Large language models and moonshots: Are you thinking too big?
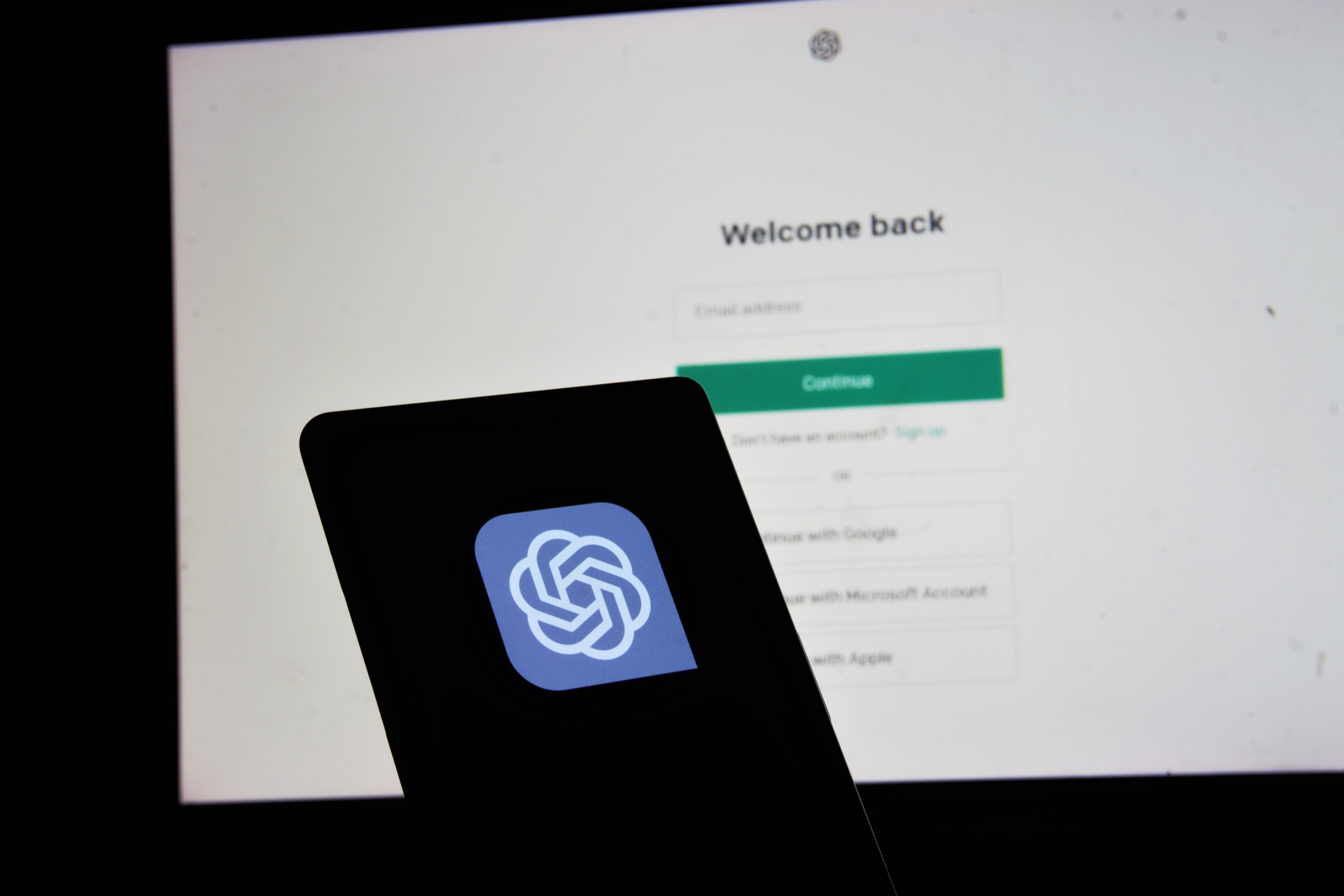
Large language models (LLMs) are having a transformative effect on organizations.
Image: Photo by Mojahid Mottakin on Unsplash
Stay up to date:
Emerging Technologies
- Large language models (LLMs) are having a transformative effect on businesses as they can process and interpret vast amounts of data in unique ways.
- Organizations may be tempted to take a giant leap towards the machine learning tool to leverage LLMs’ capabilities or feel deterred if a systems overhaul is not possible.
- To avoid missing out on the impact of LLMs, small solutions and gradual integration could help organizations utilize the technology without burning cash on moonshots.
