We need to overcome AI's inherent human bias
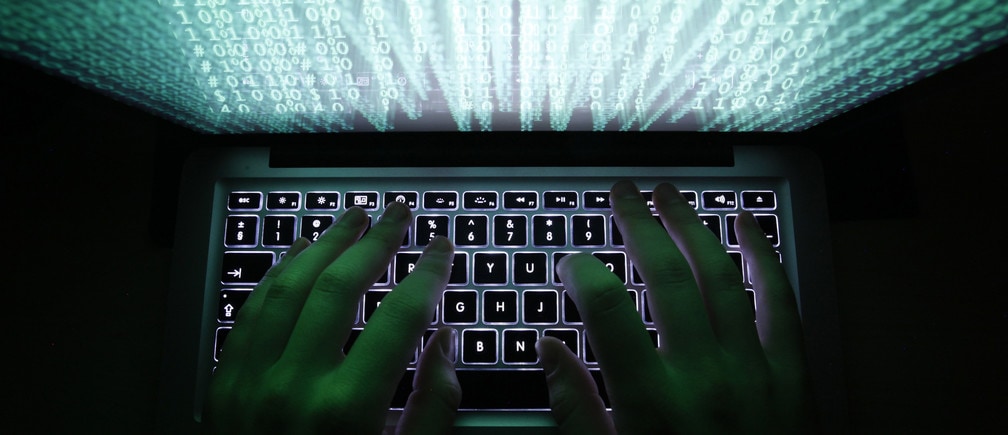
'Under the right set of circumstances, AI can perform in amazing ways - but it can also be extremely brittle' Image: REUTERS/Kacper Pempel
The development of artificial intelligence (AI) systems can often be biased and highly problematic, as recently shown by the Google AI algorithm that labelled some black people as gorillas and Microsoft's Tay Twitterbot that had to be shut down when it learned to be racist after just 16 hours.
It’s a misconception that AI is objective because it relies on mathematical computations; the construction of an AI system is an inherently human-driven process. It is unavoidable that such systems will contain bias.
When AI is noticeably biased, as in the examples above, that bias can often be corrected. This act of rectification shows us that the heart of AI is not the algorithm, but the human manipulating it. In many instances of more nuanced bias, it can be much more difficult to correct the system.
Consider an example that illustrates how a seemingly small choice in an AI algorithm can produce very different results. A very popular and widely available human resources dataset contains 15,000 observations of the nine factors that influenced employees’ decisions to resign from a company. The factors include elements such as employee satisfaction, how many projects he or she is working on, and length of service. Such data is useful to senior executives attempting to determine how best to allocate resources to retain employees.
A manager wishing to use AI to aid in employee retention programmes might choose between two very popular machine learning techniques: logistic regression and neural nets. A logistic regression algorithm tries to predict whether an event will happen (i.e. leave or stay) based on a set of statistically-relevant variables; while a neural net is a biologically–inspired approach that attempts to find patterns in the data that reveal significant relationships between variables (also known as features). When both algorithms are applied to this HR dataset, they have the same predictive accuracy of 94%, meaning neither one is superior in terms of performance.
Yet as illustrated in the table below, they point to two very different courses of action for a manager. The green cells in the table show the most likely factors behind an employee’s resignation and the red cells show the least likely, with the percentages indicating in each case how likely that particular reason was.
Looking at the logistic regression, the manager might believe that she could increase employee retention by focusing on the number of projects employees had and whether they’d been involved in a work accident. But looking at the neural net, salary becomes a leading factor, even though the logistic regression said there was little chance of salary making a difference in retention.
