AI fairness is an economic and social imperative. Here's how to address it
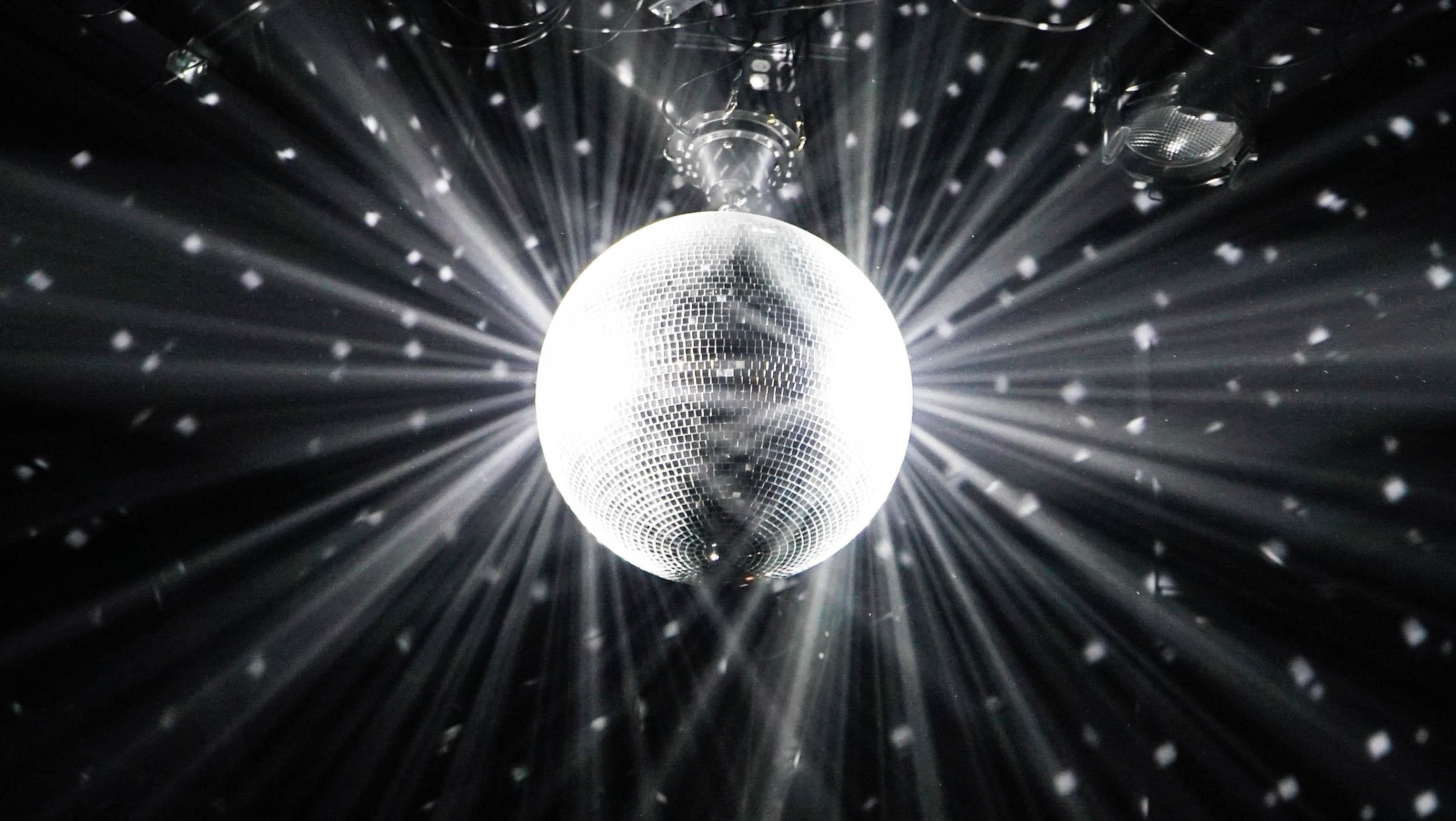
There are so many dimensions to AI fairness, and they are infiltrating data types from text, audio and video, to images and structured data, that your company is unlikely to be tackling them all.
Raja Chatila
Chair, IEEE Global Initiative on Ethics of Autonomous and Intelligent Systems, Institute of Intelligent Systems and Robotics, Sorbonne UniversityStay up to date:
Fourth Industrial Revolution
- Artificial Intelligence (AI) is providing an increasing number of recommendations to human decision makers.
- We must therefore make sure that we can trust not just AI but also its whole ecosystem.
- The notion of fairness itself is fluid and requires a multi-stakeholder consultation.
Humans have many kinds of bias; confirmation, anchoring and gender among them. Such biases may lead people to behave unfairly and, as such, as a society we try to mitigate them.
