How to manage AI's risks and rewards
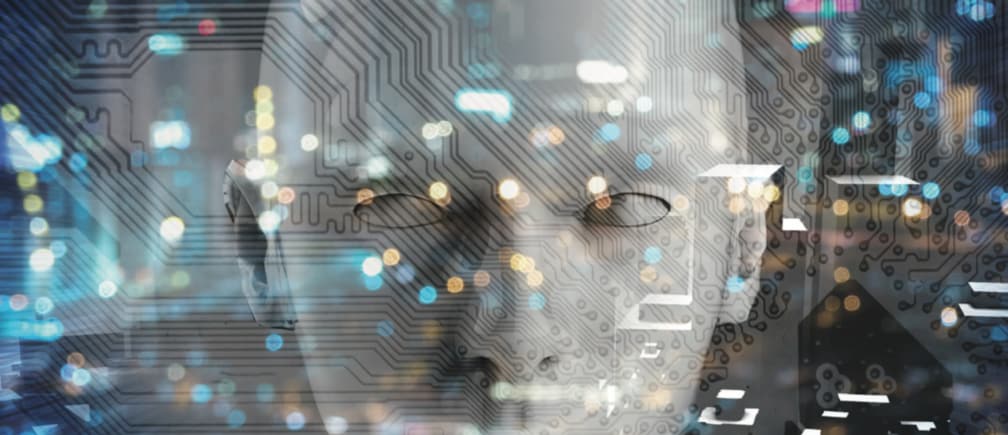
This article is part of the World Economic Forum's Geostrategy platform
Technological advances in artificial intelligence (AI) promise to be pervasive, with impacts and ramifications in health, economics, security and governance.
In combination with other emerging and converging technologies, AI has the potential to transform our society through better decision-making and improvements to the human condition.
But, without adequate risk assessment and mitigation, AI may pose a threat to existing vulnerabilities in our defences, economic systems, and social structures, argue the authors of the Wilson Center report, Artificial Intelligence: A Policy-Oriented Introduction, Anne Bowser, Michael Sloan, Pietro Michelucci and Eleonore Pauwels.
Recognizing the increasing integration of technology in society, this policy brief grounds the present excitement around AI in an objective analysis of capability trends before summarizing perceived benefits and risks. It also introduces an emerging sub-field of AI known as Human Computation, which can help achieve future AI capabilities by strategically inserting humans in the loop where pure AI still falls short.
Policy recommendations suggest how to maximize the benefits and minimize the risks for science and society, particularly by incorporating human participation into complex socio-technical systems to ensure the safe and equitable development of automated intelligence.
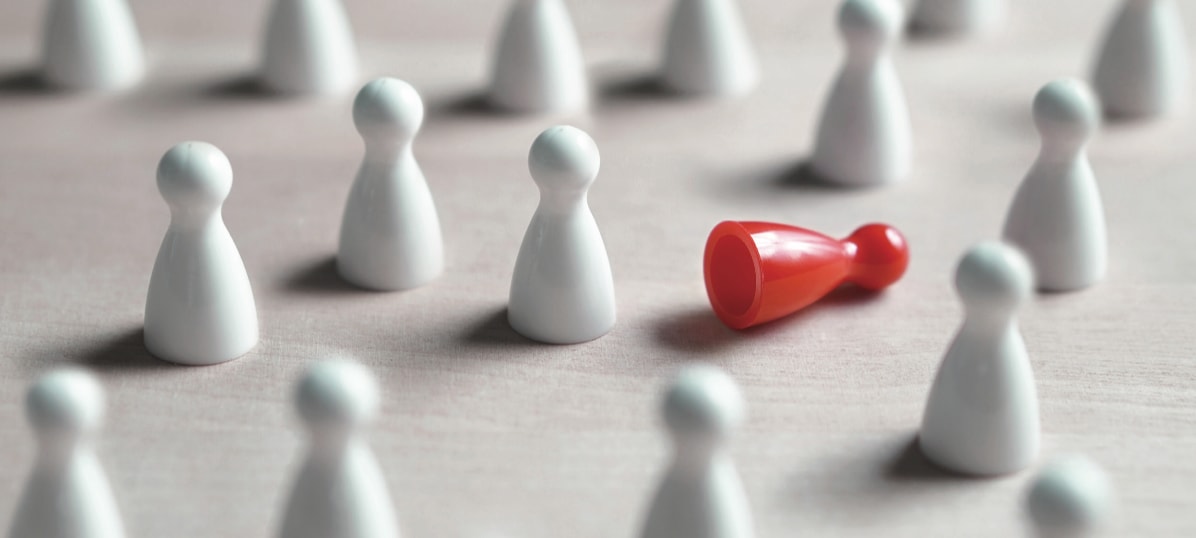
The report offers a number of key recommendations:
Planning in an Age of Complexity: Recommendations for Policymakers and Funders
AI is a critical component of the fourth industrial revolution (4IR), “a fusion of technologies that is blurring the lines between the physical, digital, and biological spheres.”
