Understanding what works for active labour market policies
The effectiveness of complex policies such as ALMPs depends on how they are designed.
Image: REUTERS/Konstantin Chernichkin
Stay up to date:
Inclusive Growth Framework
Having an effective set of active labour market policies (ALMPs) is essential to meet the challenges that automation, globalisation and demographic change impose on the labour market. Active Labour Market Policies are a general denomination for specific policies that could be broadly grouped into four big policy clusters – vocational training, assistance in the job search process, wage subsidies or public works programmes, and support to micro-entrepreneurs or independent workers. Governments allocate significant fiscal resources to ALMPs (in the past 10 years, such policies have accounted for more than 0.5% of the GDP of OECD countries) to reduce unemployment levels, increase labour income and facilitate the adoption of new technologies that boost productivity. However, evidence is often scarce and mixed as a guide in the design of effective policy solutions.
In a recent paper (Levy Yeyati et al. 2019), we analysed the effectiveness of these policies through a systematic review of more than 100 experimental evaluations that documented the effectiveness of ALMPs implemented worldwide. Specifically, we focus exclusively on programmes evaluated through Randomised Control Trials (RCTs), exploiting the fact that the past five years have witnessed a flurry of RCTs that shed new light on the impact and cost effectiveness of ALMPs (Figure 1).1 This focus on RCTs reduces the number of relevant evaluations, but allows us to focus on estimates with high internal validity and to refine the metrics used to compare results, making the findings from individual evaluations more naturally comparable.2
Figure 1 Distribution of studies included in our sample according to the year of publication
The effectiveness of multidimensional and complex policies such as ALMPs depends on how they are designed, on the quality of their implementation, on the context in which they were developed, and on their target population. For example, a vocational training programme may differ in its cost and duration, in its curricular content, and in whether or not, and how, the private sector participates, and may address a very diverse public, from experienced software programmers in Tokyo or Chicago to disadvantaged youth in the state of Madhya Pradesh. An analysis that ignores these considerations can hardly give specific and conclusive lessons for policymakers.
Following Pritchett et al. (2013), our four policy clusters can be treated as ‘classes’ of policies that could be designed and implemented in very different ways and target diverse demographic groups, with widely varying effectiveness. A review that does not consider this variability could draw conclusions of the type ‘wage subsidies work’ or ‘vocational training does not work’, statements as imprecise as ‘the ingestion of chemical components works’.3 To account for the dimensionality of the problem in an operational way, we propose a design space (a parsimonious version of the space of all of the possible instances of a class of policy, arrived at by specifying all of the choices necessary for a project to be implemented) that characterises: (i) the specific components in which the programmes can be decomposed; (ii) the implementation features and the type of public-private participation; and (iii) the economic context and the target population of the programmes. This allows us to refine the analysis and identify why policies that are similar on paper can differ in their impact and cost-effectiveness, and to isolate the specific effect of a large set of standardised variables that granularly describe the design, implementation, context and target population of our 102 ALMPs. To characterise these dimensions, we analysed all the information available in the academic publications and condensed the description of the criteria used for the identification of each of the variables into unified protocols that articulate the review process.
Comparing the overall impact of the four policy clusters analysed, we find that wage subsidies and independent worker assistance show the greatest median impact on earnings relative to the control group, with improvements of 16.7% and 16.5%, respectively. On the other hand, vocational training programmes have a median impact of 7.7%, while employment services show an almost negligible impact. The median impact on employment exhibits a similar pattern, with wage subsidies being the type of programme which reports the highest impact on this outcome category, while independent worker assistance and vocational training show a median impact of 11% and 6.7%, respectively. Interestingly, employment services interventions have a median impact of 2.6%, consistent with short-lived and inexpensive interventions that do not attempt to help build human capital, but rather to improve the propensity to find employment.
Importantly, there is a substantial variability in reported impacts on earnings and employment outcomes. This is especially true for the type of interventions in which we have more than 10 cases, such as employment services, independent worker support or assistance, and vocational training (Figure 2).
Figure 2 Boxplot of the 652 coefficients according to the estimated effect relative to the control group
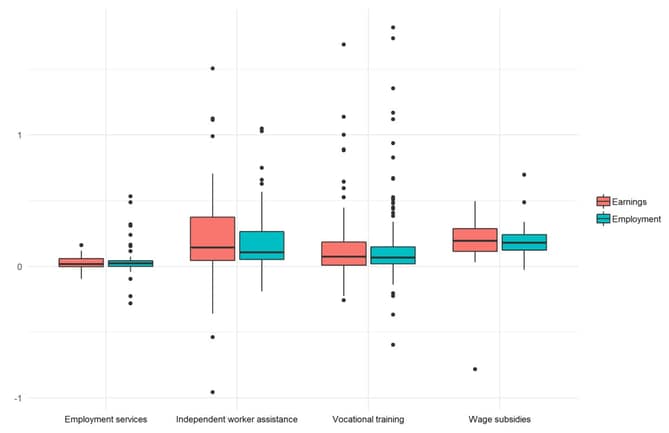
When the information is available, we add a continuous variable that identifies the average cost per person of the intervention, in 2010 PPP dollars. It is important to stress that only 51 interventions reported this critical variable, and only 22 carried out a rigorous cost-benefit analysis by means of net present value, internal rate of return or payback periods, highlighting an important limitation of the usual practice in the impact evaluation literature.
