AI know-how is the final piece of the adoption puzzle. Here's why
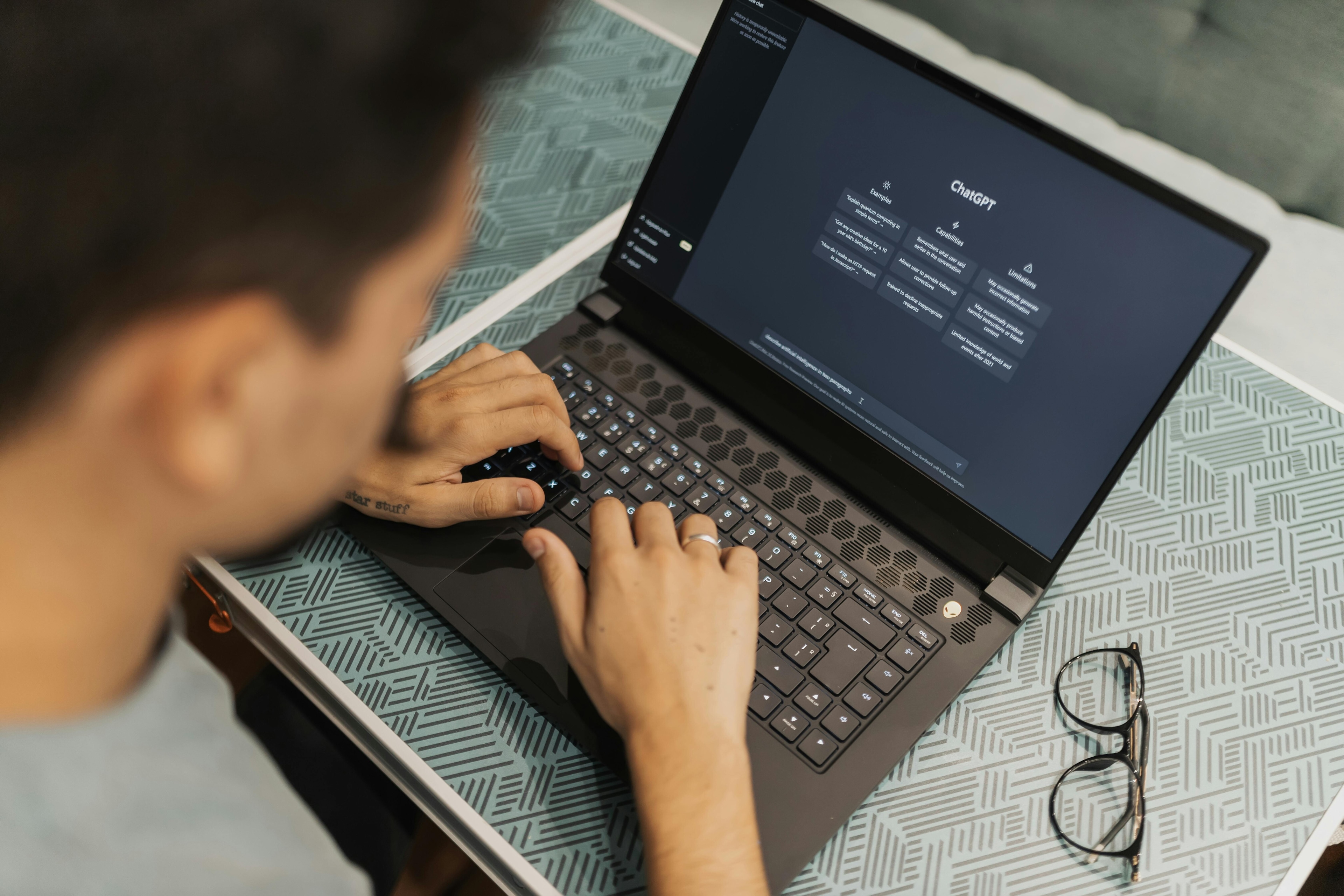
Capturing and sharing AI know-how is the logical next step in making AI's promise real.
Image: Pexels.
Stay up to date:
Artificial Intelligence
- We're entering the era of the "know-how economy" where practical AI know-how is shared, tokenized, and traded as a valuable asset.
- This application of AI knowledge is the missing link in driving widespread adoption and economic impact.
- Ultimately this democratizes and incentivizes knowledge sharing beyond technical experts.
AI investments are surging globally, but returns aren't matching the hype. The practical know-how to turn AI tools into real returns is broadly lacking. Having this knowledge would be like having the cheat codes to a video game, enabling you to finish the mission faster than your opponent.
The people with that know-how have a unique opportunity to make money without trading their time for it; the “knowledge is power era”, where we trade our time for money is coming to an end. There is a shift towards "know-how is money" – where knowing how to create value, by utilizing available tools, has value in itself. For example, a type of intellectual property that others can licence. In the AI age, know-how is what converts hype into profit.
From theoretical to tangible gains
There are more than 50,000 AI solutions currently available. The speed with which solutions are built significantly outpaces the speed with which businesses and employees are learning how to use those tools effectively. Imagine a game with over 50,000 secret passages that unlock new levels, but few know how to unlock them. That's where the opportunity lies.
