3 ways to teach robots right from wrong
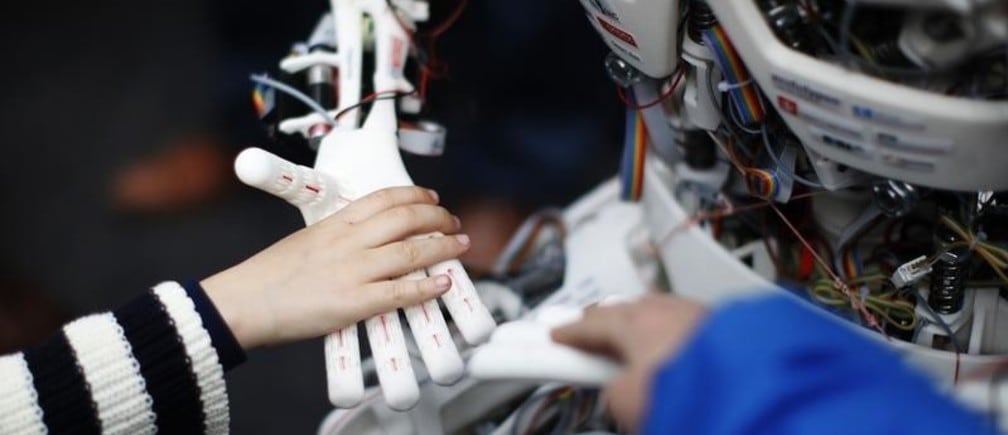
If we can't agree on what makes a moral human, how can we design moral robots? Image: REUTERS/Michael Buholzer
Today, it is difficult to imagine a technology that is as enthralling and terrifying as machine learning. While media coverage and research papers consistently tout the potential of machine learning to become the biggest driver of positive change in business and society, the lingering question on everyone’s mind is: “Well, what if it all goes terribly wrong?”
For years, experts have warned against the unanticipated effects of general artificial intelligence (AI) on society. Ray Kurzweil predicts that by 2029 intelligent machines will be able to outsmart human beings. Stephen Hawking argues that “once humans develop full AI, it will take off on its own and redesign itself at an ever-increasing rate”. Elon Musk warns that AI may constitute a “fundamental risk to the existence of human civilization”. Alarmist views on the terrifying potential of general AI abound in the media.
More often than not, these dystopian prophecies have been met with calls for a more ethical implementation of AI systems; that somehow engineers should imbue autonomous systems with a sense of ethics. According to some AI experts, we can teach our future robot overlords to tell right from wrong, akin to a “good Samaritan AI” that will always act justly on its own and help humans in distress.
Although this future is still decades away, today there is much uncertainty as to how, if at all, we will reach this level of general machine intelligence. But what is more crucial, at the moment, is that even the narrow AI applications that exist today require our urgent attention in the ways in which they are making moral decisions in practical day-to-day situations. For example, this is relevant when algorithms make decisions about who gets access to loans or when self-driving cars have to calculate the value of a human life in hazardous situations.
