How local modelling can build resilience in the post-COVID era
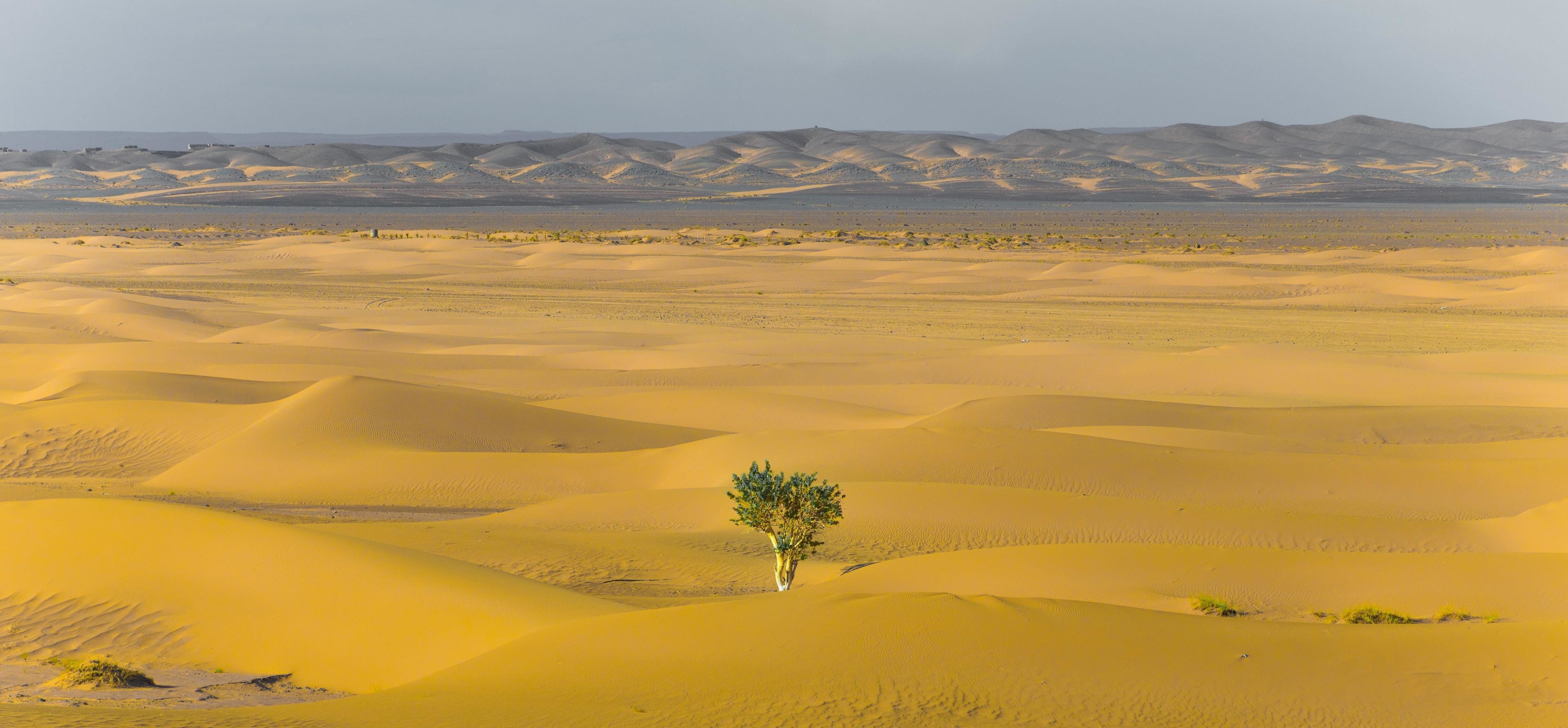
How can businesses adapt and thrive in a world shaken by multiple risks? Image: Karim Manjra on Unsplash
- Post-COVID-19, we must build more resilient supply chains and infrastructure to prepare for the next crisis.
- Technology today allows us to assess risks at a hyper-local level.
- This level of detail will be key to fostering the resilience we need.
The COVID-19 pandemic has cruelly exposed how unprepared many governments and institutions are to deal with complex, cross-border risks. Governments have adopted a range of approaches during this crisis, with differing outcomes often leading to unexpectedly high excess mortality and increasing economic costs. This fragmented response could result in the disease gaining renewed traction through the end of 2020 and beyond.
COVID-19's spread has accelerated in areas characterized by high population density, environmental degradation, poor urban infrastructure and inadequate healthcare. This disease exacerbates the battering many urban areas have already taken recently due to climate change-induced natural disasters. Society can no longer afford to wait until the next disaster strikes as globalization, urbanization, deforestation and industrial food production propagate new diseases and affect nations' food, energy and economic security. Globally-local prevention services to foster resilience are no longer a 'nice to have' but a 'must do'.
