What smartphone data tells us about Tokyo's economy
Millions call Tokyo home.
Image: REUTERS/Issei Kato
Stephen Redding
Harold T. Shapiro Professor in Economics, Economics Department and Woodrow Wilson School, Princeton UniversityStay up to date:
Cities and Urbanization
- A new model uses smartphone data to analyse the spatial variation in the economic activity of the Greater Tokyo metropolitan area.
- It considers both communiting and non-communiting trips to better understand this variation.
- Looking just at community provides a misleading picture of travel patterns, the researchers argue.
Understanding the spatial concentration of economic activity is one of the most central challenges in economics. Traditional theories of cities emphasise production decisions and the costs of workers commuting between their workplace and residence. However, much of the travel that occurs within urban areas is related not to commuting but rather to the consumption of non-traded services, such as trips to restaurants, coffee shops and bars, shopping centres, cultural venues, and other services. A pioneering paper introducing this idea of the ‘consumer city’ is Glaeser et al. (2001).
Two key challenges for research in this area have been a limited ability to measure non-commuting trips and the absence of a widely accepted theoretical model of travel for consumption. In a new paper (Miyauchi et al. 2021), we provide a new theory and evidence on the role of consumption and workplace access in understanding the spatial distribution of economic activity.
We combine smartphone data including high-frequency location information with spatially disaggregated census data to measure commuting and non-commuting trips within the Greater Tokyo metropolitan area. Guided by our empirical findings, we develop a quantitative urban model that incorporates both workplace and consumption access. We use the model to evaluate the role of consumption access in explaining the observed spatial variation in economic activity. Incorporating consumption access is quantitatively relevant for evaluating the observed impact of transport infrastructure improvements.
We first use our smartphone data to provide fine-resolution evidence on travel within the Greater Tokyo metropolitan area. Our data come from a major smartphone mapping application in Japan (Docomo Chizu NAVI), which records the GPS location of each device every five minutes. In July of 2019, the data covered about 545,000 users, with 1.4 billion data points. We measure each location visited by a user using a ‘stay’, which corresponds to no movement within 100 metres for 15 minutes.
Figure 1 shows the ‘stays’ recorded in our data for the example of Meiji Shrine, in the Shibuya municipality of Tokyo, from December 2017 to February 2018. Each red-shaded rectangle corresponds a 25-metre-by-25-metre grid cell. The darker the red shading, the larger the number of stays in that grid cell. These grid cells have been overlaid on a satellite photograph of the neighbourhood. In this photograph, the building towards the top-left of the image surrounded by trees is the main building of the Meiji Shrine.
Figure 1 Example of stays around Meiji Shrine in the Shibuya Municipality, Tokyo
Several features are apparent from this image. First, movement within the city can be observed at an extremely fine level of spatial resolution. Second, there is a sharp discontinuity in the density of stays at the road separating the wooded area around the shrine (left-hand side) from the developed area (right-hand side), suggesting that the stays accurately capture the density of movement. Third, in the middle of this wooded area, the stays are concentrated tightly along the path that runs from the road to the main building of the shrine, again confirming the ability of our data to capture the main pathways of movement through the city.
Using our smartphone data, we designate each anonymised user’s home location as their most frequent location (defined by groups of geographically contiguous stays) and their work location as their second most frequent location. Non-commuting trips to other locations are allocated into different types using spatially-disaggregated census data on employment by sector. We validate our smartphone commuting measures by showing that they are highly correlated with the measures from the official census data. We also demonstrate that they exhibit patterns in line with the findings of other studies using commuting data, including Ahlfeldt et al. (2015) and Heblich et al. (2020).
Once our smartphone data was validated with the census commuting data, we show that focusing solely on these commuting trips provides a misleading picture of travel patterns. First, non-commuting trips are more frequent than commuting trips, as illustrated in Figure 2. Therefore, concentrating solely on commuting trips substantially underestimates the amount of travel within urban areas.
