5 ways generative AI could transform clinical trials
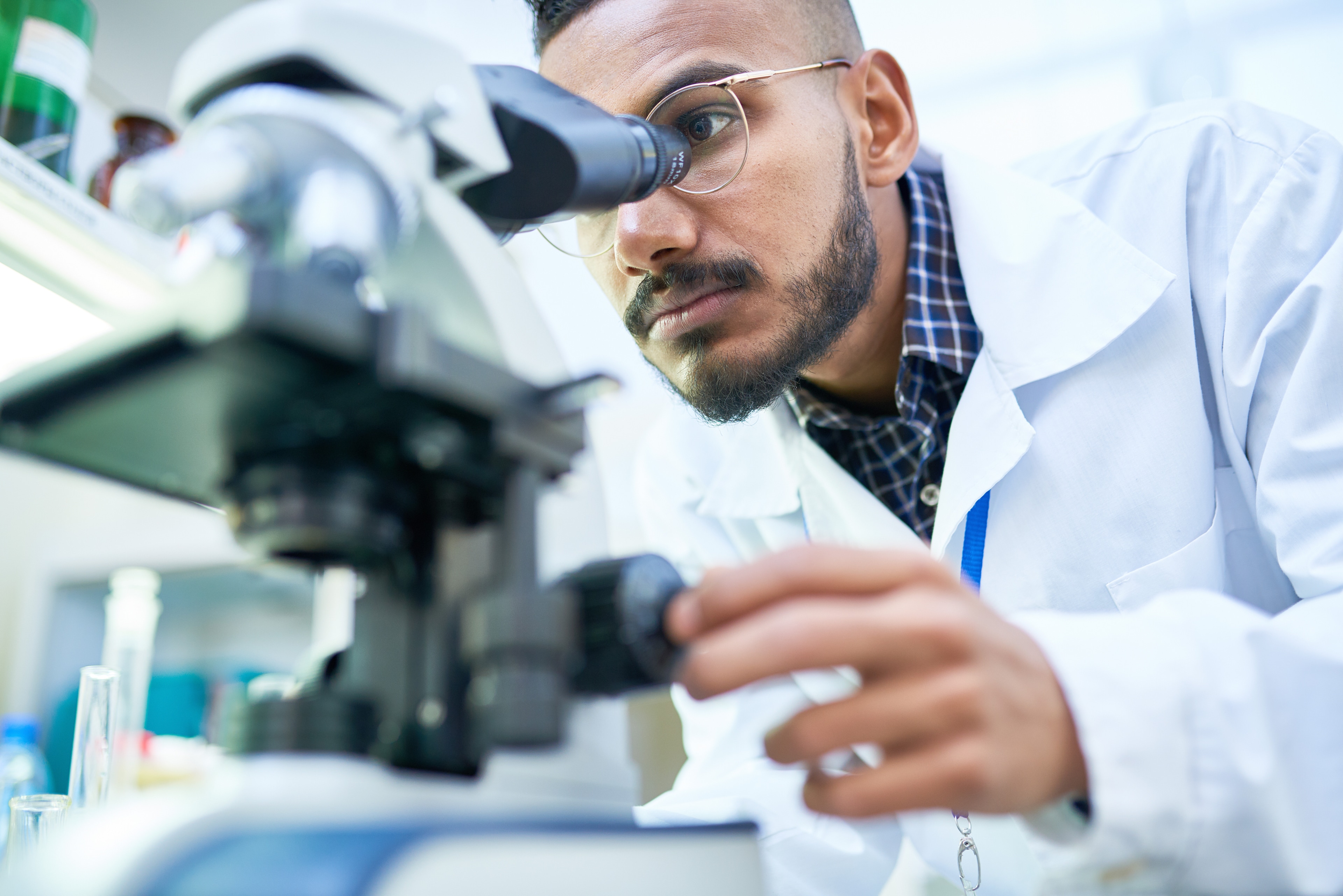
Generative artificial intelligence (genAI) could transform clinical trials and medical research. Image: Getty Images/SeventyFour
- Ushering a new drug from lab to market is expensive and time-consuming – factors that are only increasing over time.
- Using more generative artificial intelligence (genAI) in clinical development processes could help to reduce costs, save time and create more representative clinical trial data.
- A recent report outlines five ways in which genAI could transform clinical trials around the world, as well as how to overcome the main barriers to make this a reality.
The journey from drug discovery to patient access is complex, costly and time-consuming. While generative AI (genAI) is transforming preclinical drug discovery, similar disruptions are needed in clinical development, which is known for inefficiencies.
A recent report, Intelligent Clinical Trials: Using Generative AI to Fast-Track Therapeutic Innovations, by the World Economic Forum and consultancy firm ZS explores how genAI can overcome these challenges in clinical trials. GenAI has the potential to revolutionize clinical development and certain key steps will help to unlock its full impact.