This is especially important when humans are in the position of taking high-stake decisions that impact others. We do so via a combination of education, conduct guidelines and regulations.
Now that artificial intelligence (AI) is providing an increasing number of recommendations to human decision-makers, it is important to make sure that, as a technology, it is not biased and thus respects the value of fairness.
Indeed, those initiatives that aim to make AI as beneficial as possible (related to AI ethics) include AI fairness as one of the main topics of discussion and concrete work.
It is time to identify and suggest a more comprehensive view of AI fairness; one that covers all dimensions and exploits their interrelation
”Examples of these abound and range from the Ethics Guidelines for Trustworthy AI of the European Commission High Level Expert Group on AI to the focus of the World Economic Forum Global Future Council on AI for Humanity.
While AI fairness has been a major focus for companies, governments, civil society organisations, and multi-stakeholder initiatives for several years now, we have seen a plethora of different approaches over time. Each of these has focused on either one or several aspects of AI fairness.
But it is now time to identify and suggest a more comprehensive view; one that covers all the dimensions of AI fairness and exploits their interrelation, in a bid to build the most effective framework, techniques and policies.
Here's the sticking point, though: since AI systems are built by humans, who collect the training and test data and make the development decisions, they can – consciously or otherwise – be injected with biases. This, in turn, may lead to the deployment of AI systems that reflect and amplify such biases, resulting in decisions or recommendations that are systematically unfair to certain categories of people.
Tools to improve the explainability of AI models enable the identification of the reasons behind the AI decisions, and can therefore be useful to identify bias.
”There are already several technical tools that can help here: they detect and mitigate AI bias over various data types (text, audio, video, images and structured data). Indeed, existing bias in society can be embedded in AI systems, and undesired correlations between some features (such as gender and loan acceptability) can be mitigated by detecting and avoiding them.
Tools to improve the explainability of AI models enable the identification of the reasons behind the AI decisions, and can therefore also be useful in identifying bias in AI data or models. However, technical aspects and solutions to AI bias constitute just one dimension, and possibly the easiest one, of achieving AI fairness.
Beyond the technicalities
Achieving AI fairness is not just a technical problem; it also requires governance structures to identify, implement and adopt appropriate tools to detect and mitigate bias in data collection and processing on the one hand, and frameworks to define the necessary and appropriate oversight for each specific use case on the other.
Let's also not forget that the notion of fairness itself is context dependent, and should be defined according to specific application scenarios. The correct definition can only be identified through a multi-stakeholder consultation in which those who build and deploy AI systems discuss them with users and relevant communities, to identify the relevant notion of fairness.
'Nobody taught me I was biased'
Another dimension of AI fairness relates to education. Since human biases are mostly unconscious, any path to achieving AI fairness necessarily starts from awareness building (educating). AI developers need to become aware of their biases and how they could possibly inject them into AI systems during the development pipeline.
But educating developers is not enough: the whole environment around them must be aware of possible biases, and learn how to detect and mitigate them. A culture of fairness must be built. Within this, managers need to understand how to build diverse developers’ teams, and define incentives for AI bias detection and mitigation.
Executives and decision makers need help in understanding AI bias issues and their possible impact on clients, impacted communities and their own company.
Such education needs to be complemented by appropriate methodologies, which need to not only be adopted, but also enforced and facilitated. To achieve this, companies need to define the most suitable internal governance framework for their business models and deployment domains.
Beyond the systems themselves
Not only should AI systems be fair and exercise caution in amplifying human biases, but they should also make sure they are not a source of inequality among groups or communities.
It follows that diversity and inclusion within societies is another dimension of AI fairness that refers to the impact of the use of AI in a specific societal context. Far from increasing inequality, technology ought to improve accessibility, and also reduce the digital gap along various axes, such as gender, disabilities, geography and ethnicity.
Fairness has a global dimension, too, and must be promoted equally between different regions in the world, but taking into account the specificities of each region.
Another important dimension of AI fairness has to do with how to define the appropriate and shared rules to assure that the particular AI that is deployed and used is fair. AI producers should play their part in defining and implementing internal principles, guidelines, methodologies, and governance frameworks to make sure the AI they produce is fair, robust, explainable, accurate and transparent.
However, there is no reason why other institutions shouldn't help co-create AI frameworks, too; we're talking guidelines, best practices, standards, audits, certifications, regulations and laws. After all, it requires a careful combination of these mechanisms, defined through a multi-stakeholder consultation, to frame the fairness of the AI technology used and its impact on society correctly.
The space of AI fairness is therefore wide and complex. All AI stakeholders should devote time and resources to playing their part in their relevant portion of this space.
The main motivation is clear: making sure technology respects and supports human values, and prevents them from being put in danger. However, there are other motivations for AI builders, too. Indeed, addressing AI fairness is both an economic and social imperative.
