How does job matching improve productivity in the workplace?
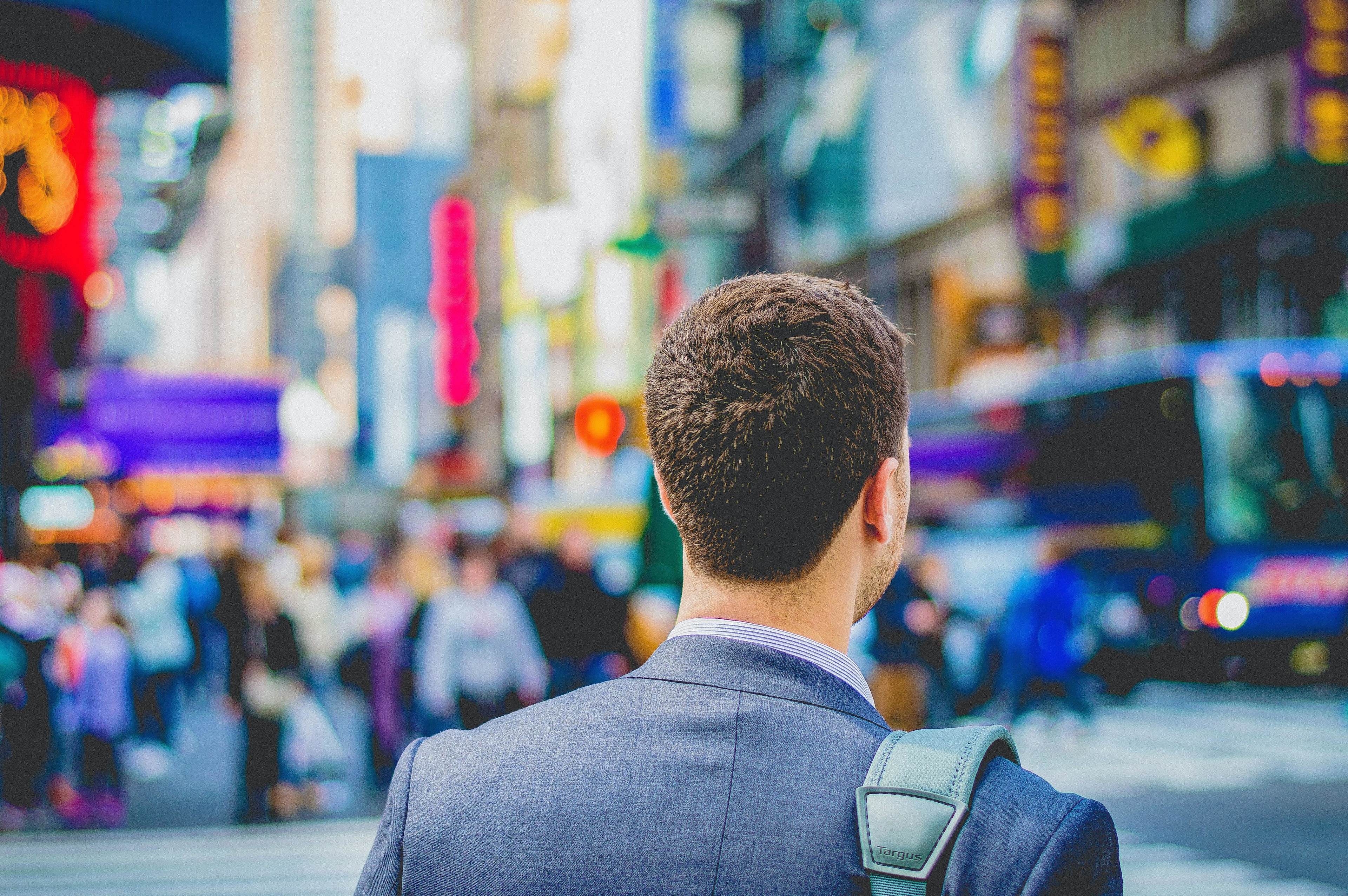
Measuring managers’ ability in matching workers to jobs is itself no easy task. Image: Unsplash/ Saulo Mohana
- Recent research has highlighted the contribution that managerial decisions make to firms’ productivity.