Large language models (LLMs) are transforming how we do business today. Trained on vast, diverse datasets, these artificial intelligence (AI) models respond to human inputs (or prompts) with remarkable results. They can write limericks and code, summarize massive corpora of information and even understand protein and molecule structures.
With this kind of technology, our instincts are to think big. And plenty of companies have. But it’s important to understand that not all technological advancements must be groundbreaking to be impactful. The most significant changes often occur in measured, incremental steps.
Here’s how you can leverage LLMs as strategic enhancements to your existing operations, driving real return on investment while fostering a culture that embraces AI and the changes that come with it.
Better healthcare through AI
Consider a healthcare provider with hundreds of thousands of patients and a common problem: employees stretched thin, sometimes allowing important information to slip through the cracks.
In this real case scenario, the missing information was vital patient data. When a patient goes in for a checkup, their doctor will write notes about the next steps. In this case, that information would be inputted into several systems checked by nurses and other hospital employees. Sometimes, a doctor would suggest the patient come back in six months but with no record made. Patients were missing critical follow ups with potentially dramatic consequences for their health.
They solved this problem with an LLM. What the model did was simple: it summarized text in those doctor notes, looking especially for those follow-up visits and flagging each instance. The result was that thousands of patients came in for checkups they might have otherwise missed. This use of LLMs is simple yet powerful – yet it shows the power LLMs can have, especially when they connect to your existing systems, and don't require a complete systems overhaul. And it’s saving lives today.
Strategic framework for deploying LLMs
There are numerous examples of where LLMs can add value but the healthcare case study provides a great rubric to benefit others: using existing data to solve an important problem rather than reinventing the wheel. Drilling down, here’s a framework for using LLMs as tools to solve problems you already have:
- Start with a need, not the technology: Look for operational areas where efficiency can be significantly improved with minimal disruption. Many businesses prioritize areas where communication, data processing or content generation are bottlenecks; however, don’t be afraid to think more broadly.
- Be modest: Begin with manageable projects that can show quick wins. For example, instead of building an entire chatbot for your customer success, consider classifying messages so that an LLM can help triage effectively and fix minor issues before they become big.
- Integrate with existing systems: Ensure your LLM solution complements and enhances your current processes and tools rather than replacing them entirely.
- Focus on return on investment: Machine learning engineers often come from a research background and want to explore. While that’s admirable, it’s not always wise. Prioritize projects that have a clear path to tangible returns.
- Reduce fear and barriers: It’s worth admitting some employees might feel threatened by AI broadly and LLMs specifically. Smaller projects they can interact with and benefit from, especially ones that allow them to leverage their expertise more deeply, can alleviate some of these fears.
What is algorithm bias?
The future is integrated
During the 20th century, when the internet was becoming ubiquitous, you may have run into companies with “internet departments.” While this feels quaint, it is instructive as organizations back then saw a powerful, new technology as something to silo and contain rather than to integrate broadly into their existing infrastructure. For some, the sentiment was that if you weren’t online, then you may as well not exist.
That doesn’t mean that what happened to Blockbuster Video as agile models as Netflix emerged will happen to large companies yet to embrace AI. It’s also doubtful they will adopt a “machine learning department.” The reality is that companies will integrate machine learning in nearly every part of the business. You may not need to be an early adopter of machine learning but you will need to get there eventually.
LLMs are the best way to make that exact transition. There are many ways to start using them, from leveraging commercially available APIs (application programming interfaces), fine-tuning open-source models on your unique data or even building your own LLMs from scratch.
By avoiding costly moonshots, you can build the organizational muscles to implement LLM solutions that will free up employee capacity, make their jobs easier and enhance business efficiency. LLMs can be treated as any other project – assess how much they’ll take to build and the value they provide. However, the key is getting the ball rolling and starting small to avoid not embracing LLMs at all.
Accept our marketing cookies to access this content.
These cookies are currently disabled in your browser.
Don't miss any update on this topic
Create a free account and access your personalized content collection with our latest publications and analyses.
License and Republishing
World Economic Forum articles may be republished in accordance with the Creative Commons Attribution-NonCommercial-NoDerivatives 4.0 International Public License, and in accordance with our Terms of Use.
The views expressed in this article are those of the author alone and not the World Economic Forum.
Forum Stories newsletter
Bringing you weekly curated insights and analysis on the global issues that matter.
More on Emerging TechnologiesSee all
Shahid Ahmed
April 23, 2025
Manfred Elsig and Rodrigo Polanco Lazo
April 23, 2025
Michael Siegel
April 23, 2025
Khalid Alaamer
April 22, 2025
Gustavo Maia
April 22, 2025
Cathy Hackl
April 21, 2025