Companies that deploy AI or other technologies but cannot be trusted to uphold the values explored here will have challenges in having their products adopted widely.
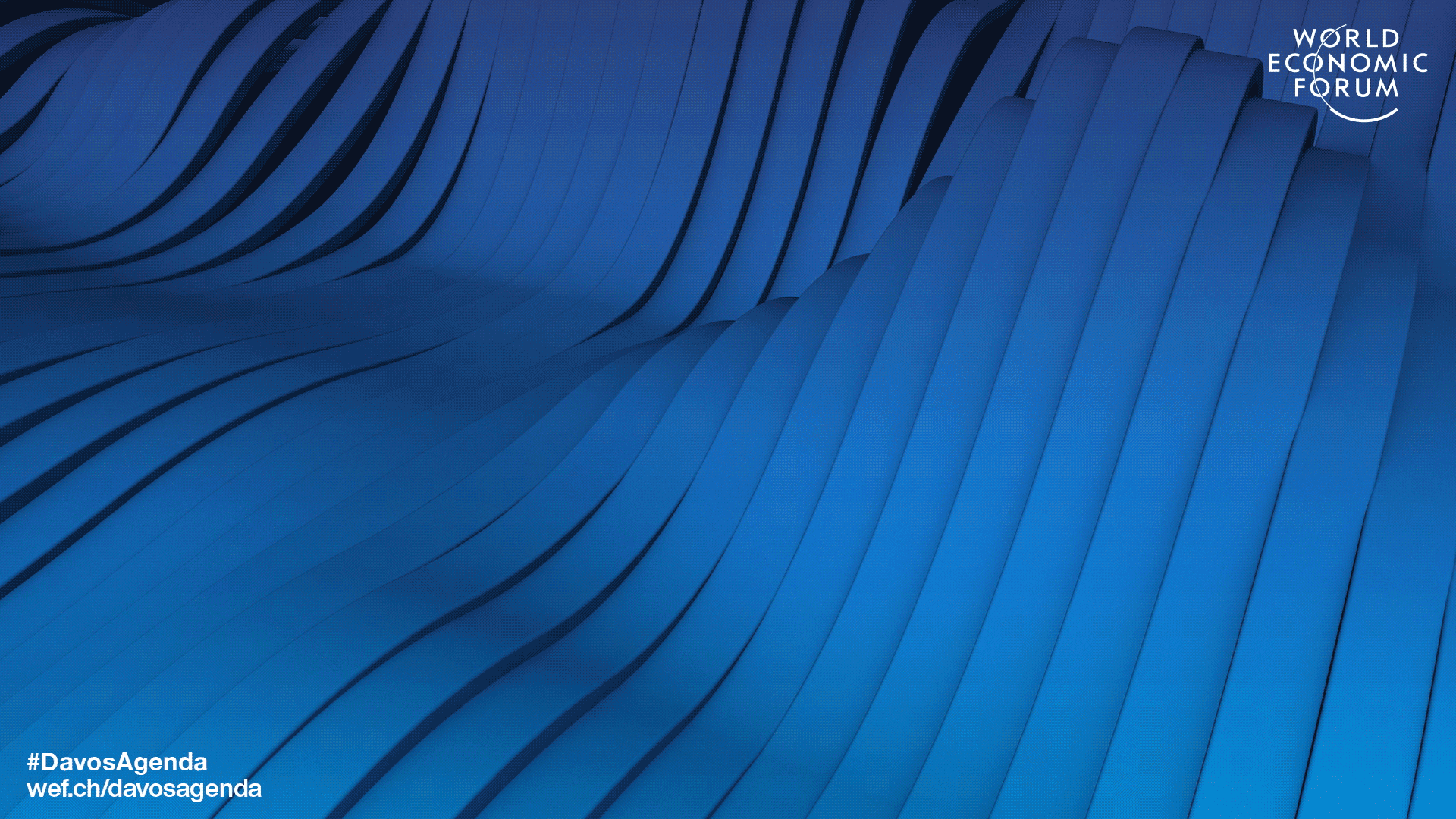
Don't miss any update on this topic
Create a free account and access your personalized content collection with our latest publications and analyses.
License and Republishing
World Economic Forum articles may be republished in accordance with the Creative Commons Attribution-NonCommercial-NoDerivatives 4.0 International Public License, and in accordance with our Terms of Use.
The views expressed in this article are those of the author alone and not the World Economic Forum.
Forum Stories newsletter
Bringing you weekly curated insights and analysis on the global issues that matter.
More on Forum InstitutionalSee all
Madeleine North
January 28, 2025
Klaus Schwab
January 27, 2025
Kaiser Kuo
January 25, 2025
Elizabeth Mills
January 25, 2025