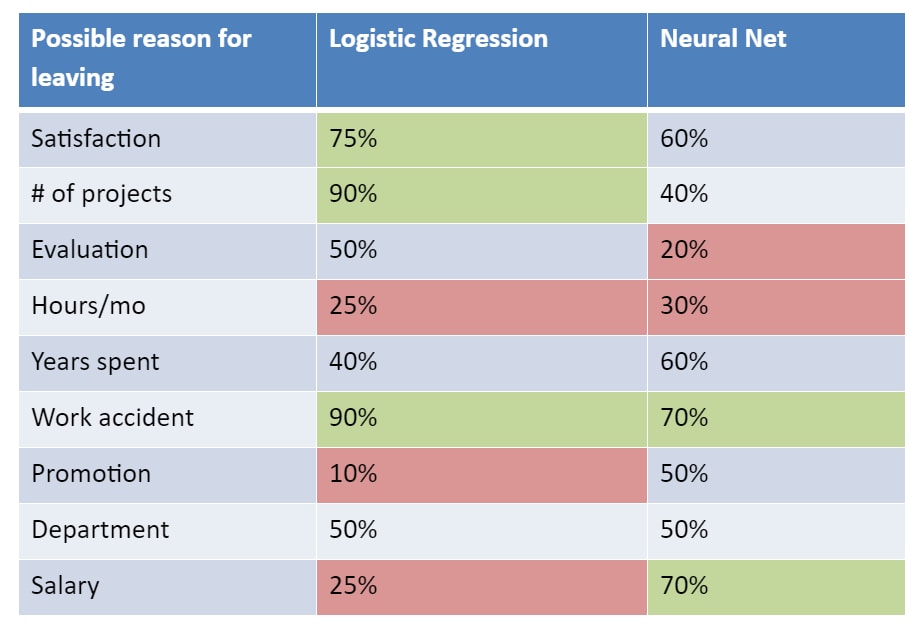
So which model should a manager believe? Should the company invest in improving job satisfaction, reducing the number of projects and reducing work accidents? Or will increasing salary and reducing work accidents be the best approach? Clearly in this case study, algorithm choice (remember that statistically, they are equally correct) would have a major impact on how a company commits its resources.
How and why to choose an algorithm will often have sound reasons, but such selections can often be a result of familiarity in terms of what was taught at one’s college (which is not standardized by any measure) or what a boss wants an employee to use (because that is often what he or she best understands). Developing a principled and documented approach to various types of data analytics, understanding the strengths and limitations of such approaches and educating the workforce on their application in a standardized manner would go a long way in helping companies in such endeavours. However, creating such new AI divisions and processes can be a daunting task for companies who struggle to hire enough qualified people in these areas.
Under the right set of circumstances, AI can perform in amazing ways. Given a large data set, an AI algorithm can reveal some relationship that’s either not recognizable by a human, such as a tumour in a medical scan, or that cannot be recognized as quickly by a human, like a pending collision in a car. But AI can also be extremely brittle: once outside a relatively narrow set of circumstances, defined and tweaked by the humans that have created them, it can fail spectacularly. Moreover, while in applications of AI, such as speech recognition, we can know with high certainty whether an outcome is correct, the same cannot be said for AI applications attempting to estimate human decision-making.
Algorithms are created by engineers and computer scientists who determine how to initialize various statistical parameters, which can be somewhat arbitrary, and then tune or adjust them to a specific data set to achieve the desired outcome. In some cases, human-designated labels are assigned to various cases to improve an algorithm’s overall predictive accuracy. These, often subjective, parameters can lead to a brittleness problem for AI algorithms, causing them to fail under unforeseen conditions. The recent crash of Google's self-driving car, which ran into a bus because it did not understand how to effectively merge into traffic, is just such an example.
One often overlooked source of AI bias is humans interpreting the results of algorithms. These complex AI algorithms produce sets of probabilities and clusters of figures that are akin to the infamous stream of numbers in the Matrix movies. Human experts in statistical reasoning must take these numbers and apply contextual meaning to them and then use that to instruct other humans or computers in how to interpret the results. Such instruction often takes the form of embedded algorithms and, because of this interdependence, it is often very difficult to reconstruct why an AI system performed in a particular way, which hides any bias that may have been added by the human interpreter layers before. Attempting to explain the results as well as the possible limitations of the solution space is very difficult.
Researchers are beginning to realize these difficulties and a new field called explainable AI is emerging. Recognizing that most of the algorithms and probabilistic reasoning on which they rely are very difficult to understand, researchers are attempting to determine how to best design or represent AI algorithm reasoning so that cause and effect are easier to understand.
Our industries can help this fledgling field by establishing new requirements for explainable AI that will, in turn, provide the necessary motivation for engineers and computer scientists to value the holistic AI system (human decision maker + algorithm), instead of trying to best each other with clever new algorithms that only they can understand. With AI's increasing application in safety-critical applications, such as medical devices, cars, planes and trains, as well as in financial trading and even in smart homes, it is imperative that we find a way to communicate when and where an AI-enabled system could fail so that we can develop resilient systems instead of brittle ones.
Don't miss any update on this topic
Create a free account and access your personalized content collection with our latest publications and analyses.
License and Republishing
World Economic Forum articles may be republished in accordance with the Creative Commons Attribution-NonCommercial-NoDerivatives 4.0 International Public License, and in accordance with our Terms of Use.
The views expressed in this article are those of the author alone and not the World Economic Forum.
Stay up to date:
Emerging Technologies
Related topics:
Forum Stories newsletter
Bringing you weekly curated insights and analysis on the global issues that matter.
More on Emerging TechnologiesSee all
Michele Mosca and Donna Dodson
December 20, 2024