Figure 2 Frequency of stays at work and other locations (excluding home locations)
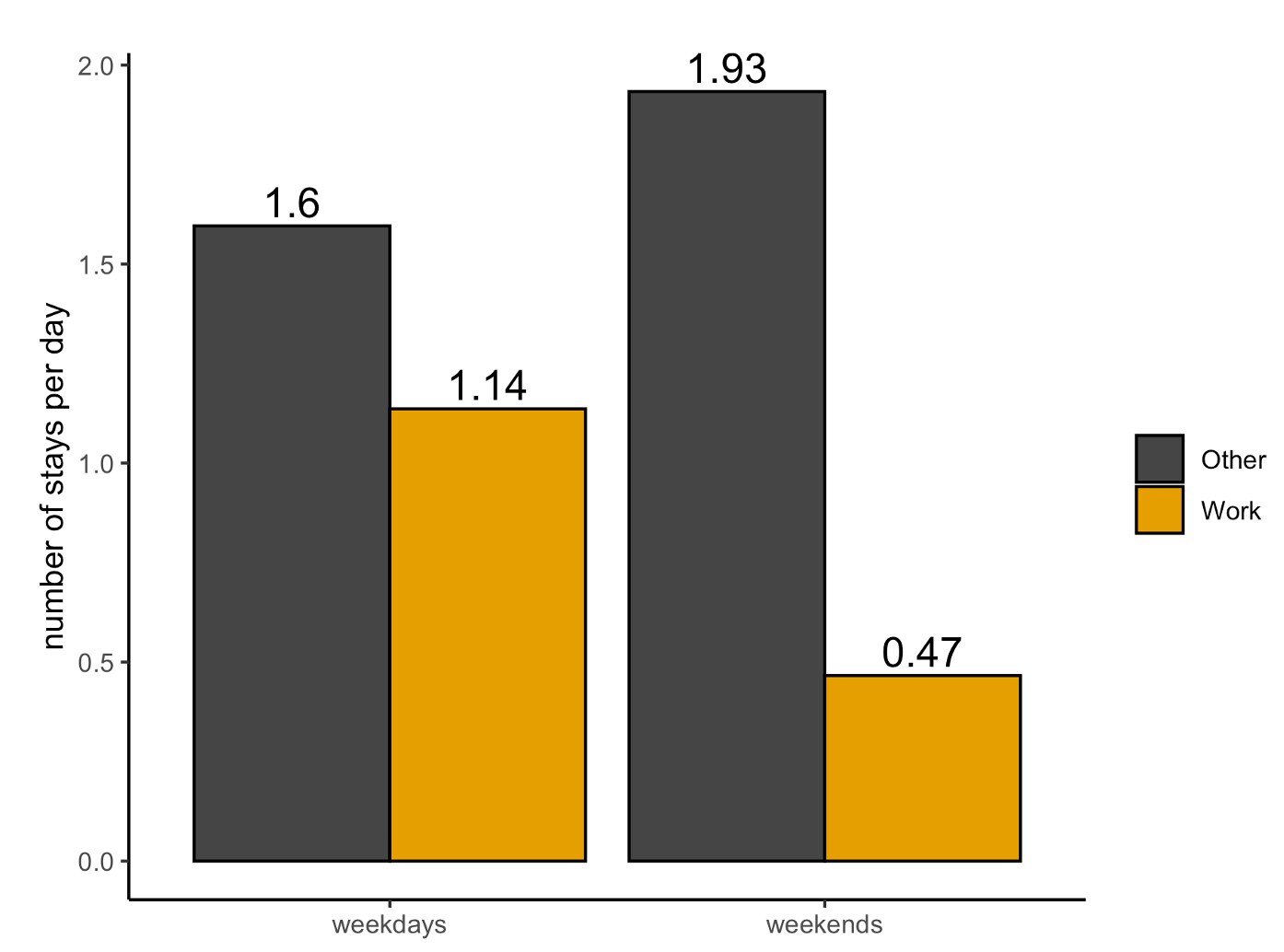
Second, we show that these non-commuting trips are closely related to the availability of non-traded services, which is consistent with our model that views them as travel to consume non-traded services.
Third, we find that non-commuting trips have destinations closer to home than commuting trips, with responses of travel flows to travel times that are larger in absolute value than those for commuting trips. Therefore, the spatial patterns of non-commuting trips are not well approximated by those for commuting trips.
Fourth, we show that trip chains are a relevant feature of the data: non-commuting trips occur along the journey between home and work, highlighting the relevance of jointly modelling commuting and non-commuting trips.
These findings from smartphone data that show the importance of consumption trips are consistent with evidence from new sources of big data, including credit-card transactions (e.g. Allen et al. 2020) and Yelp restaurants data (e.g. Glaeser et al. 2018 and Davis et al. 2019).
To account for the observed patterns in our smartphone data, we develop a quantitative theory of internal city structure that incorporates both commuting and consumption trips. We consider a city that consists of a discrete set of blocks with differing productivity, amenities, supply of floor space and transport connections. Consumer preferences are defined over consumption of a traded good, a number of different types of non-traded services, and residential floor space. The traded good and non-traded services are produced using labour and commercial floor space. We assume that workers’ location decisions are nested.
