Davos 2024: 5 business leaders on adopting AI and managing associated risks
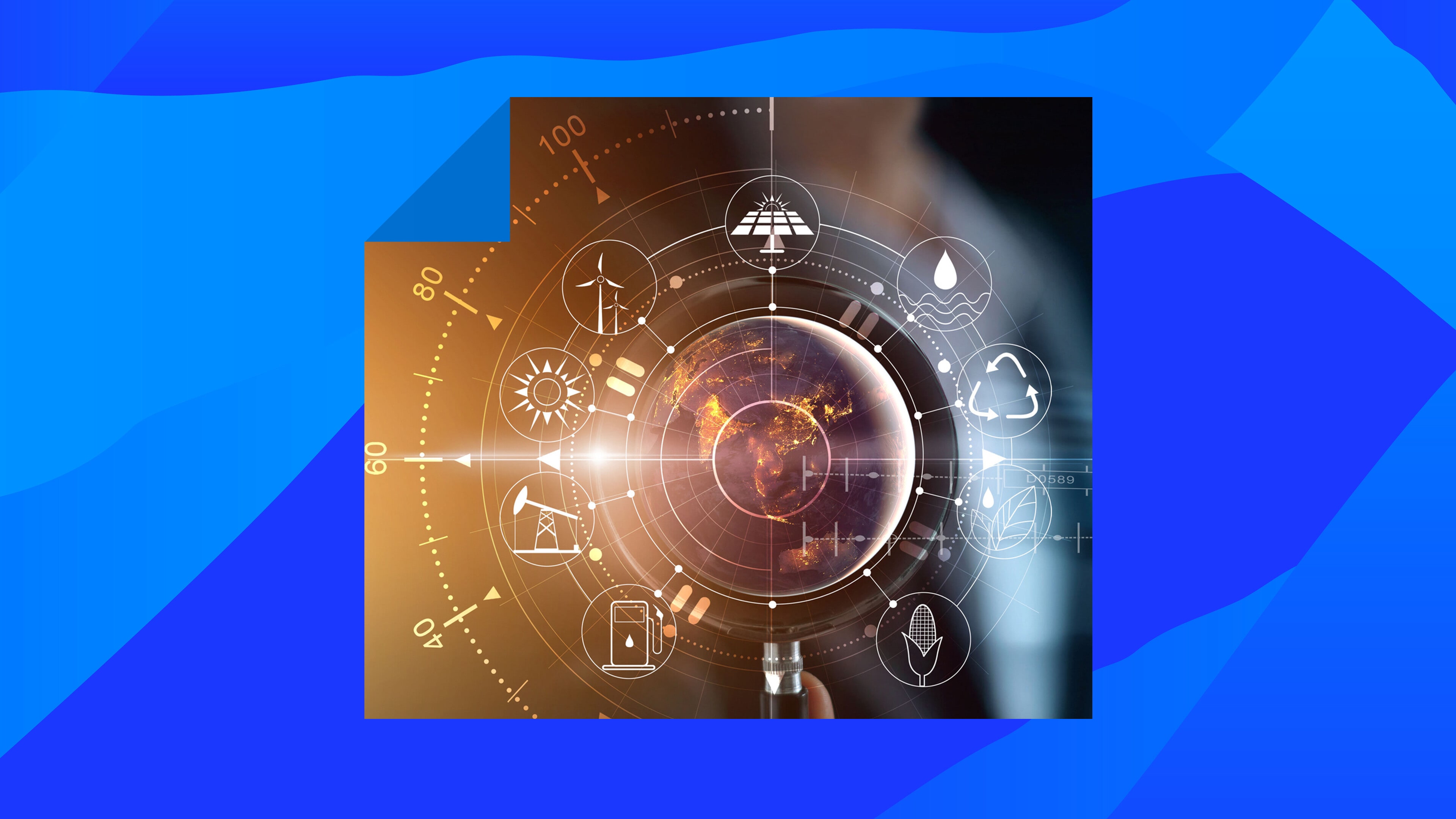
From early adopters to late adopters, who's getting it right on AI? Image: Unsplash/Hitesh Choudhary
- Operationalizing artificial intelligence (AI) isn’t without challenges, but unlike previous technologies, it has the ability to troubleshoot some of its own problems within its design.
- Effective AI implementation has to be with people at the centre of AI governance systems to alleviate the trust and confidence in AI for critical sectors.
- A new playbook of integrated information and operational technology will be needed to utilize AI and automation and take businesses to new bounds.
Artificial intelligence (AI) – despite important consequences on job re-organization and potential replacement of some positions – will lead to the emergence of a range of new roles, outlines the newly released Future of Growth Report 2024.
From an innovation growth point of view, it raises an increasingly important question: Where to find the talent needed in some of these fast-growing roles and avoid a scenario in which talent availability becomes a binding constraint for economic development and growth?
On the other hand, the exponential growth of the global AI market also highlights the need for establishing standards and frameworks that ensure responsible AI practices and procurement, especially for commercial enterprises.
How are businesses navigating these challenges while leveraging its potential? Here, four leaders share insights on how their companies are exploring the field.
Have you read?
'Innovation is burgeoning'
Jeff Schumacher, CEO NAX Group
AI is the next frontier in a long line of disruptive technologies, offering enterprises immense potential to create value and solve complex challenges, such as reducing scope3 emissions. Yet, to truly realize the promise of AI, businesses must not only adopt it but also operationalize it.
This process involves connecting AI models with observable actions, leveraging data subsequently fed back into the system to complete the feedback loop. The critical element lies in automating these steps, enabling rapid, self-learning iterations that propel continuous improvement and innovation.
