We trained an algorithm to detect cancer in just 2 hours
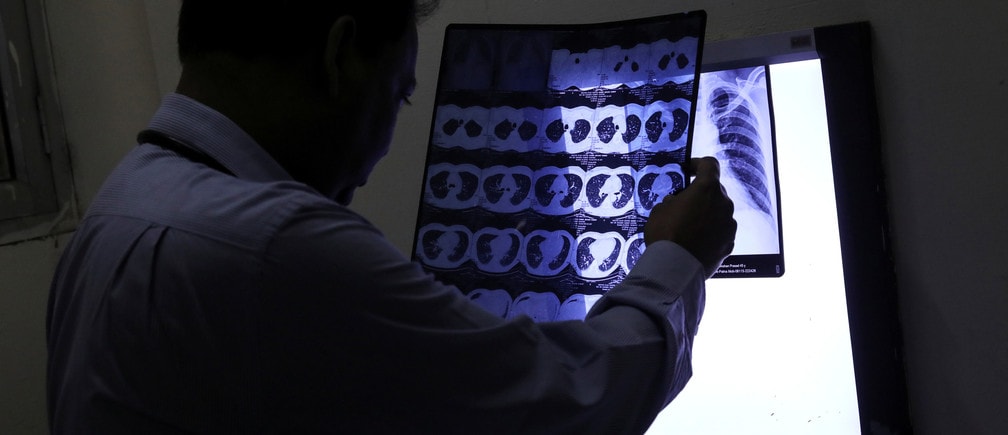
Years of medical training in just two hours. Image: REUTERS/Anushree Fadnavis - RC1B7CBFA290
Doctors across the world are beginning to rely on artificial intelligence algorithms to help accelerate diagnostics and treatment plans, with the goal of making more time to see more patients, with greater precision. We all can understand—at least conceptually—what it takes to be a doctor: years of medical school lectures attended, stacks of textbooks and journals read, countless hours of on-the-job residencies. But the way AI has learned the medical arts is less intuitive.
In order to get more clarity on how algorithms learn these patterns, and what pitfalls might still lurk within the technology, Quartz partnered with Leon Chen, co-founder of medical AI startup MD.ai, and radiologist Luke Oakden-Rayner, to train two algorithms and understand how it matches with a medical professional as it learns. One detects the presence of tumorous nodules, and the second gauges the potential of it being malignant.
The artificial intelligence being developed for medical use is typically complicated pattern matching: An algorithm is shown many many medical scans of organs with tumors, as well as tumor-free images, and tasked with learning the patterns that differentiate the two categories.
We showed our algorithms nearly 200,000 images of malignant, benign, and tumor-free CT scans, in both 2D and 3D. The way we measure how accurate the nodule detection algorithm is as it learns to find these tumors is the same as they would be implemented in a specialist’s office, with a metric called “recall.” Recall tells us the percentage of nodules the algorithm catches, given a set number of false alarms. For instance, 60% for Recall@1 means it would catch 60% of tumors, with one false alarm per scan allowed. For the malignancy algorithm, it’s simply the percent of correctly identified nodules.
Theoretically, you could set the false-alarm threshold higher or lower, but it would impact the percentage of nodules caught. If four false alarms were allowed per nodule caught, for example, the percentage would go up. In real-world settings, more false alarms mean unnecessary tests for patients. But each doctor could be comfortable with different levels of algorithmic sensitivity, either prioritizing accuracy or fewer false positives, depending on their own workflow.
Don't miss any update on this topic
Create a free account and access your personalized content collection with our latest publications and analyses.
License and Republishing
World Economic Forum articles may be republished in accordance with the Creative Commons Attribution-NonCommercial-NoDerivatives 4.0 International Public License, and in accordance with our Terms of Use.
The views expressed in this article are those of the author alone and not the World Economic Forum.
Stay up to date:
Digital Communications
Related topics:
The Agenda Weekly
A weekly update of the most important issues driving the global agenda
You can unsubscribe at any time using the link in our emails. For more details, review our privacy policy.
More on Health and Healthcare SystemsSee all
Shyam Bishen
November 20, 2024