Causal AI: the revolution uncovering the ‘why’ of decision-making
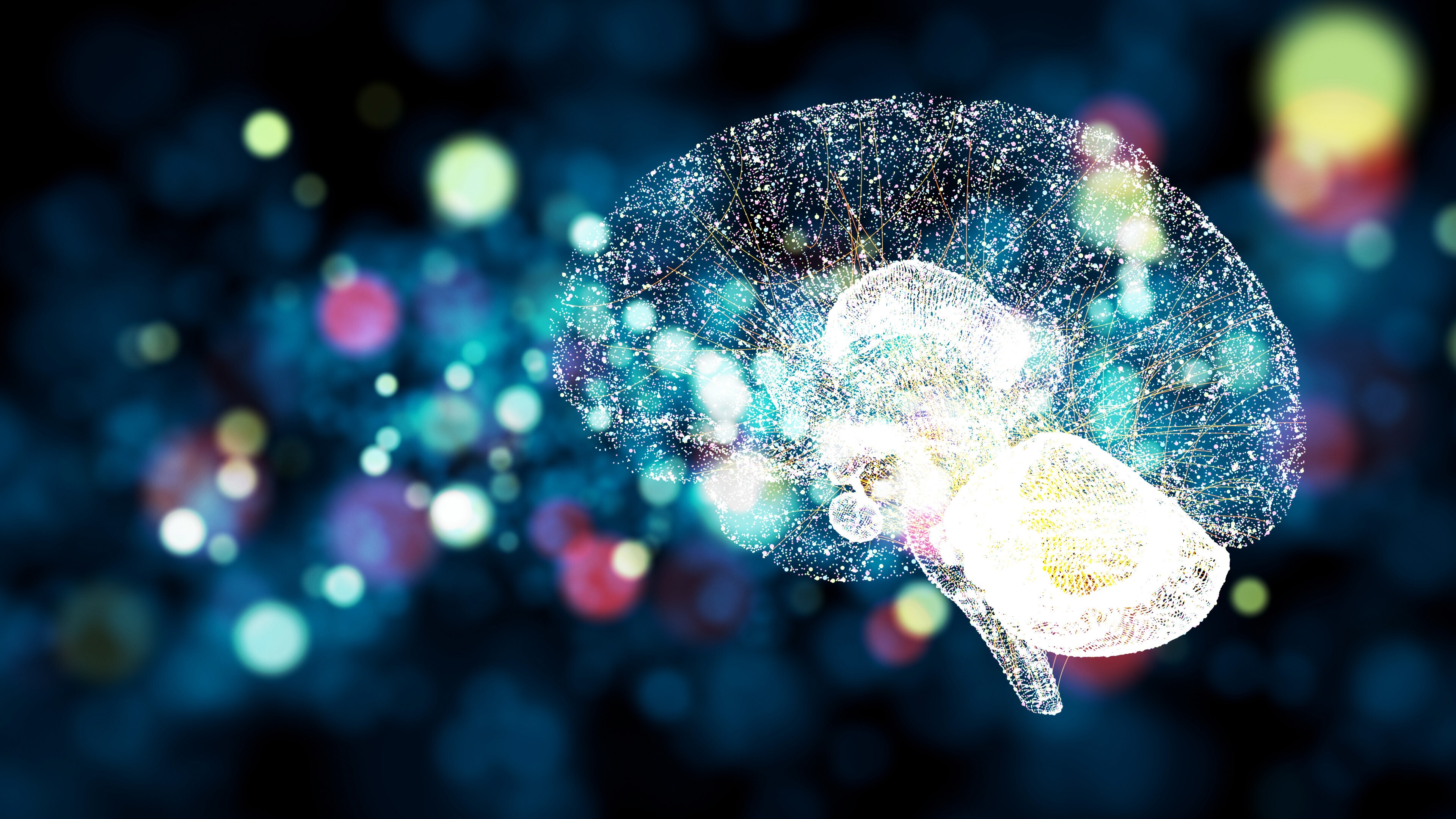
Integrating genAI with causal AI facilitates rapid, intuitive analysis through the former while ensuring decisions are informed by the robust, methodical insights derived from the latter.
Image: Getty Images/iStockphoto
Stay up to date:
Artificial Intelligence
- GenAI is falling short in terms of applicability to decision-making situations.
- Where it relies on recognizing correlations and patterns in events, causal AI is rooted in a deeper understanding of the cause and effect behind them.
- Teaming up genAI and causal AI combines the advantages of fast and slow thinking, facilitating decision-making that is both quick and accurate.
Generative AI (genAI) has shown much promise and generated significant excitement across the technology world over the last 18 months. In particular, Large Language Models (LLMs) can engage in human-like conversations, answer questions, and even create coherent and creative text, leading some to argue that they have effectively passed the Turing test – a long-standing benchmark for evaluating machine intelligence.
This has prompted many to conclude that humanity is progressing towards artificial general intelligence (AGI) and that genAI alone will get us there. However, the lack of success for genAI in current enterprise use cases, particularly those related to decision-making, suggests that LLMs, while impressive, are not the AI silver bullet. In the short term, a more holistic view of AI needs to be taken for enterprise use cases related to decision-making and, in the long term, for the development of AGI.
