How AI is revolutionizing Earth observation
Modern mapping technology is changing the way we view the Earth.
Image: Unsplash.
Stay up to date:
Earth Observation
- AI is transforming Earth observation through 'embeddings' – translating raw data into meaningful insights.
- Embeddings enable AI to recognize patterns in Earth's surface data – more accurately identifying environmental changes.
- This technology has the potential to revolutionize how we interact with, understand and protect our planet.
Maps have captivated us throughout history. Mapping everything would be very confusing, so we are forced to choose certain aspects which reflect our values. Digital maps not only allow you to see, but also search the names of places and limited concepts. But imagine a new kind of map where you can search for virtually anything on Earth. Ecosystems, biodiversity, fires, floods, roads, crops, etc. Thanks to groundbreaking AI techniques, this vision is no longer science fiction but a reality in the making. As these new powerful tools become mainstream, it's imperative we address critical issues around the production of maps: their governance, accessibility, and privacy.
Traditional mapping tools rely on drawing points and lines – modern digital maps on pixels. But both are limited in their ability to connect the details with the bigger picture. AI gives us the superhuman ability to understand both ends of the spectrum, translating raw data into meaningful insights and uncovering the hidden patterns and relationships within Earth’s surface – to see each tree and forest.
The recent rise of AI, particularly the widespread use of Transformer models (the “T” of ChatGPT), has revolutionized many fields, from text generation, to image description, medical diagnosis and weather predictions. At the heart of these models lies the concept of “embeddings”, a powerful technique that helps AI learn by creating numerical summaries, or index cards, that capture both meaning and context. Like a skilled librarian, AI uses embeddings to take note of the smallest nuances and most hidden patterns, instantly pointing to all relevant sources. To illustrate the power of these new embeddings-based tools, since 2019 we can create AI models, like “BERT” , that not only recreate Wikipedia with close to 90% precision, but also are 1% of its size. Embeddings are extremely powerful semantic compressions.
From pixels to predictions
These AI-based embeddings are at the core of these new maps. We are just starting to apply these powerful tools to the vast archives of existing Earth observation from decades ago. We have long had the data to answer questions like how many trees there are in the world, but it always took too much computer effort to go over each one. Cue Earth embeddings, where we have infinite piles of pixels to work with – AI now creates these dense mathematical summaries. A rich tapestry of distilled signals, navigating across space, time and concepts – not just forests and trees, but river flows, floods, droughts, and cities. Earth embeddings are explicitly designed to compress the entire image to extremely short summaries and they are often less than 5% of the size. Of course, these summaries are not perfect, but they have proven remarkably good. In tests using our open model Clay, these embeddings have shown over 90% accuracy in classifying land use, detecting aquaculture, and estimating biomass. It is hard to grasp how profoundly this can change geospatial analysis at scale. Also, how simple it is conceptually and how little used so far.
Earth is the biggest unexplored domain of AI
The general field of Earth observation suffers from a lack of awareness, as pointed out by the recent Amplifying the Global Value of Earth Observation report by the World Economic Forum. It identifies an additional $3.8 trillion opportunity to the global economy and 2Gt of greenhouse gases per year eliminated by 2030. That very same report explicitly references AI as a source of optimism to unlock its value to non-experts, including geo embeddings.
While embeddings hold immense potential for revolutionizing geospatial analysis, their adoption and application remains limited. This is partly because it requires expertise in three distinct areas: AI, geospatial analysis and sustainability. It’s rare to even have expertise in one of these areas. Moreover, like building any other map, it also requires expertise on how to govern the creation and deployment of these tools, as well as addressing privacy and national security concerns, data biases and limitations. Embeddings can vastly reduce barriers of compute, access or technical skills, but they are not ready to be blindly deployed without context or redress and misuse prevention mechanisms.
On the other hand, AI for Earth offers unique benefits for the wider field of AI governance. Other domains, like text, are full of legal uncertainty over copyright and licensing. In AI for Earth we literally have petabytes of completely open data, with open licenses, that include the entire world, monthly, dating back decades. Moreover, AI models of text need to account for a wide range of content quality, propaganda and fiction mixed with historical records. All Earth data represents real observations of real locations. With AI for Earth there is an outsized opportunity to shape expectations of what to do, how to do it and who does it.
How is the World Economic Forum fighting the climate crisis?
So, where do we stand today? There are more than 60 AI for Earth models, such as Microsoft’s SatCLIP, NASA’s Privthi or Clay. They all validate the technical, commercial and public potential of this approach, yet they remain mostly niche, academic and non-operational. It is hard to create them, hard to use them, and too soon to trust them for the critical use cases we have seen, such as automated flood detection, crop predictions, or disaster response. As of October 2024 none of the major AI models, like ChatGPT, Llama, or Claude disclose using any Earth data for training, or are able to understand it.
A new era of geospatial understanding
Embeddings represent a paradigm shift in how we interact with and understand our planet. They help solve the current problem of having extremely large archives of global and historical data, but where no information can easily be found. By moving beyond the limitations of pixels, we can unlock a wealth of easier, faster and better access to information about the natural world, empowering many more stakeholders to make informed decisions about their future.
As climate change and sustainability challenges grow, AI will be crucial in helping us understand and protect our planet. Earth embeddings are not just a technical advancement – they’re a new way of seeing the world, enabling us to make better decisions for the future. It’s time to embrace this technology and unlock its full potential for a healthier, more sustainable Earth.
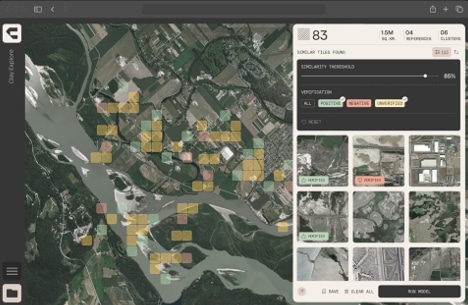
As climate change and sustainability challenges grow, AI will be crucial in helping us understand and protect our planet. Embeddings are not just a technical advancement—they’re a new way of seeing the world, enabling us to make better decisions for the future. It’s time to embrace this technology and unlock its full potential for a healthier, more sustainable Earth.
Accept our marketing cookies to access this content.
These cookies are currently disabled in your browser.
Don't miss any update on this topic
Create a free account and access your personalized content collection with our latest publications and analyses.
License and Republishing
World Economic Forum articles may be republished in accordance with the Creative Commons Attribution-NonCommercial-NoDerivatives 4.0 International Public License, and in accordance with our Terms of Use.
The views expressed in this article are those of the author alone and not the World Economic Forum.
Related topics:
Forum Stories newsletter
Bringing you weekly curated insights and analysis on the global issues that matter.
More on Fourth Industrial RevolutionSee all
Kelly Ommundsen
July 4, 2025
Ibrahim Odeh and Oliver Tsai
July 2, 2025
Hannan Happi
July 1, 2025
Benjamin Hertz-Shargel
July 1, 2025