The long road to clinical trial innovation
Bringing a drug from the lab to market is an arduous process, taking between eight and 12 years and often costing upwards of $2.5 billion – figures that are only increasing. There has been a 3-fold increase in cost over the past two decades, while the duration of the third clinical trial phase has extended from approximately 2.25 years in 2010 to 3.25 years in 2021. Even with increasing financial and time investments, clinical trials – which account for nearly 40% of US pharmaceutical company research budgets – continue to be plagued by a 90% failure rate. Trials also often exclude underserved populations, perpetuating inequities in healthcare delivery.
These inefficiencies are not just financial; they also delay access to life-saving treatments. While major initiatives such as decentralized clinical trials and digital endpoints attempt to address some aspects of clinical development, the potential for applying genAI to improve clinical development remains vast.
GenAI: The catalyst for change
Clinical development consists of a series of related, overlapping processes – all of which contribute in varying degrees to a trial’s success and all of which (again, to varying degrees) are ripe for transformation through genAI. Based on interviews with more than a dozen clinical development experts, we’ve identified the five processes with the biggest potential for transformation through genAI.
1. Clinical trial design
Clinical trial design is often called the Holy Grail of clinical development transformation due to its complexity and the enormous benefits of improving it. Current methods are manual, error-prone and time-intensive, causing costly delays. By leveraging unstructured data, real-world evidence and regulatory guidance, companies could streamline trial design. This would reduce timelines and could save hundreds of millions of dollars.
2. Trial feasibility and site selection
Patient recruitment accounts for as much as 40% of clinical trial costs, and yet trial site selection is based on limited data and outdated or ad hoc processes. With genAI, trial sponsors could analyse operational data, disease prevalence and real-time real-world evidence to optimize site selection, predict recruitment potential and ensure sites have the necessary resources and capabilities to conduct studies successfully.
3. Patient recruitment and retention
Slow recruitment methods and narrow inclusion criteria lead to challenges in patient enrolment for clinical trials. This is exacerbated by high workload and challenges faced by clinical trial sites, reducing performance and patient satisfaction. More data sources and predictive AI could be used to identify a broader, more diverse participant pool, while also applying models to predict patient dropout risks and create tailored interventions to keep participants engaged.
4. Data analysis
The growing sophistication of trial data necessitates more efficient analytical techniques. GenAI can automate routine tasks like data cleaning and statistical programming, reducing human error and speeding up decision-making. At the same time, it could also unlock insights across the development lifecycle by significantly reducing the time between data collection and informed decision.
5. Regulatory submission and review
Regulatory submission is a largely siloed and manual affair, leading to inefficiency, errors and duplication of effort. In the future, genAI could automatically convert data into the required formats, flag omissions, cross-reference as needed and ensure regulatory compliance. This would reduce the need for requests for more information from regulators, which bring extra costs for clinical trial providers.
Barriers to genAI adoption
Companies are already investing heavily in AI and genAI to accelerate the delivery of new medicines, but significant challenges remain. For example, a lack of standardized data sharing, inconsistent data quality and absence of incentives to share data can throttle AI’s transformational potential. Differing regulations across geographies also create compliance complexities that could hinder genAI transformation of clinical trials.
Our research also indicated a lack of skilled personnel with expertise in both AI and clinical development. Further, a focus on short-term results and general risk aversion mean clinical development teams often default to the status quo at the expense of taking chances with unproven methods such as genAI. There is also an external trust deficit when it comes to genAI – from regulators to patients and beyond, stakeholders need assurance that AI outputs are safe, unbiased and ethical.
Accelerating the use of genAI in clinical trials
Unlocking genAI's full potential could save billions in wasted costs, while speeding up the delivery of life-saving treatments. Life sciences companies, policymakers, regulators, tech firms and NGOs must all play a role in overcoming these obstacles.
Policymakers and industry leaders must define and deploy clear standards for data collection, sharing and quality. Moreover, the industry must align on which data are necessary to inform the decision process when designing and executing the clinical studies. Governments must also invest in efforts to build infrastructure enabling the secure aggregation and sharing of clinical data.
Public-private partnerships will help to align stakeholder interests, reduce fragmentation and foster the culture of innovation across the healthcare ecosystem. Training programmes and certifications could equip clinical trial teams with the necessary expertise to embed genAI in clinical development without risking patient safety. At the same time, regulatory frameworks must balance the need for AI transparency with data privacy concerns to foster transparency.
Integrating generative AI into clinical trials is not just a business innovation, it's a necessity as healthcare costs continues to rise and global health stagnates. This means optimizing clinical development with genAI is both a moral and a financial imperative. By reimagining trials with AI and collaborating to create a supportive ecosystem, we can drive a new era of therapeutic innovation that benefits patients, healthcare providers and society.
Don't miss any update on this topic
Create a free account and access your personalized content collection with our latest publications and analyses.
License and Republishing
World Economic Forum articles may be republished in accordance with the Creative Commons Attribution-NonCommercial-NoDerivatives 4.0 International Public License, and in accordance with our Terms of Use.
The views expressed in this article are those of the author alone and not the World Economic Forum.
Stay up to date:
Artificial Intelligence
Related topics:
Forum Stories newsletter
Bringing you weekly curated insights and analysis on the global issues that matter.
More on Health and Healthcare SystemsSee all
Shobana Kamineni and Shyam Bishen
January 15, 2025