The term AI overpromises. Let's make machine learning work better for humans instead
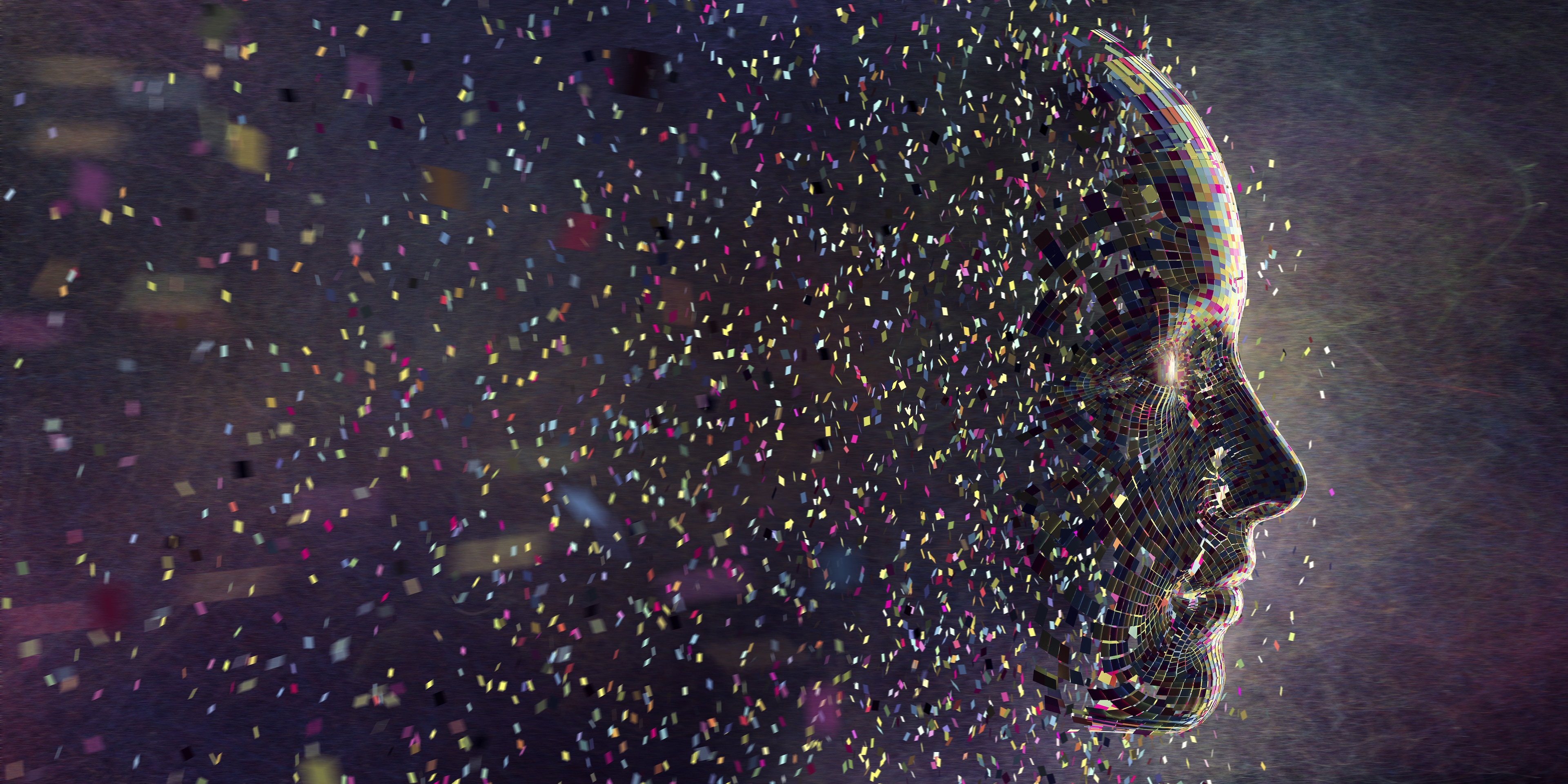
The machine learning sector should strive to integrate human users better.
Image: Getty Images
Stay up to date:
Emerging Technologies
Listen to the article
• Current 'artificial intelligence' operates on a limited 'monkey see monkey do' basis.
• There is little sign such systems can make the leap to higher forms of cognition.
• We should concentrate our efforts into better integrating machine learning with human operators.
One of the popular memes in literature, movies and tech journalism is that man’s creation will rise and destroy it. Lately, this has taken the form of a fear of AI becoming omnipotent, rising up and annihilating mankind.
The economy has jumped on the AI bandwagon; for a certain period, if you did not have “AI” in your investor pitch, you could forget about funding. (Tip: If you are just using a Google service to tag some images, you are not doing AI.)
However, is there actually anything deserving of the term AI? I would like to make the point that there isn’t, and that our current thinking is too focused on working on systems without thinking much about the humans using them, robbing us of the true benefits.
What companies currently employ in the wild are nearly exclusively statistical pattern recognition and replication engines. Basically, all those systems follow the “monkey see, monkey do” pattern: They get fed a certain amount of data and try to mimic some known (or fabricated) output as closely as possible.
When used to provide value, you give them some real-life input and read the predicted output. What if they encounter things never seen before? Well, you better hope that those “new” things are sufficiently similar to previous things, or your “intelligent” system will give quite stupid responses.
But there is not the slightest shred of understanding, reasoning and context in there, just simple re-creation of things seen before. An image recognition system trained to detect sheep in a picture does not have the slightest idea what “sheep” actually means. However, those systems have become so good at recreating the output, that they sometimes look like they know what they are doing.