- A company's effectiveness to match employees to their most suitable jobs was an indicator of its overall success, the study found.
- The findings could be particularly valuable at times when the need to cope with technological innovation, pandemics, climate change, or wars requires widespread firm restructuring and workers’ reallocation.
Few questions in economics are as important as what drives the productivity of individual firms and entire countries. Especially if they are persistent over time, differences in productivity end up parting the winners from the losers among firms and among their employees; in the aggregate, they are key determinants of cross-country differences in living standards (Bloom et al. 2010). Traditionally, economists explained differences in productivity by the quality of capital and labour used by firms, and the technology with which they are combined (Syverson 2011).
Lately, economists have realised that the management of human resources is no less important in determining firm productivity. Research on managerial practices (Bloom and Van Reenen 2007, Bloom et al. 2017, 2019, Cette et al. 2020, Patnaik et al. 2021) has highlighted that managerial decisions contribute to firms’ productivity by fine-tuning workers’ incentives, via pay for performance, promotions, and monitoring. But less emphasis has been placed on how managers match workers to jobs. As each worker has specific skills and attitudes to different tasks, and typically hones them during his or her career, allocating each to the best possible job is a non-trivial problem that not all managers may be equally good at solving. Yet, a manager’s skill in solving this matching problem can, in principle, be an important determinant of a firm’s productivity, especially in the face of large shocks that require firm restructuring and reallocation of workers, such as digitalisation, pandemics, and climate change.
Measuring managers’ ability in matching workers to jobs is itself no easy task. The reason why it has been neglected in studies of managerial practices is that these are based on replies to surveys regarding the way managers run their firms’ operations, and it would be very difficult to use self-reported information to evaluate whether they allocate each worker to the best possible use within the firm. In a recent paper (Coraggio et al. 2022), we attack the problem by combining machine-learning (ML) techniques with administrative employer-employee matched data and bring them jointly to bear on the measurement of the quality of job-worker matches within firms. We then validate the resulting measure of job assignment quality (JAQ) by exploring its correlation with workers’ wages over their careers and with firm performance, and by testing whether improving management quality increases the match quality of rank-and-file employees.
A new measure of job assignment quality
The idea behind our measure is simple: to understand how well firms allocate their employees to jobs, we first identify a set of firms which supposedly excel at this task (‘top firms’) and then learn from them how they allocate workers, obtaining an ‘optimal allocation rule’. By learning from the top firms, the rule mimics their behaviour and allows us to predict the most suitable job for each employee.1
Essentially, the rule synthesises the top firms’ managers’ allocation strategies as conveyed by their decisions. But what makes a firm a ‘top’ one? It seems natural to expect that firms that excel in assigning employees to jobs where they are most productive will themselves be more productive than others. Thus, the optimal allocation rule is best inferred from the firms in the top productivity decile.2
We apply this logic to Swedish employer-employee administrative data for 2001–10, proceeding in four steps. First, we estimate via a ML algorithm how workers’ characteristics map into jobs in the most productive firms. Second, we predict worker suitability to each job based on the function estimated via the algorithm. Third, we measure whether a worker’s actual job coincides with the job that she is predicted to be most suited for within the firm (eJAQ). Finally, we build the firm-level JAQ measure by averaging eJAQ across all the employees of each firm.
Job assignment quality correlates with productivity
If our measures contain meaningful information, the worker-level measure should be positively and significantly associated with labour market experience and with workers’ wages – as managers learn about workers’ characteristics, they can be expected to assign them to more suitable jobs (Fredriksson et al., 2018), and workers themselves adapt their skills to their tasks via on-the-job training (Guvenen et al 2020). Moreover, insofar as an improvement in job allocation generates productivity gains, these are likely to be partly appropriated by workers in the form of higher wages.