LLMs operate by transforming natural language into the language of probability and using this to produce outputs. Given a prompt, an LLM will output the word that is statistically the most likely to come next. Knowledge is produced probabilistically. In contrast, most human knowledge is encoded in causal relationships rather than probabilistic ones. Take as an example facts such as “symptoms do not cause disease” and “ash does not cause fire”. Humans use these causal relationships to reason about the world and make decisions. The probabilistic language used by LLMs has no notion of cause and effect.
So, it's little surprise that LLMs often fall short when it comes to reasoning, thinking critically or exhibiting genuine comprehension. For these reasons, LLMs struggle to unlock many enterprise use cases. Hallucinations, bias, lack of transparency and limited interpretability make businesses hesitant to trust LLMs for decision-making.
The challenge of applying LLMs to decision-making use cases highlights the reasons for lack of enterprise adoption in the short term and the gaps when considering whether LLMs alone offer a route to AGI. Larger language models and more training data will not bridge the gap; AI needs additional capabilities, including reasoning on cause and effect.
The human brain and System 2 thinking
A useful way of thinking holistically about the AI landscape is to compare current approaches to the way humans learn, process information and generate outputs. To better understand this, we can draw direct comparisons between contemporary AI techniques and the workings of the human brain.
While the brain remains an enigma in many ways, researchers have proposed frameworks to help explain its complex operations. One such framework, introduced by Nobel laureate Daniel Kahneman in his work Thinking, Fast and Slow, has particularly compelling parallels with the current state of AI.
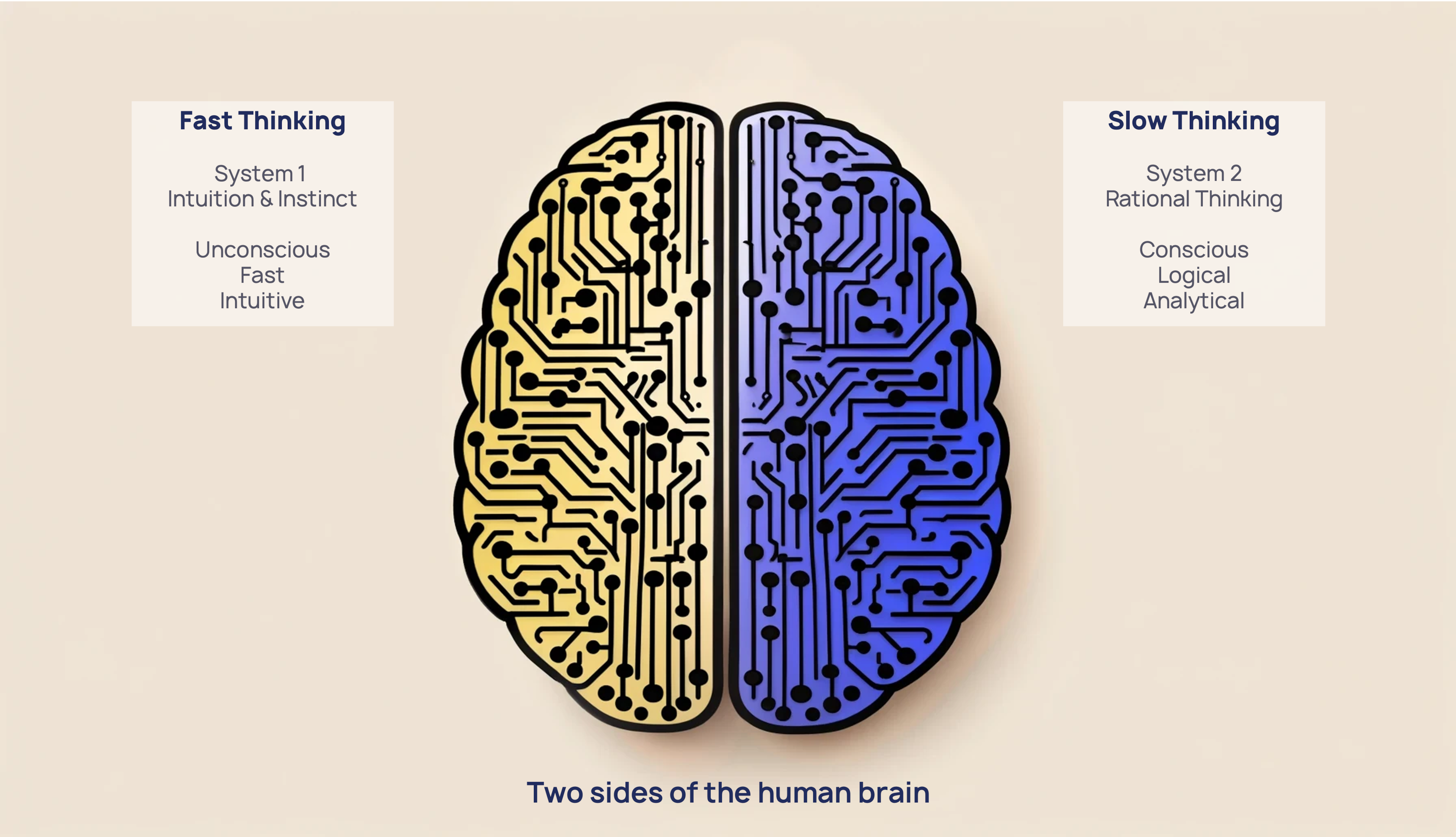
Kahneman's framework posits that the human brain operates in two distinct modes: fast thinking and slow thinking. Fast thinking, or System 1 thinking, is characterized by quick, associative and intuitive processes that occur unconsciously. When presented with a familiar image or situation, our brains instantly respond based on prior experiences and patterns. In contrast, slow thinking (System 2) is a conscious, logical and analytical mode of processing information. It is the thinking we engage in when faced with complex problems or novel situations that require careful reasoning and deliberation.