In pursuing this goal, however, established leaders often grapple with limitations rooted in experience, hampered by technology functions inundated with backlogs and technical debt from critical systems reliant on outdated technologies. These challenges highlight the impracticality of attempting transformation from within – it’s too time-consuming, costly, and risky.
To truly realize the promise of AI, businesses must not only adopt it but also operationalize it.
”In recognizing these hurdles and the escalating need to confront large-scale systemic issues, businesses are increasingly forging strategic partnerships with software and services companies. Such collaborations signify the evolving landscape of industry practices and serve as a catalyst for businesses to create innovative solutions to challenges that previously seemed insurmountable.
'People are key to confidence in AI'
Carmine Di Sibio, EY Global Chairman and CEO
The biggest threat to business adoption of AI is not a lack of desire but confidence. In a recent EY survey, nearly 70% of CEOs revealed that uncertainty around generative AI made it challenging to develop and execute a strategy quickly.
Leaders’ concerns are not without merit. Consider AI chatbots: they produce erroneous outputs or “hallucinate” 3% of the time, with incidents peaking at 27%. Companies also have reservations about data privacy, misinformation and intellectual property. In sectors where generative AI has immense potential to improve lives – such as health care, financial services and logistics – such risks are untenable. Indeed, they are for most businesses.
Every new technology has a hype phase before its total value is realized. However, navigating the in-between can be messy. So, how can organizations harness AI confidently? An important first step is implementing people-led governance mechanisms. For example, the EY AI principles cover crucial considerations such as accountability, security, transparency and sustainability. It also means using human input to “fine-tune” models and interpret outputs. In other words, to unlock AI’s value, we must put people at the centre.
After all, technologies we’ve come to rely on – from the internet to cellphones – were once AI-powered transformation strategies to optimize performance and foster confidence in this game-changing technology.
'Pitching AI enthusiasm just right'
Lisa Heneghan, Chief Digital Officer, KPMG International
AI is unquestionably the “internet” moment of our time. The speed at which it will be debated, embraced and improved will surprise even the most enthusiastic supporters. In KPMG’s CEO Outlook survey, 70% of senior executives told us that generative AI is their top investment priority and that it’ll pay off in the next three to five years. It’s clear that despite economic uncertainty, CEOs are focused and determined to make AI work for them.
For corporate leaders, the danger right now is the “fear of missing out” driving decisions which could damage long-term profits or create new ethical or cyber security challenges. It’s a delicate balancing act between late adopters who may miss golden growth opportunities and early adopters who risk making impulsive moves that could backfire.
Companies should start investing today. Understanding the business case will not be about savings but based on driving experience and skills, which will help identify the opportunities. Focus on getting the foundations in place and building the right tech platforms that are agile enough to adapt to the rapidly evolving AI landscape. It is better to test and learn now than watch and miss the opportunity to lead change – time to market is more important than perfection.
It’s a delicate balancing act between late adopters who may miss golden growth opportunities and early adopters who risk making impulsive moves that could backfire.
”'Making AI work with safety at the fore'
Dr. John Markus Lervik, Chief Strategy & Development Officer and Co-Founder, Cognite
Industrial companies face an AI revolution dilemma. How do they innovate for competitiveness with AI when hallucinations are not an option for critical asset operations in energy and manufacturing?
The ageing industrial workforce, low data literacy, difficulty attracting talent, hazardous working environments, increasingly complex optimization needs and sustainability all call for AI-based solutions, including AI robotics. With disruptive generative AI innovation occurring in every part of society, industrial enterprise boardrooms increasingly push for AI strategy and investment as the highest priority. But how do we make generative AI work for industries where safety is the top priority?
The answer is surprisingly simple. By combining generative AI large language models with industrial knowledge graphs. If the “knowledge graph” is as new to you as large language models, that is because, in the context of digital transformation, they are better known as the data models that power digital twins. From that perspective, large language models need digital twins to make generative AI work for industry.
Digital twins provide a deterministic, real-time, governed and access-controlled digital representation of real-world assets with all its processes, whereas large language models offer a natural language conversational interface for anyone to access the digital twin for insight and analytics. It’s a win-win.
'Companies must act on AI-driven OT automation'
Anant Maheshwari, President and CEO Global High Growth Regions, Honeywell
AI is rapidly creating new opportunities across industries, yet most companies are still trying to define how to use AI to impact profit and loss positively. Accelerating AI across Operational Technology (OT) automation is one opportunity. OT has leveraged control automation for decades, and it is here that AI can become the logical leap forward.
Reaching this goal requires organizations to adopt a new integrated software and hardware playbook for OT as a critical automation enabler. Additionally, the right technology partners are needed to combine a patchwork of IT and OT systems under a common strategy and coherent execution building on embedded control systems. Get this right, and the returns will be significant.
The pace of AI’s evolution is evident and companies must act on AI-driven OT automation now to avoid getting left behind.
”One example of such automation can be seen in a real estate company to reduce energy intensity across an international buildings portfolio. It has achieved higher revenues from tenants who want a data-driven pathway to reduce scope 3 emissions. Another example can be seen from a global energy company that has dramatically reduced downtime and associated costs and increased throughput revenues by deploying AI-enabled controls across its OT assets.
These “early movers” are leveraging AI to impact profit and loss positively and drive higher shareholder returns. However, the pace of AI’s evolution is evident and companies must act on AI-driven OT automation now to avoid getting left behind.
Related topics:
More on Emerging TechnologiesSee all
Michele Mosca and Donna Dodson
December 20, 2024