Both predictions find support in our data. Figure 1 shows the binned scatter plot of eJAQ against labour market experience. The likelihood of being assigned to the job predicted by the ML algorithm increases with experience, almost doubling (from 35% to 63%) over the span of a 50-year working life. The largest gain (about 17 percentage points) occurs in the first five years of a worker’s career – this accords with the intuition that learning is faster for junior workers, and that their reallocation to more suitable jobs is easier than for senior employees (Farber and Gibbons 1996). Moreover, better matches between workers and jobs are associated with higher compensation, suggesting that assigning workers to the right jobs brings about efficiency gains – a worker allocated to her most suitable job earns 0.7%-1.4% more than a mismatched worker with the same characteristics.
Figure 1 Worker-level job allocation quality and work experience
At the firm level, JAQ features a significant, sizeable, and robust positive correlation with measures of firm performance, even when controlling for the firm-level variables generally associated with productivity (industry, capital and labour, ownership) and the workers’ characteristics used to predict JAQ. Figure 2 shows that firm-level productivity correlates positively with JAQ, controlling for year and 2-digit industry dummies. Investigating the correlation of JAQ with various indicators of firm performance, we find that a one-standard-deviation increase in JAQ is associated with a 15.7% increase in sales per employee. This is consistent with Criscuolo et al. (2021), who find that differences in productivity outcomes of frontier and laggard firms depend on the composition, diversity, and competencies of their managers and their workers, and that this ‘human side’ explains about a third of the observed differences in productivity across firms, based on the analysis of employer-employee data set from ten countries.
Figure 2 Firm productivity and job allocation quality
Note: The figure plots firm productivity, measured by the value added per employee, against the firm-level measure of job assignment quality (JAQ), controlling for year and industry fixed effects.
Management matters for matching
These results are consistent with our algorithm capturing a best-practice rule to allocate workers to jobs, whose adoption is correlated with higher firm-level productivity. Why don’t all firms in our sample follow such a best-practice rule? As the hiring of workers, their assignment to jobs, and promotions are typically management decisions, it is natural to inquire whether workers’ assignment to jobs is systematically related to managerial quality in our data.
Indeed, this is the case. Figure 3 show the estimated dynamic treatment effects on rank-and-file workers around managerial turnover events, respectively associated with an increase (panel a) or a decrease (panel b) in the quality of the firm’s management (measured by the management’s own eJAQ). Panel a shows that replacement of incumbent managers with better ones tends to occur in the wake of sharp and statistically significant deterioration in the allocation of rank-and-file workers to jobs (by 10 percentage points on average), and are followed by a significant and persistent improvement of the same magnitude over the subsequent five years, starting at the time of the first replacement. Conversely, panel b indicates that replacement of incumbent managers with worse ones tend to occur in firms that start from a normal level of managerial quality, and are associated with a strong, persistent, and statistically significant deterioration in the allocation of rank-and-file workers (by about 15 percentage points on average). Overall, this evidence suggests that changes in managerial quality are an important driver of changes in workers’ allocation, and therefore – for better or for worse – of organisational change within firms.
Have you read?
Figure 3 Response of rank-and-file workers’ JAQ to management turnover resulting in higher (a) or lower (b) managers’ JAQ
a) Management turnover with increase in managers' JAQ
b) Management turnover with decrease in managers' JAQ
Note: The figure shows the coefficients of event time dummies around managerial turnover events in regressions explaining the JAQ of rank-and-file workers, using the method by Callaway and Sant’Anna (2021).
Conclusions
To sum up, the matching between workers and jobs matters for productivity differentials across firms and responds significantly to changes in the skills and experience of management, when the quality of worker-job matches is measured as proposed by Coraggio et al. (2022).