The fourth industrial revolution is characterized by the convergence of new and emerging technologies in, complex socio-technical systems that permeate every aspect of human life
”Compared to previous revolutions involving processes like mechanization, mass production, and automation, the fourth industrial revolution is characterized by the convergence of new and emerging technologies in, complex socio-technical systems that permeate every aspect of human life.
Convergence also implies the increasing interaction of multiple fields, such as AI, genomics and nanotechnology, which rapidly expands the range of possible impacts that need to be considered in any science policy exercise.
Ten years ago, nanotechnology was celebrated largely for its impacts on chemistry and material sciences. But the ability to precision engineer matter at genetically relevant scale has resulted in significant advances in genomics and neurosciences, such as creating the ability to model networks of neurons. This example illustrates how the convergence of two emerging technologies —AI and genomics—leads to advances beyond the initial capabilities of either alone.
Meeting the challenges of convergence requires drawing on a wide range of expertise, and taking a systems approach to promoting responsible research and innovation.
As “outsiders” to the AI design processes, it is extremely difficult for policymakers to estimate AI development due to limited comprehension of how the technology functions. Many may also draw inspiration from traditional regulatory models that are inadequate for AI, playing a catch-up game to decode the terms of reference used by researchers, or fall victim to the human fallacy of overestimating the short-term capabilities of new technologies.
There will be significant systems’ transformations through AI over the next few decades, but perhaps it will be more incremental than we fear or imagine.
Conduct broad and deep investigations into AI with leading researchers from the private sector and universities.
In the US, early reports from policy bodies and researchers at institutions such as Stanford offer high-level roadmaps of AI R&D. Expert groups convening under organizations like IEEE compliment these overviews with in-depth considerations of things like ethically-aligned AI design to maximize human well-being.
In the near-future, AI researchers involved in collaboration with policymakers should conduct additional in-depth studies to better understand and anticipate aspects of AI related to (for example) job automation at a more granular level, considering impact across time, sectors, wage levels, education degrees, job types and regions. For instance, rather than low-skills jobs that require advanced hand-dexterity, AI systems might more likely replace routine but high-level cognitive skills. Additional studies could investigate areas like national security.
Advocate for a systems approach to AI research and development that accounts for other emerging technologies and promotes human participation.
It is in our reach to build networks of humans and machines that sense, think, and act collectively with greater efficacy than either humans or AI systems alone
”AI seeks to replicate human intelligence in machines – but humanlike intelligence already exists in humans. Today there is an opportunity to develop superhuman intelligence by pairing the complementary abilities of human cognition with the best available AI methods to create hybrid distributed intelligent systems. In other words, it is in our reach to build networks of humans and machines that sense, think, and act collectively with greater efficacy than either humans or AI systems alone.
The emerging subfield of AI known as Human Computation is exploring exactly those opportunities by inserting humans into the loop in various information processing systems to perform the tasks that exceed the abilities of machine AI. For this reason, human computation is jokingly referred to as “Artificial AI”.
Powerful examples of human computation can be found in citizen science platforms, such as stardust@home, in which 30,000 participants discovered five interstellar dust particles hidden among a million digital images, or Stall Catchers, where thousands of online gamers help accelerate a cure for Alzheimer’s disease.
Human Computation also includes crowdsourcing platforms like Wikipedia, which aggregates information into the world’s most comprehensive collection of human knowledge, or reCAPTCHA, which has the dual purpose of validating humans for websites while extracting information from images.
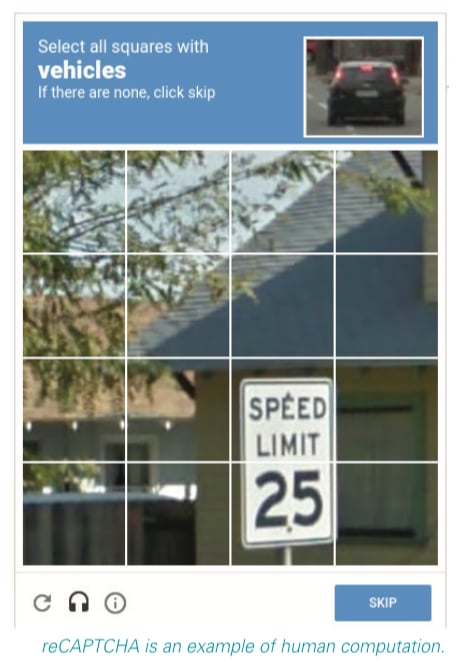
Furthermore, platforms like Habitat Network leverage the reciprocity of online and offline behaviour to influence conservation behaviour in people’s own backyards.