Companies are investing millions in AI capabilities but struggling to translate that into tangible returns. The practical applications like "here's exactly how we saved $500,000 using ChatGPT to optimize our supply chain," aren't being shared, documented, or leveraged as assets.
It's not some coding wizardry, it's about knowing which prompts get results – like how to ask AI to help you with a sales proposal – which workflows save hours, and which applications boost revenue. This know-how isn't just valuable; it's the basic currency for entry into tomorrow's economy.
Where AI know-how becomes currency
While many struggle to turn AI investments into returns, resourceful businesses with AI know-how are starting to race ahead. For example:
- Savion Ray: a European campaign agency, decreased market research time by 96% and cut video editing from 8 hours to under 1 hour, growing their client base from 15 to 25 without a single new hire. That is a 67% capacity boost.
- Waku: a five person consumer goods startup in New York, turned AI into their sixth teammate, automating CFO tasks, research, emails, and slides.
- Yum Bakery: based in Canada, utilizes Claude to forecast demand and optimize inventory tracking, saving 50 hours per cycle, reducing waste, and securing better vendor deals. They are now developing a sales metric tracker to enhance staff performance, focused on increasing sales and improving customer experience.
- TRN: a global recruitment network based in the UK, turned data into a superpower by using AI to analyze member activity, conversations, and sentiment data, boosting email open rates by aligning content with real-time user needs. This data-driven approach is enabling targeted content creation and boosting event attendance.
These early wins hint at broader potential across industries as tools and know-how evolve. And these success stories don’t just benefit the users – they hand AI solution builders a playbook to turn promises into profits.
The value of AI know-how for AI solution builders
For AI solution builders, these examples are a goldmine. Instead of pitching abstract capabilities, they can showcase a growing library of real-world use cases with verified ROI. Like Savion Ray's growing profits. Or Yum Bakery's cost cuts. Or TRN's increased client satisfaction.
These aren't just testimonials; they're replicable blueprints that other businesses can adapt or license, flipping the traditional adoption curve. Rather than technical support experts slowly trickling applications down to mainstream users, shared know-how makes successful implementations immediately visible and actionable, shrinking the gap between cutting-edge and common practice. This accelerates adoption, turning AI tools from niche experiments into market standards at unprecedented speed.
Shared AI know-how is a way to democratize AI
This approach does more than accelerate adoption; it levels the playing field by turning know-how into a resource that can be shared, adapted, and even traded, like blueprints in a digital marketplace. A retail manager with no coding skills but a knack for inventory management can contribute a game-changing AI workflow, valued for its impact, not its complexity.
LinkedIn's 2025 report highlights that 39% of leaders now prioritize soft skills like adaptability alongside AI literacy. In this emerging landscape, business acumen and domain expertise become as critical as technical skills, ensuring AI's benefits reach beyond tech gatekeepers to every corner of the workforce.
This democratization is key to unlocking AI's economic promise. The $13 trillion of global GDP by 2030, forecasted by McKinsey, can start materializing, but only if millions of practical applications are implemented across industries.
The guardrails
Of course, this requires infrastructure: quality verification systems, impact measurement protocols, and privacy safeguards. These aren’t trivial hurdles and will require iterations to ensure know-how can be traded safely at scale.
The core concept, transforming AI know-how from an individual asset to a tradable resource, could fundamentally reshape how we drive adoption and extract value from this transformative technology. Even without these systems fully in place, there is space for you to step up and contribute to the AI know-how.
Your move in the new game
We're at a tipping point. Goldman Sachs estimates global AI investment could approach $200 billion this year. The technical pieces are falling into place but the missing link is the knowledge layer, the bridge between cutting-edge capability and widespread economic impact.
Capturing and sharing AI know-how is the logical next step in making AI's promise real. By creating a system where valuable AI workflows and applications are documented, shared and traded, we can accelerate adoption while creating entirely new value streams in the digital economy.
Your AI cheat codes aren't just personal advantages. They're assets that could reshape the global economic game. So, play around with the more established solutions like ChatGPT, Claude or Grok, find things that work, and share them. You're not just in the game; you're rewriting the rules.
Accept our marketing cookies to access this content.
These cookies are currently disabled in your browser.
Don't miss any update on this topic
Create a free account and access your personalized content collection with our latest publications and analyses.
License and Republishing
World Economic Forum articles may be republished in accordance with the Creative Commons Attribution-NonCommercial-NoDerivatives 4.0 International Public License, and in accordance with our Terms of Use.
The views expressed in this article are those of the author alone and not the World Economic Forum.
Related topics:
Forum Stories newsletter
Bringing you weekly curated insights and analysis on the global issues that matter.
More on Jobs and the Future of WorkSee all
Eli Joseph
March 28, 2025
Utkarsh Amitabh and Ali Ansari
March 28, 2025
Gareth Francis
March 28, 2025
Matthew Oliver
March 24, 2025
Piyachart (Arm) Isarabhakdee
March 20, 2025
Wouter Durville
March 14, 2025