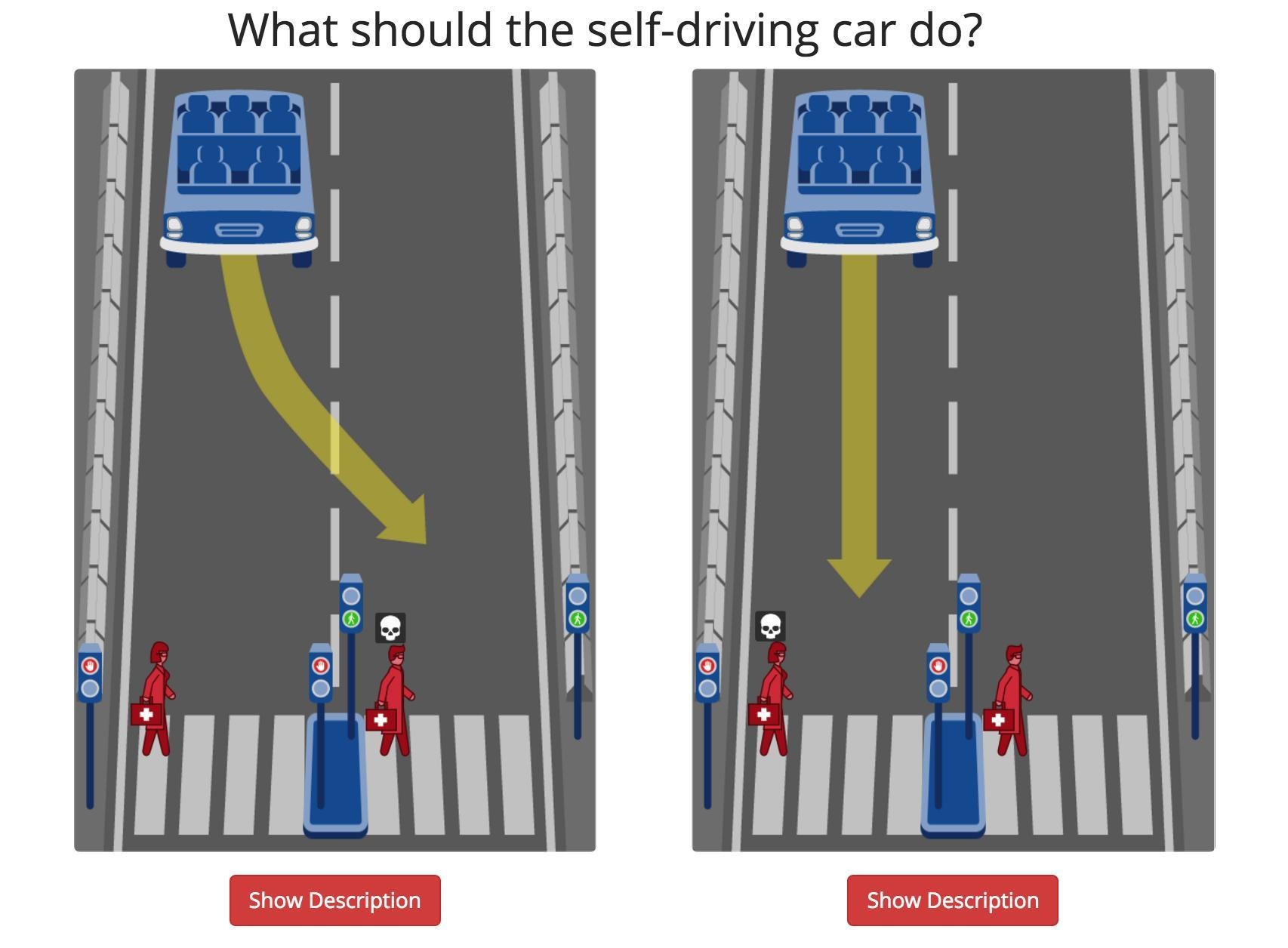
Teaching morality to machines is hard because humans can’t objectively convey morality in measurable metrics that make it easy for a computer to process. In fact, it is even questionable whether we, as humans have a sound understanding of morality at all that we can all agree on. In moral dilemmas, humans tend to rely on gut feeling instead of elaborate cost-benefit calculations. Machines, on the other hand, need explicit and objective metrics that can be clearly measured and optimized. For example, an AI player can excel in games with clear rules and boundaries by learning how to optimize the score through repeated playthroughs.
After its experiments with deep reinforcement learning on Atari video games, Alphabet’s DeepMind was able to beat the best human players of Go. Meanwhile, OpenAI amassed “lifetimes” of experiences to beat the best human players at the Valve Dota 2 tournament, one of the most popular e-sports competitions globally.
But in real-life situations, optimization problems are vastly more complex. For example, how do you teach a machine to algorithmically maximise fairness or to overcome racial and gender biases in its training data? A machine cannot be taught what is fair unless the engineers designing the AI system have a precise conception of what fairness is.