Although the sample of ALMPs for which we have cost data is limited, we can identify some indicative patterns. Wage subsidies, support to independent workers or micro-entrepreneurs and vocational trainings have comparable median cost per participant, ranging from 1,744 and 1,518 2010 PPP US dollars, with much greater variability in the second group. In turn, employment services are notably less expensive policies, with a median cost per participant of 277 2010 PPP US dollars and limited variability across programmes (Figure 3).
Figure 3 Boxplot of unit costs, cost per treated participant by four-way programme classification, 2010 PPP US Dollars
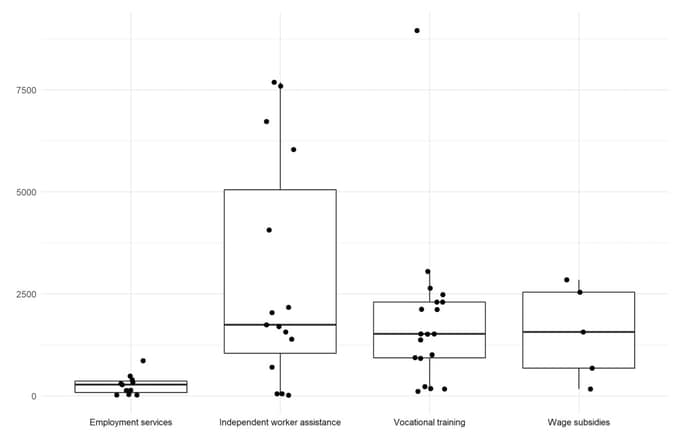
The reported impacts of ALMPs on employment and earnings outputs, although moderately positive on average, are subject to a great variability due to the multidimensional design space of these policies.
As we pointed out, ALMPs are generally complex policies with high-dimensional design spaces, highly dependent on contextual factors and the quality of their implementation.4 Any systematic review that does not describe the design space of the policies evaluated and considers the existing variability within the same intervention class or their interactions with the context and the target population, may have limited use from a practical policy perspective.
To address this drawback, we run meta-analytic regressions that exploit the descriptive granularity of the design space, to identify policy components and contextual factors associated with a greater probability of success. Figure 4 summarises the main findings with statistical significance at conventional level of eight different models. They are the combination of two cut-offs for the positive and statistically significant binary variable (5% and 10%) and four subsamples.
Figure 4 Main findings with statistical significance at conventional levels in meta-analytic regressions
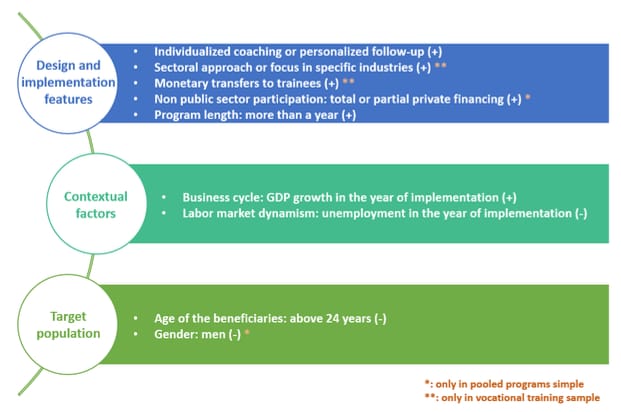
Several insights arise from this exercise:
- Design. Individualised coaching or follow-up of the participants, training exclusively focused on a specific industry, and the provision of monetary incentives to trainees all correlate with better outcomes in vocational training programmes (the most frequent ALMPs in our dataset).
- Context. The effectiveness of a programme correlates positively with growth and negatively with unemployment.
- Target. Training programmes tend to be more effective for young people (we find no significant difference across genders or educational levels).
Improving the effectiveness of this empirical research would require the systematic collection of granular and valuable information, from agreed protocols to publish relevant information in a systematised and tabulated registry. The design space proposed in the paper is a preliminary version of such a protocol.
Don't miss any update on this topic
Create a free account and access your personalized content collection with our latest publications and analyses.
License and Republishing
World Economic Forum articles may be republished in accordance with the Creative Commons Attribution-NonCommercial-NoDerivatives 4.0 International Public License, and in accordance with our Terms of Use.
The views expressed in this article are those of the author alone and not the World Economic Forum.
Forum Stories newsletter
Bringing you weekly curated insights and analysis on the global issues that matter.
More on Equity, Diversity and InclusionSee all
Thelma Obiakor and Bisong Anthony Ekpang
May 23, 2025
Victor Dzau and Paula Bellostas Muguerza
May 21, 2025
Silja Baller
May 15, 2025
Tea Trumbic and Dhivya O’Connor
May 13, 2025
Harrison Lung and Hatem Bamatraf
May 7, 2025