This kind of collective action on a massive scale may be the only foil against societal issues that arose due to deleterious collective action, such as combustion engine usage. Examples from these “first generation” human computation applications only hint at the untapped potential of hybrid human/machine systems.
Human computation approaches to AI address such dystopian concerns by advancing the design of AI systems with human stakeholders in the loop who drive the societally-relevant decisions and behaviours of the system. T
The conscientious development of AI systems that carefully considers the coevolution of humans and technology in hybrid thinking systems will help ensure that humans remain ultimately in control, individually or collectively, as systems achieve superhuman capabilities.
Promote innovation, avoid centralizing and dramatically expanding regulation.
Current regulations regarding AI are as additions to products, and thus, subsumed under existing legislative authorities. For example, the Food and Drug Administration (FDA) regulates precision medicine initiatives, while the National Highway Traffic Safety Administration under the DoT issues guidance around autonomous vehicles while leaving key decisions to the states. Experts agree that expanding and centralizing regulation will inhibit growth.
Recognizing that “ambiguous goals” coupled with broad transparency requirements have encouraged firms to prioritize consumer privacy, some researchers advocate for a similar approach in AI, where strong transparency requirements promote innovation yet force accountability.
At the same time there is a need to safeguard the public interest by addressing shared challenges. That includes developing safety guidelines and accident investigation processes, protecting individual privacy and public security, designing systems with transparent decision-making, and managing public perception through effective science communication.
Seek to maximize benefits and minimize risks by issuing guidance and by building or supporting subject-specific communities. These efforts, in turn, help to encourage broad perspective and creative activity.
To promote top-down coordination within the federal government, the Obama Administration chartered a National Science and Technology Council (NSTC) Subcommittee on Machine Learning and Artificial Intelligence in 2016.
But cross-agency innovation also comes from grassroots, bottom-up communities. The federal communities of practices (COP)s supported by Digital.gov are important hubs for sharing and developing common strategies.
One COP already explores the role of artificial intelligence in delivering citizen services. Executive guidance could encourage more COPs to form.
The public sector is an important stakeholder in AI R&D, as well as a valuable source of expertise.
As a first step towards engaging the public the Obama Administration co-hosted five workshops with a few universities and NGOs and issued a public Request for Information (RFI). Additional gatherings could leverage the knowledge of industry leaders as well as state and local government advocates and customers.
Beyond one-off events, in line with government ideals of openness and transparency ongoing programmes for public engagement and outreach should also be supported. One example of a programme already active is the Expert & Citizen Assessment of Science & Technology (ECAST) network, which brings universities, nonpartisan policy research organizations, and science educators together to inform citizens about emerging science and technology policy issues, and solicit their input to promote more fully informed decision- making.
Advance artificial intelligence R&D through grants as well as prize and challenge competitions.
While basic research can take decades to yield commercial results, research such as Claude Shannon’s information theory, which enabled later developments such as the Internet, illustrate the value of theoretical work.
Foundational research to advance AI should help develop safety and security guidelines, explore ways to train a capable workforce, promote the use of standards and benchmarks, and unpack key ethical, legal, and social implications.
Agencies should continue to prioritize areas including robotics, data analysis, and perception while also advancing knowledge and practice around human-AI collaboration, for example through human computation.
Applied AI R&D unfolds in an innovation ecosystem where diverse stakeholders strategically collaborate and compete by applying for funding, procuring new technologies, and hiring top researchers.
Funding agencies should incentivize collaboration by bringing industry, academia, and government interests together in pre-competitive research. NSF’s Industry–University Cooperative Research Centers Program (IUCRC) already enables such collaboration to advance foundations for autonomous airborne robotics.
There are also Small Business Innovation Awards (SBIRs), which can allow small companies the opportunity to explore high-risk, high-reward technologies with commercial viability.
While the impacts of prize and challenge competitions are difficult to quantify, DARPA believes that ten years after the Grand Challenge “defence and commercial applications are proliferating.”
Prizes and challenges allow agencies to incentivize private sector individuals and groups to share novel ideas or create technical and scientific solutions. These mechanisms are low-cost opportunities to explore targeted AI developments that advance specific agency missions.
Don't miss any update on this topic
Create a free account and access your personalized content collection with our latest publications and analyses.
License and Republishing
World Economic Forum articles may be republished in accordance with the Creative Commons Attribution-NonCommercial-NoDerivatives 4.0 International Public License, and in accordance with our Terms of Use.
The views expressed in this article are those of the author alone and not the World Economic Forum.
Stay up to date:
Artificial Intelligence
Related topics:
The Agenda Weekly
A weekly update of the most important issues driving the global agenda
You can unsubscribe at any time using the link in our emails. For more details, review our privacy policy.
More on Emerging TechnologiesSee all
David Elliott
November 25, 2024