Isn’t that good enough, you may ask? Well, for some limited cases, it is. But it is not “intelligent”, as it lacks any ability to reason and needs informed users to identify less obvious outliers with possibly harmful downstream effects.
The ladder of thinking has three rungs, pictured in the graph below:
Imitation: You imitate what you have been shown. For this, you do not need any understanding, just correlations. You are able to remember and replicate the past. Lab mice or current AI systems are on this rung.
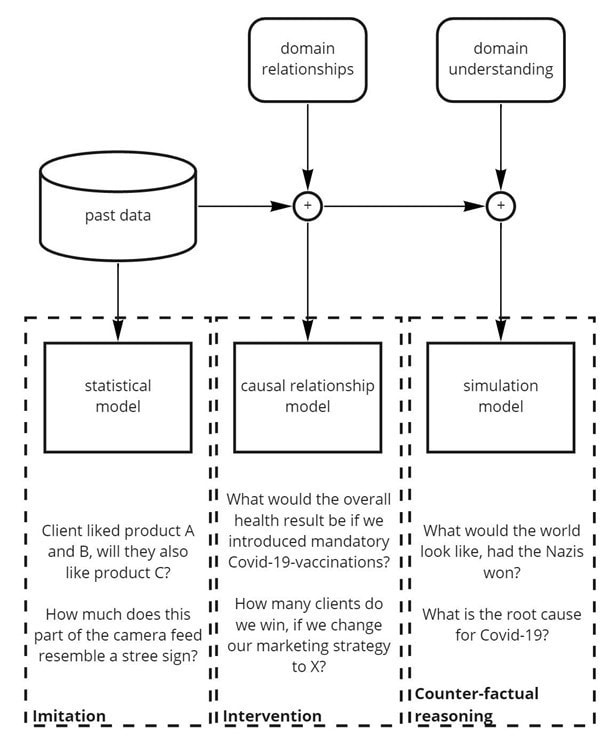
Intervention: You understand causal connections and are able to figure out what would happen if you now would do this, based on what you learned about the world in the past. This requires a mental model of the part of the world you want to influence and the most relevant of its downstream dependencies. You are able to imagine a different future. You meet dogs and small children on that rung, so it is not a bad place to be.
Counterfactual reasoning: The highest rung, where you wonder what would have happened, had you done this or that in the past. This requires a full world model and a way to simulate the world in your head. You are able to imagine multiple pasts and futures. You meet crows, dolphins and adult humans here.
In order to ascend from one rung to the next, you need to develop a completely new set of skills. You can’t just make an imitation system larger and expect it to suddenly be able to reason. Yet this is what we are currently doing with our ever-increasing deep learning models: We think that by giving them more power to imitate, they will at some point magically develop the ability to think. Apart from self-delusional hope and selling nice stories to investors and newspapers, there is little reason to believe that.
And we haven’t even touched the topic of computational complexity and economical and ecological impact of ever-growing models. We might simply not be able to grow our models to the size needed, even if the method worked (which it doesn’t, so far).
Whatever those systems create is the mere semblance of intelligence and in pursuing the goal of generating artificial intelligence by imitation, we are following a cargo cult.
Instead, we should get comfortable with the fact that the current ways will not achieve real AI, and we should stop calling it that. Machine learning (ML) is a perfectly fitting term for a tool with awesome capabilities in the narrow fields where it can be applied. And with any tool, you should not try to make the entire world your nail, but instead find out where to use it and where not.
Machines are strong when it comes to quickly and repeatedly performing a task with minimal uncertainty. They are the ruling class of the first rung.
Humans are strong when it comes to context, understanding and making sense with very little data at hand and high uncertainties. They are the ruling class of the second and third rung.
So what if we focus our efforts away from the current obsession with removing the human element from everything and thought about combining both strengths? There is an enormous potential in giving machine learning systems the optimal, human-centric shape, in finding the right human-machine interface, so that both can shine. The ML system prepares the data, does some automatable tasks and then hands the results to the human, who further handles them according to context.
ML can become something like good staff to a CEO, a workhorse to a farmer or a good user interface to an app user: empowering, saving time, reducing mistakes.
Building a ML system for a given task is rather easy and will become ever easier. But finding a robust, working integration of the data and the pre-processed results of the data with the decision-maker (i.e. human) is a hard task. There is a reason why most ML projects fail at the stage of adoption/integration with the organization seeking to use them.
How is the World Economic Forum ensuring the responsible use of technology?
Solving this is a creative task: It is about domain understanding, product design and communication. Instead of going ever bigger to serve, say, more targetted ads, the true prize is in connecting data and humans in clever ways to make better decisions and be able to solve tougher and more important problems.
Don't miss any update on this topic
Create a free account and access your personalized content collection with our latest publications and analyses.
License and Republishing
World Economic Forum articles may be republished in accordance with the Creative Commons Attribution-NonCommercial-NoDerivatives 4.0 International Public License, and in accordance with our Terms of Use.
The views expressed in this article are those of the author alone and not the World Economic Forum.
Related topics:
Forum Stories newsletter
Bringing you weekly curated insights and analysis on the global issues that matter.
More on Emerging TechnologiesSee all
Cathy Hackl
April 21, 2025
Aimée Dushime
April 18, 2025
Zara Ingilizian and Oliver Wright
April 18, 2025
Dylan Reim and Judith Vega
April 17, 2025
Emmanuel Gatera
April 16, 2025