In fact, this measure can be applied more widely in testing predictions regarding the allocation of human capital in corporate finance, organisation theory, and labour economics. Moreover, it can be constructed for any country and time period where employer-employee administrative data exist and include workers’ job assignments, without requiring either expensive surveys (Bloom and Van Reenen 2007, Bloom et al. 2019) or detailed expert evaluations of the skills required for each job (Lise and Postel-Vinay 2020, Guvenen et al. 2020).
Needless to say, a measure of the quality of job-worker matches is particularly valuable at times when technological innovation such digitalisation and artificial intelligence, and disasters such as pandemics or climate change, force widespread firm restructuring and require the reallocation of existing workers to new jobs or their replacement with workers featuring new skills.
References
Bloom, N, E Brynjolfsson, L Foster, R Jarmin, M Patnaik, I Saporta-Eksten, and J Van Reenen (2017), “Adding a piece to the productivity puzzle: management practices”, VoxEU.org 17 May.
Bloom, N, E Brynjolfsson, L Foster, R Jarmin, M Patnaik, I Saporta-Eksten, and J Van Reenen (2019), “What Drives Differences in Management Practices?”, American Economic Review 109(5): 1648– 1683.
Bloom, N, R Homkes, R Sadun and J Van Reenen (2010), “Why good practices really matter in healthcare”, VoxEU.org 17 December.
Bloom, N and J Van Reenen (2007), “Measuring and Explaining Management Practices Across Firms and Countries”, The Quarterly Journal of Economics 122(4): 1351–1408.
Callaway, B and P H Sant’Anna (2021), “Difference-in-differences with multiple time periods”, Journal of Econometrics 225(2): 200–230.
Cette, G, J Lopez, J Mairesse, and G Nicoletti (2020), “Managerial practices influence the ability of economies to weather large shocks”, VoxEU.org, 2 December.
Coraggio, L, M Pagano, A Scognamiglio and J Tåg (2022), “JAQ of All Trades: Job Mismatch, Firm Productivity and Managerial Quality”, CEPR Discussion Paper No. 17167.
Criscuolo, C, P Gal, T Leidecker and G Nicoletti (2021), “The human side of productivity: Uncovering the role of skills and diversity for firm productivity”, VoxEU.org 23 December.
Farber, H S and R Gibbons (1996), “Learning and Wage Dynamics”, The Quarterly Journal of Economics 111(4): 1007–1047.
Fredriksson, P, L Hensvik, and O N Skans (2018), “Mismatch of Talent: Evidence on Match Quality, Entry Wages, and Job Mobility”, American Economic Review 108(11): 3303–3338.
Guvenen, F, B Kuruscu, S Tanaka, and D Wiczer (2020), “Multidimensional Skill Mismatch”, American Economic Journal: Macroeconomics 12(1): 210–244.
Lise, J and F Postel-Vinay (2020), “Multidimensional Skills, Sorting, and Human Capital Accumulation”, American Economic Review 110(8): 2328–2376.
Patnaik, M, A Lamorgese, A Linarello, and F Schivardi (2021), “Management practices boosted firm performance in COVID-19 by facilitating remote work: evidence from Italy”, VoxEU.org, 1 May.
Syverson, C (2011), “What Determines Productivity?”, Journal of Economic Literature 49(2): 326–365.
Endnotes
1 The rule takes into account both firm and employee characteristics in predicting job allocations.
2 Productivity deciles are computed on productivity fixed effects from a regression of productivity on other determinants including firms’ fixed effects. The analysis is done within firms’ industry and size classes.
Don't miss any update on this topic
Create a free account and access your personalized content collection with our latest publications and analyses.
License and Republishing
World Economic Forum articles may be republished in accordance with the Creative Commons Attribution-NonCommercial-NoDerivatives 4.0 International Public License, and in accordance with our Terms of Use.
The views expressed in this article are those of the author alone and not the World Economic Forum.
Stay up to date:
Society & Future of Work
Related topics:
Forum Stories newsletter
Bringing you weekly curated insights and analysis on the global issues that matter.
More on Jobs and the Future of WorkSee all
Kate Bravery and Mona Mourshed
December 20, 2024