In the dynamic post-COVID-19 normal, society must develop deeper resilience within its institutions and processes. Against the backdrop of rapidly changing cultures, increasing digitalization, growing demographic shifts and increasing wealth inequality, the interconnected nature of health, economics, politics and technology makes the definition of 'normal' a moving target. COVID-19 is accelerating this pace and scope of change while exposing inadequacies.
More than ever, government leaders and corporate executives require better decision support. To date, robust technology-enabled, decision-support systems have been fragmented and unevenly deployed. This needs to change. In order to survive a potential economic depression, industry and government must work fist-in-glove to ensure resilient supply chains and infrastructures and prepare for scenarios in which catastrophes hit simultaneously, such as a hurricane like Cyclone Amphan and subsequent evacuation during a pandemic.
Many governments already collect massive amounts of data, and some companies have developed an impressive array of risk and resilience-modelling technologies. Unfortunately, the relevant data are mostly not well-curated, and advanced technologies are largely not in the hands of key decision-makers.
Disaster evacuation during COVID-19
India and Bangladesh were hit recently by Cyclone Amphan, which threatened millions of lives. They are not alone. The first of June this year marked the start of hurricane season in the US and typhoon season in Japan. The global nature of these interconnected risks makes globally-local solutions even more essential to building resilient societies.
In the context of a pandemic, social distancing and hygiene are as important in reducing transmission in evacuation shelters as during non-disaster situations. Unfortunately, there are much greater obstacles in the way of compliance and enforceability. Hurricane evacuations require that people move while in close contact to each other, exacerbating the threat of contagion. As a result, hurricane evacuations, which are difficult in their own right, are further complicated by shelter-in-place restrictions and could reseed the pandemic. According to One Concern's SEIR model (a compartmental epidemiological model), the number of infected people can increase by up to 20% over three days during an evacuation.
This approach to this challenge adapts compartmental epidemiological models to run at a hyper-local scale to develop new pandemic-related solutions. These solutions model the potential impact of hurricane evacuations on the spread of COVID-19 by tracking transitions between those who are susceptible, exposed, asymptomatic, pre-symptomatic, symptomatic (mild and severe), hospitalized and recovered in order to optimize disaster response.
Infection risk can then be modelled in densely populated areas, providing insights that can be used when planning the reopening of schools, churches and workplaces. By using hyper-local infection-rate data, decision-makers can assess hazards regarding the spread of infectious diseases.
Predictive analytics guide a safe return to work
Bringing back employees and customers safely is currently a key issue for business leaders. Executives need to rethink their productivity and workforce strategies to better prepare for a potential second COVID-19 wave and to build lasting resilience.
A recent analysis examined three US automotive factories (the Tesla factory in Fremont, California, the Ford factory in Chicago, Illinois, and the Mercedes-Benz factory in Tuscaloosa, Alabama) to predict infection rates, which would impact automotive manufacturers' decisions regarding production. We considered geography, the reopening date, number of employees, local COVID-19 case rate over two weeks, death rate in the local population, and factory age. For example, the modelled solution predicted a 99% chance that one particular factory would have at least one worker who would test positive for COVID-19. Two days later, employees at that factory did indeed test positive, forcing the owner to temporarily halt production at a cost of millions of dollars. Resilience-as-a-service's hyper-local pandemic models can help decision-makers speed up recovery and minimize disruption.
Resilience-as-a-service in insurance
Pandemic risk-awareness has risen significantly due to COVID-19, with losses driving new policy exclusions and crippling businesses. Given its coverage and claim-adjustment processes, traditional insurance is not well-suited to pandemic-caused business interruption; many argue pandemics are uninsurable. All agree, however, that the best insurance is prevention, highlighting the need to support better decisions.
The SARS pandemic in 2004 woke parts of the world to pandemic risk. Unfortunately, at that time, technology and data were inadequate to carry out the type of modelling possible today. Now with new machine intelligence and data unification, we can model risks at a hyper-local level to drive predictive analytics guiding preventive services to help mitigate business interruption. Further, these tools can shape government response in real time, optimally redirecting resources and services.
COVID-19 is forcing the insurance industry to rethink pandemic risk and develop new solutions to help before, during, and after events. Government, industry, and the insurance sector can work together to minimize business interruption losses and build societal resilience.
Don't miss any update on this topic
Create a free account and access your personalized content collection with our latest publications and analyses.
License and Republishing
World Economic Forum articles may be republished in accordance with the Creative Commons Attribution-NonCommercial-NoDerivatives 4.0 International Public License, and in accordance with our Terms of Use.
The views expressed in this article are those of the author alone and not the World Economic Forum.
Stay up to date:
Insurance
Related topics:
Forum Stories newsletter
Bringing you weekly curated insights and analysis on the global issues that matter.
More on Global RisksSee all
Marie McAuliffe
December 18, 2024