While genAI, with its ability to process vast amounts of data and generate human-like responses, resembles the fast thinking mode, it lacks the deeper reasoning capabilities that characterize slow thinking. If we assume that true intelligence requires the seamless integration of both modes, it becomes apparent that current AI technologies are insufficient. To bridge this gap, a new approach is required to reproduce the slow, logical and analytical thinking characteristic of human reasoning.
The ‘why’ of causal AI
Causal AI, an emerging field that focuses on creating machines with the ability to understand and reason about cause-and-effect relationships, is one technology that can start to fill the gap. Unlike traditional AI approaches that rely on correlations and patterns, causal AI aims to uncover the underlying causal structures that govern the world.
By learning cause-and-effect information from data and then using this to reason about the world, causal AI operates much more similarly to how humans think when they are thinking slowly. It allows AI to move beyond pattern recognition and delve into understanding the “why” behind the patterns.
One of the key advantages of causal AI is that it enables imagination, or counterfactual reasoning; the ability to ask “what if” questions and explore alternative scenarios. This is a crucial aspect of human decision-making currently lacking in today’s AI. For instance, in healthcare, a causal AI system could analyze patient data and generate counterfactuals to predict how a patient's health would have been different if they had received a different treatment or if certain risk factors were absent.
Causal AI can also help address the issue of bias in AI. By explicitly modelling the causal relationships between variables, causal AI can identify and mitigate spurious correlations and associations that may lead to biased predictions. For example, in the context of credit risk assessment, a causal AI system could distinguish between the true causal factors that influence creditworthiness and the spurious correlations that may arise due to historical biases in the data.
Accept our marketing cookies to access this content.
These cookies are currently disabled in your browser.
The future of enterprise decision-making
The critical challenge for enterprise decision-making is achieving “fast” thinking while ensuring proposed actions are grounded and optimum. Integrating genAI with causal AI facilitates rapid, intuitive analysis through the former while ensuring decisions are informed by the robust, methodical insights derived from the latter. With the application of causal AI, enterprises can address any cause-and-effect challenge. The confluence of causal AI and genAI offers enterprises the dual advantages of speed and accuracy, enabling them to navigate complex decisions confidently and at pace.
Consider the scenario where a retail industry leader seeks to evaluate the effects of a pricing adjustment on sales volume and its consequent impact on the supply chain. Traditional methods involve extensive analysis across various platforms and dashboards. However, with the combined power of genAI and causal AI, the same executive can input this query into an LLM, which then applies advanced pricing and supply chain causal models to deliver insightful analysis. This approach not only elucidates the direct consequences of past actions but also allows for exploring future scenarios, facilitating strategic planning and decision-making.
The collaboration between genAI and causal AI enriches the decision-making process with the capability for natural language queries and responses alongside detailed, comprehensible text-based explanations. This integration is instrumental in investigating and understanding cause-and-effect relationships, thus empowering businesses with a framework for reliable, scalable and explainable decision-making strategies. Enhancing genAI with causal AI heralds a revolution in enterprise decision-making, combining rapid analytical capabilities and deep causal understanding.
How is the World Economic Forum creating guardrails for Artificial Intelligence?
GenAI lacks the necessary grounding for decision-making use cases across the enterprise. By embracing causal reasoning and its potential to enable logical (slow) thinking in AI systems, the number of use cases to which enterprises can apply AI will significantly increase. While AGI will need more than this, causal AI is a step in the right direction.
Accept our marketing cookies to access this content.
These cookies are currently disabled in your browser.
Don't miss any update on this topic
Create a free account and access your personalized content collection with our latest publications and analyses.
License and Republishing
World Economic Forum articles may be republished in accordance with the Creative Commons Attribution-NonCommercial-NoDerivatives 4.0 International Public License, and in accordance with our Terms of Use.
The views expressed in this article are those of the author alone and not the World Economic Forum.
Forum Stories newsletter
Bringing you weekly curated insights and analysis on the global issues that matter.
More on Emerging TechnologiesSee all
Cathy Hackl
April 21, 2025
Aimée Dushime
April 18, 2025
Zara Ingilizian and Oliver Wright
April 18, 2025
Dylan Reim and Judith Vega
April 17, 2025
Emmanuel Gatera
April 16, 2025