This has led some authors to worry that a naive application of algorithms to everyday problems could amplify structural discrimination and reproduce biases in the data they are based on. In the worst case, algorithms could deny services to minorities, impede people’s employment opportunities or get the wrong political candidate elected.
Based on our experiences in machine learning, we believe there are three ways to begin designing more ethically aligned machines:
AI researchers and ethicists need to formulate ethical values as quantifiable parameters. In other words, they need to provide machines with explicit answers and decision rules to any potential ethical dilemmas it might encounter. This would require that humans agree among themselves on the most ethical course of action in any given situation – a challenging but not impossible task. For example, Germany’s Ethics Commission on Automated and Connected Driving has recommended to specifically programme ethical values into self-driving cars to prioritize the protection of human life above all else. In the event of an unavoidable accident, the car should be “prohibited to offset victims against one another”. In other words, a car shouldn’t be able to choose whether to kill one person based on individual features, such as age, gender or physical/mental constitution when a crash is inescapable.
Engineers need to collect enough data on explicit ethical measures to appropriately train AI algorithms. Even after we have defined specific metrics for our ethical values, an AI system might still struggle to pick it up if there is not enough unbiased data to train the models. Getting appropriate data is challenging, because ethical norms cannot be always clearly standardized. Different situations require different ethical approaches, and in some situations there may not be a single ethical course of action at all – just think about lethal autonomous weapons that are currently being developed for military applications. One way of solving this would be to crowdsource potential solutions to moral dilemmas from millions of humans. For instance, MIT’s Moral Machine project shows how crowdsourced data can be used to effectively train machines to make better moral decisions in the context of self-driving cars.
Policymakers need to implement guidelines that make AI decisions with respect to ethics more transparent, especially with regard to ethical metrics and outcomes. If AI systems make mistakes or have undesired consequences, we cannot accept “the algorithm did it” as an adequate excuse. But we also know that demanding full algorithmic transparency is technically untenable (and, quite frankly, not very useful). Neural networks are simply too complex to be scrutinized by human inspectors. Instead, there should be more transparency on how engineers quantified ethical values before programming them, as well as the outcomes that the AI has produced as a result of these choices. For self-driving cars, for instance, this could imply that detailed logs of all automated decisions are kept at all times to ensure their ethical accountability.
We believe that these three recommendations should be seen as a starting point for developing ethically aligned AI systems. Failing to imbue ethics into AI systems, we may be placing ourselves in the dangerous situation of allowing algorithms to decide what’s best for us. For example, in an unavoidable accident situation, self-driving cars will need to make some decision for better or worse. But if the car’s designers fail to specify a set of ethical values that could act as decision guides, the AI system may come up with a solution that causes more harm. This means that we cannot simply refuse to quantify our values. By walking away from this critical ethical discussion, we are making an implicit moral choice. And as machine intelligence becomes increasingly pervasive in society, the price of inaction could be enormous – it could negatively affect the lives of billions of people.
Machines cannot be assumed to be inherently capable of behaving morally. Humans must teach them what morality is, how it can be measured and optimised. For AI engineers, this may seem like a daunting task. After all, defining moral values is a challenge mankind has struggled with throughout its history. Nevertheless, the state of AI research requires us to finally define morality and to quantify it in explicit terms. Engineers cannot build a “good samaritan AI”, as long as they lack a formula for the good samaritan human.
Don't miss any update on this topic
Create a free account and access your personalized content collection with our latest publications and analyses.
License and Republishing
World Economic Forum articles may be republished in accordance with the Creative Commons Attribution-NonCommercial-NoDerivatives 4.0 International Public License, and in accordance with our Terms of Use.
The views expressed in this article are those of the author alone and not the World Economic Forum.
Stay up to date:
Electronics
Related topics:
Forum Stories newsletter
Bringing you weekly curated insights and analysis on the global issues that matter.
More on Emerging TechnologiesSee all
Michele Mosca and Donna Dodson
December 20, 2024