Workers observe idiosyncratic:
preferences for amenities in each location and choose where to live;productivities in each workplace and sector and choose where to work;qualities for the non-traded services supplied by each location and choose where to consume these non-traded services; andtaste shocks for each route to consume these non-traded services and choose which of these routes to take (e.g. home-work-consume-home versus home-consume-home).
When making each of these choices, workers take into account their expected access to surrounding locations. Population mobility implies that workers must obtain the same expected utility from all populated locations.
The model implies extended gravity equations for commuting and non-commuting trips, which provide good approximations to the observed data. We use these extended gravity equations to estimate a theoretically consistent measure of travel access. Intuitively, we use the observed trips in the data and the structure of the model to reveal the relative attractiveness of locations for employment and consumption. From the model’s population mobility condition, we derive a sufficient statistic for the relative attractiveness of locations, which incorporates both the residential population share and the price of floor space. This sufficient statistic for the relative attractiveness of locations can be decomposed into our measure of travel access and a residual for residential amenities.
Comparing our model that incorporates both consumption and workplace access to a special case that captures only workplace access, we find a substantially larger contribution of travel access once we take into account consumption access (56% compared to 37%), and a correspondingly smaller contribution from the residual of residential amenities (44% compared to 63%).
Taken together, this pattern is consistent with the idea that much economic activity in urban areas is concentrated in the service sector, and that access to surrounding locations to consume these services is an important determinant of workers’ choice of residence and workplace.
The model can be used to undertake a counterfactual for transport infrastructure improvement, such as the construction of a new subway line. In addition to the initial shares of commuting trips, these counterfactuals would now also depend on the initial shares of non-commuting trips.
Frameworks that focus solely on commuting trips generally underestimate the welfare gains from transport-infrastructure improvements because they undercount the number of passenger journeys that benefit from the reduction in travel costs. Furthermore, these frameworks generate different predictions for the impact of new transport infrastructure on the spatial distribution of economic activity because of the different bilateral patterns of commuting and non-commuting trips.
We compare the model’s counterfactual predictions for the opening of a new subway line in the city of Sendai to the observed impact in the data. Incorporating consumption access generates similar estimated effects as in the observed data. Focusing solely on commuting trips underestimates the welfare gains from the transport improvement by around one-half because of the substantial undercounting of non-commuting trips within urban areas.
Taken together, our findings suggest that access to consumption opportunities and access to employment opportunities both play a central role in understanding the spatial concentration of economic activity.
References
Ahlfeldt, G, S Redding, D Sturm and N Wolf (2015), “The economics of density: Evidence from the Berlin Wall”, Econometrica 83(6): 2127–89.
Allen, T, S Fuchs, S Ganapati, A Graziano, R Madera and J Montoriol-Garriga (2020), “Is tourism good for locals? Evidence from Barcelona”, Dartmouth College, mimeograph.
Davis, D R, J I Dingel, J Monras and E Morales (2019), “How segregated is urban consumption?”, Journal of Political Economy 127(4): 1684–738.
Glaeser, E L, J Kolko and A Saiz (2001), “Consumer city”, Journal of Economic Geography 1(1): 27–50.
Glaeser, E L, H Kim and M Luca (2018), “Using traditional and digital data sources together in economic research”, VoxEU.org, 17 January.
Heblich, S, S Redding and D Sturm (2020), “The making of the modern metropolis: Evidence from London”, Quarterly Journal of Economics 135(4): 2059–133.
Miyauchi, Y, K Nakajima and S J Redding (2021), “Consumption access and the spatial concentration of economic activity: Evidence from smartphone data”, CEPR Discussion Paper 15839.
Don't miss any update on this topic
Create a free account and access your personalized content collection with our latest publications and analyses.
License and Republishing
World Economic Forum articles may be republished in accordance with the Creative Commons Attribution-NonCommercial-NoDerivatives 4.0 International Public License, and in accordance with our Terms of Use.
The views expressed in this article are those of the author alone and not the World Economic Forum.
Related topics:
Forum Stories newsletter
Bringing you weekly curated insights and analysis on the global issues that matter.
More on Urban TransformationSee all
Jeff Merritt and Vivian Brady-Phillips
July 25, 2025
Muhammad Hassan Dajana and James Balzer
July 22, 2025
Olivia Nielsen
July 16, 2025
Luis Antonio Ramirez Garcia
July 14, 2025
Sam Markey, Basmah AlBuhairan, Muhammad Al-Humayed and Anu Devi
July 8, 2025