competing in the Age of Artificial Intelligence,” BCG article, January 2017, and “Putting Artificial Intelligence to Work ,” BCG article, forthcoming.)
AI and jobs
Contrary to recent dire predictions about AI’s effect on employment, our survey suggests cautious optimism. Most respondents, for example, do not expect that AI will lead to a reduction of jobs at their organization within the next five years. (See Exhibit 2.) Nearly 70% also said they are not fearful that AI will automate their own jobs. By a similar margin, respondents hope that AI will take over some of their more boring and unpleasant current tasks. However, respondents overwhelmingly agree that AI will both require employees to learn new skills within the next five years and augment their existing skills.
Just about any company today needs a plan with respect to AI. Most do not have one, and those that have been slower to move have some catching up to do. It’s not too late to start, but those companies that wait much longer will find the playing field tilted ever more steeply against them. The full report provides some concrete steps on what to do next.
Don't miss any update on this topic
Create a free account and access your personalized content collection with our latest publications and analyses.
License and Republishing
World Economic Forum articles may be republished in accordance with the Creative Commons Attribution-NonCommercial-NoDerivatives 4.0 International Public License, and in accordance with our Terms of Use.
The views expressed in this article are those of the author alone and not the World Economic Forum.
Related topics:
Forum Stories newsletter
Bringing you weekly curated insights and analysis on the global issues that matter.
More on Emerging TechnologiesSee all
Wesley Spindler, Luna Atamian Hahn-Petersen and Sadaf Hosseini
May 19, 2025
Elena Fersman
May 14, 2025
Rya G. Kuewor
May 13, 2025
Tea Trumbic and Dhivya O’Connor
May 13, 2025